MIX-MAB: Reinforcement Learning-based Resource Allocation Algorithm for LoRaWAN
2022 IEEE 95TH VEHICULAR TECHNOLOGY CONFERENCE (VTC2022-SPRING)(2022)
摘要
This paper focuses on improving the resource allocation algorithm in terms of packet delivery ratio (PDR), i.e., the number of successfully received packets sent by end devices (EDs) in a long-range wide-area network (LoRaWAN). Setting the transmission parameters significantly affects the PDR. Employing reinforcement learning (RL), we propose a resource allocation algorithm that enables the EDs to configure their transmission parameters in a distributed manner. We model the resource allocation problem as a multi-armed bandit (MAB) and then address it by proposing a two-phase algorithm named MIX-MAB, which consists of the exponential weights for exploration and exploitation (EXP3) and successive elimination (SE) algorithms. We evaluate the MIX-MAB performance through simulation results and compare it with other existing approaches. Numerical results show that the proposed solution performs better than the existing schemes in terms of convergence time and PDR.
更多查看译文
关键词
IoT, LPWAN, LoRaWAN, LoRa, distributed resource allocation, reinforcement learning, multi-armed-bandit
AI 理解论文
溯源树
样例
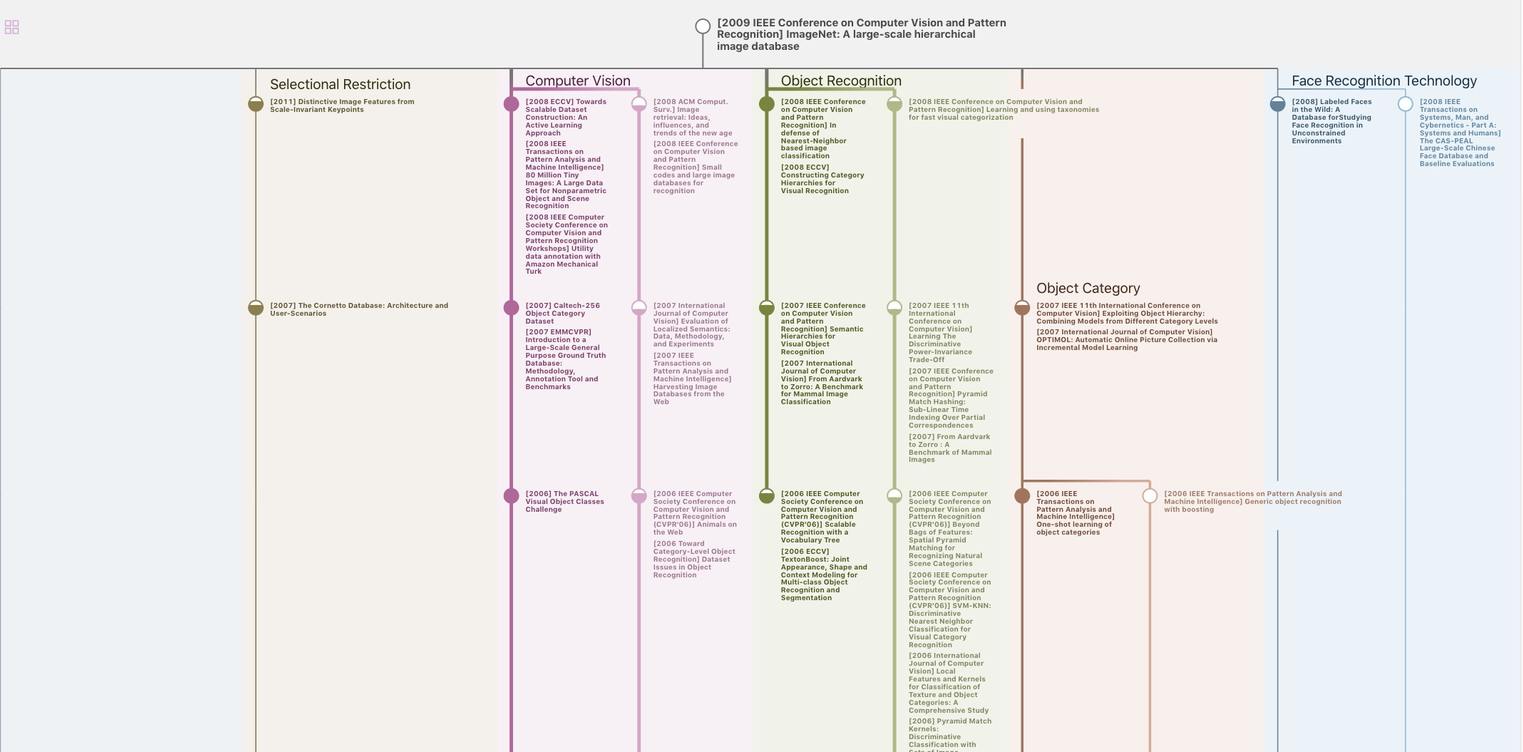
生成溯源树,研究论文发展脉络
Chat Paper
正在生成论文摘要