Pushing the Limits of Learning-based Traversability Analysis for Autonomous Driving on CPU
arxiv(2022)
摘要
Self-driving vehicles and autonomous ground robots require a reliable and accurate method to analyze the traversability of the surrounding environment for safe navigation. This paper proposes and evaluates a real-time machine learning-based Traversability Analysis method that combines geometric features with appearance-based features in a hybrid approach based on a SVM classifier. In particular, we show that integrating a new set of geometric and visual features and focusing on important implementation details enables a noticeable boost in performance and reliability. The proposed approach has been compared with state-of-the-art Deep Learning approaches on a public dataset of outdoor driving scenarios. It reaches an accuracy of 89.2% in scenarios of varying complexity, demonstrating its effectiveness and robustness. The method runs fully on CPU and reaches comparable results with respect to the other methods, operates faster, and requires fewer hardware resources.
更多查看译文
关键词
traversability analysis,autonomous driving,learning-based
AI 理解论文
溯源树
样例
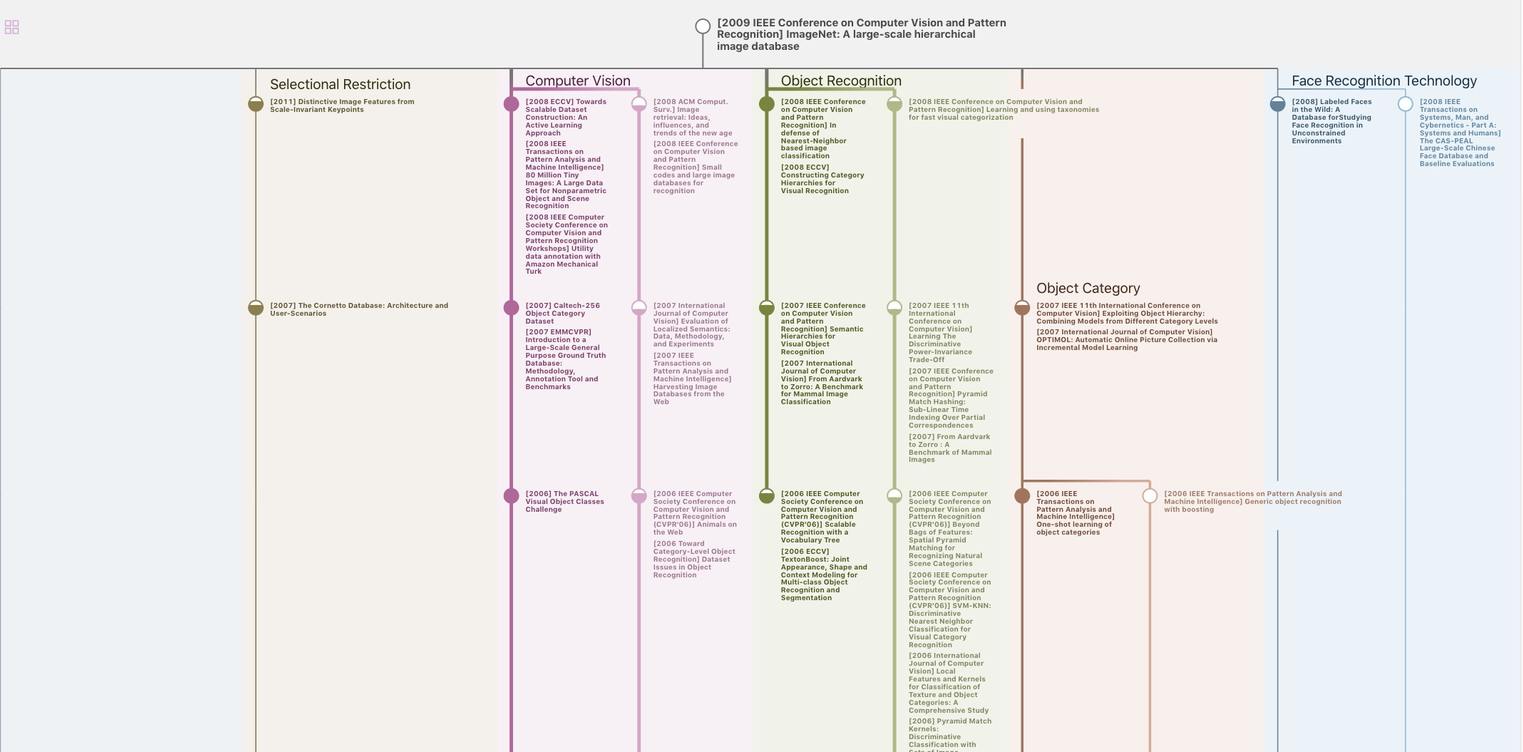
生成溯源树,研究论文发展脉络
Chat Paper
正在生成论文摘要