FIFA: Making Fairness More Generalizable in Classifiers Trained on Imbalanced Data
ICLR 2023(2022)
摘要
Algorithmic fairness plays an important role in machine learning and imposing fairness constraints during learning is a common approach. However, many datasets are imbalanced in certain label classes (e.g. "healthy") and sensitive subgroups (e.g. "older patients"). Empirically, this imbalance leads to a lack of generalizability not only of classification, but also of fairness properties, especially in over-parameterized models. For example, fairness-aware training may ensure equalized odds (EO) on the training data, but EO is far from being satisfied on new users. In this paper, we propose a theoretically-principled, yet Flexible approach that is Imbalance-Fairness-Aware (FIFA). Specifically, FIFA encourages both classification and fairness generalization and can be flexibly combined with many existing fair learning methods with logits-based losses. While our main focus is on EO, FIFA can be directly applied to achieve equalized opportunity (EqOpt); and under certain conditions, it can also be applied to other fairness notions. We demonstrate the power of FIFA by combining it with a popular fair classification algorithm, and the resulting algorithm achieves significantly better fairness generalization on several real-world datasets.
更多查看译文
关键词
Fairness,Generalization
AI 理解论文
溯源树
样例
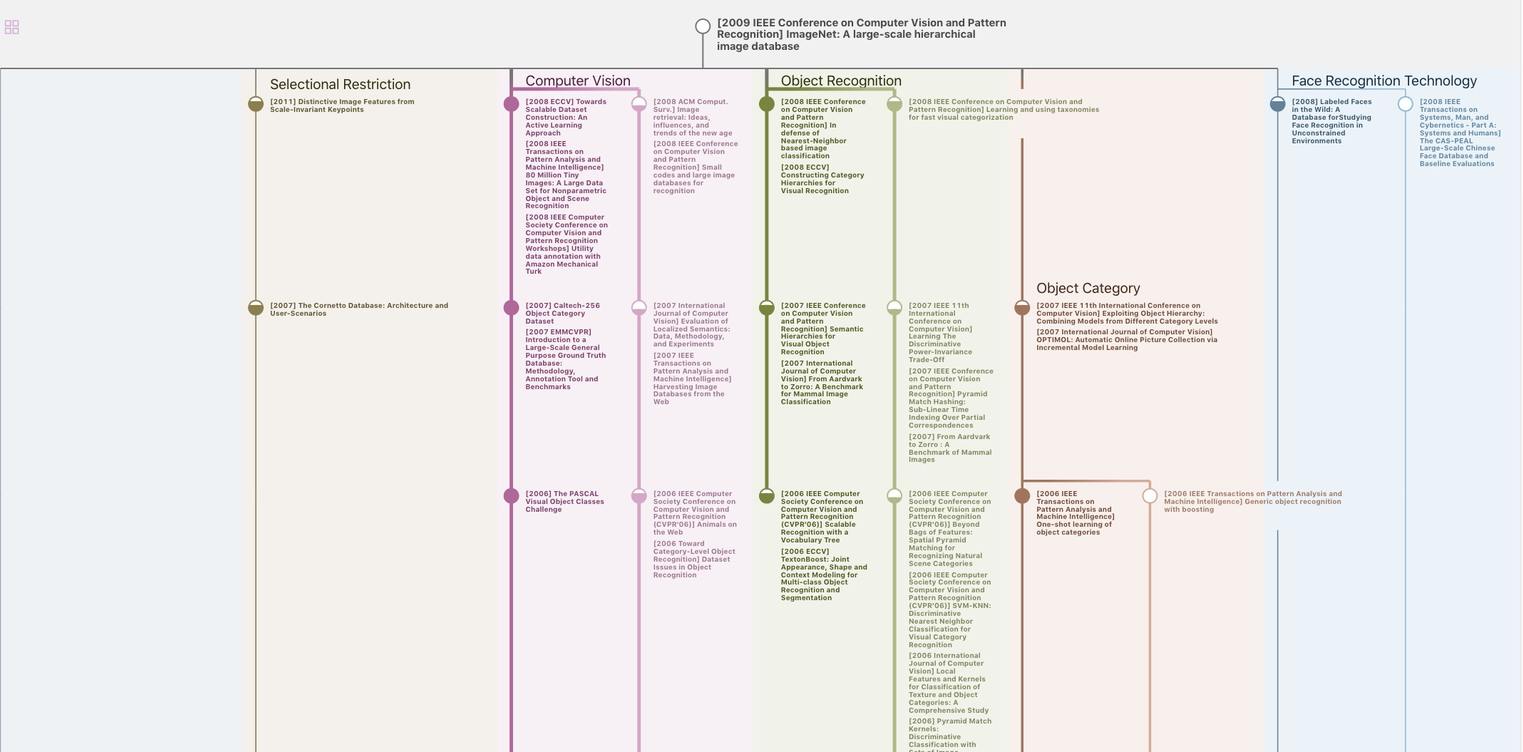
生成溯源树,研究论文发展脉络
Chat Paper
正在生成论文摘要