Shedding a PAC-Bayesian Light on Adaptive Sliced-Wasserstein Distances
arxiv(2022)
摘要
The Sliced-Wasserstein distance (SW) is a computationally efficient and theoretically grounded alternative to the Wasserstein distance. Yet, the literature on its statistical properties -- or, more accurately, its generalization properties -- with respect to the distribution of slices, beyond the uniform measure, is scarce. To bring new contributions to this line of research, we leverage the PAC-Bayesian theory and a central observation that SW may be interpreted as an average risk, the quantity PAC-Bayesian bounds have been designed to characterize. We provide three types of results: i) PAC-Bayesian generalization bounds that hold on what we refer as adaptive Sliced-Wasserstein distances, i.e. SW defined with respect to arbitrary distributions of slices (among which data-dependent distributions), ii) a principled procedure to learn the distribution of slices that yields maximally discriminative SW, by optimizing our theoretical bounds, and iii) empirical illustrations of our theoretical findings.
更多查看译文
关键词
pac-bayesian,sliced-wasserstein
AI 理解论文
溯源树
样例
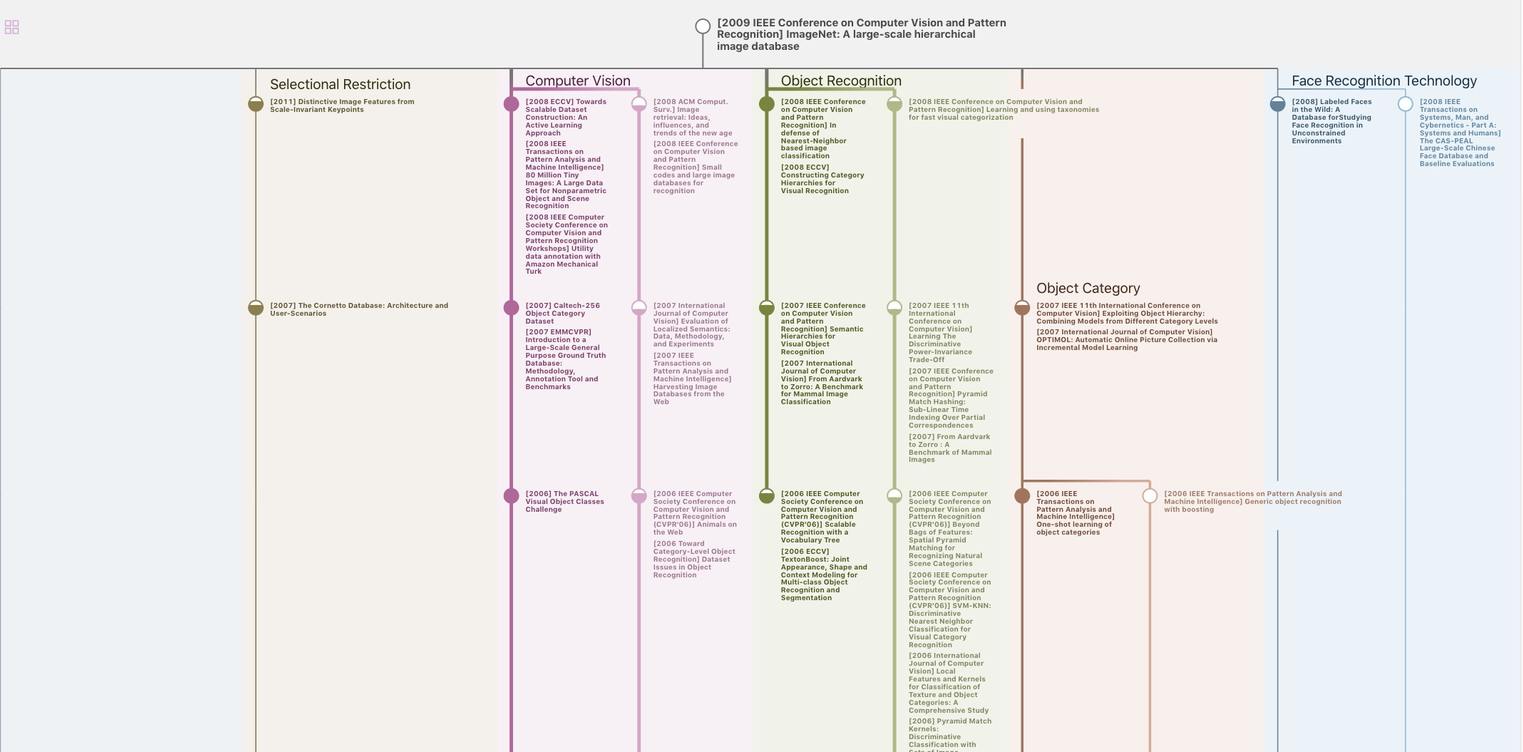
生成溯源树,研究论文发展脉络
Chat Paper
正在生成论文摘要