Multistructure Contrastive Learning for Pretraining Event Representation
IEEE TRANSACTIONS ON NEURAL NETWORKS AND LEARNING SYSTEMS(2024)
摘要
Event representation aims to transform individual events from a narrative event chain into a set of low-dimensional vectors to help support a series of downstream applications, e.g., similarity differentiation and missing event prediction. Traditional event representation models tend to focus on single modeling perspectives and thus are incapable of capturing physically disconnected yet semantically connected event segments. We, therefore, propose a heterogeneous event graph model (HeterEvent) to explicitly represent such event segments. Furthermore, another challenge in traditional event representation models is inherited from the datasets themselves. Data sparsity and insufficient labeled data are commonly encountered in event chains, easily leading to overfitting and undertraining. Therefore, we extend HeterEvent with a multistructure contrastive learning framework (MulCL) to alleviate the training risks from two structural perspectives. From the sequential perspective, a sequential-view contrastive learning component (SeqCL) is designed to facilitate the acquisition of sequential characteristics. From the graph perspective, a graph-view contrastive learning component (GraCL) is proposed to enhance the robustness of graph training by comparing different corrupted graphs. Experimental results confirm that our proposed MulCL $_{[W+E]}$ model outperforms state-of-the-art baselines. Specifically, compared with the previously proposed supervised model HeterEvent $_{[W+E]}$ [Zheng et al. (2020)], MulCL $_{[W+E]}$ shows an average improvement of 5.3% in terms of accuracy for the inference-ability-based tasks. For the representation-ability-based tasks, MulCL $_{[W+E]}$ achieves an average improvement of 2.7% in terms of accuracy for the hard similarity tasks and an improvement of 4.1% in terms of the Spearman's correlation for the transitive sentence similarity task, respectively.
更多查看译文
关键词
Training,Task analysis,Modeling,Computational modeling,Predictive models,Data models,Mutual information,Contrastive learning,event representation,graph pretraining,sequence pretraining
AI 理解论文
溯源树
样例
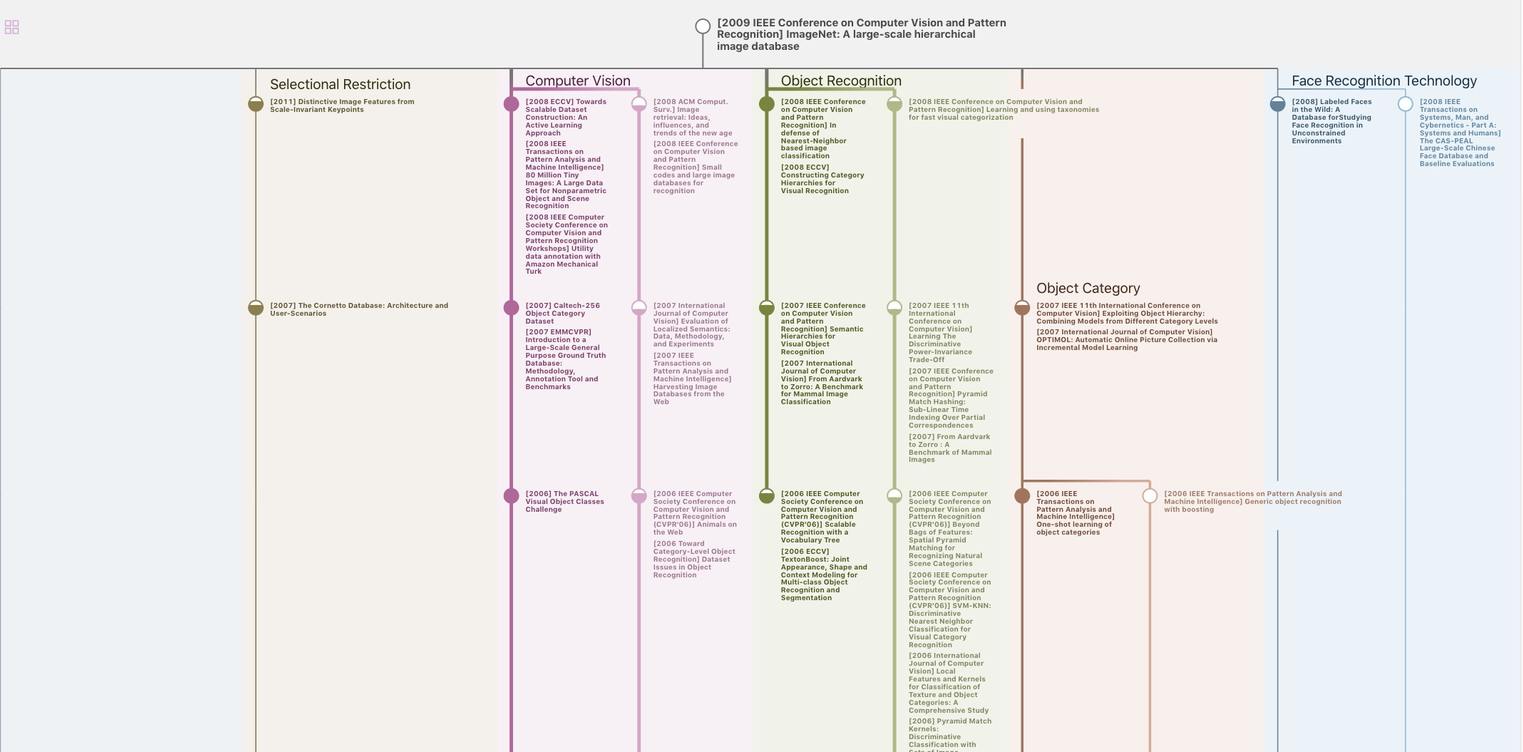
生成溯源树,研究论文发展脉络
Chat Paper
正在生成论文摘要