Contrast-Enhanced 4D MRI for Internal Target Volume Generation in Treatment Planning for Liver Tumors
International Journal of Radiation Oncology*Biology*Physics(2022)
摘要
Background
Liver tumors are often invisible on four-dimensional commuted tomography (4D-CT). Imperfect imaging surrogates are used to estimate the tumor motion. Here, we assessed multiple 4D magnetic resonance (MR) binning algorithms for directly visualizing liver tumor motion for radiotherapy planning.
Methods
Patients were simulated using a 3 Tesla MR and CT scanner. Three prototype binning algorithms (phase, amplitude, and two-directional) were applied to the 4D-MRIs, and the image quality was assessed using a qualitative clarity score and quantitative sharpness score. Radiation plans were generated for internal target volumes (ITVs) derived using 4D-MRI and 4D-CT, and the dosimetry of targets were compared. Paired t-tests were used to compare sharpness scores and dosimetric data.
Results
Twelve patients with 17 liver tumors were scanned between May and November 2021. Compared to phase binning, two-directional demonstrated equal or better clarity and sharpness scores (end-expiration: 0.33 vs 0.38, p = 0.018, end-inspiration: 0.28 vs 0.31, p = 0.010). Compared to amplitude binning, two-directional binning captured hysteresis of ≥ 3 mm in 35 % of patients. Evaluation of dosimetry CT-optimized plans revealed that PTV coverage of MR-derived targets were significantly lower than CT-derived targets (PTV receiving 90 % of prescription: 75.56 % vs 89.38 %, p = 0.002).
Conclusion
Using contrast-enhanced 4D-MRI is feasible for directly delineating liver tumors throughout the respiratory cycle. The current standard of using radiation plans optimized for 4D-CT-derived targets achieved lower coverage of directly visualized MRI targets, suggesting that adopting MRI for motion management may improve radiation treatment of liver lesions and reduce the risk of marginal misses.
更多查看译文
关键词
4D MRI,Liver tumors,Internal target volume,Motion management
AI 理解论文
溯源树
样例
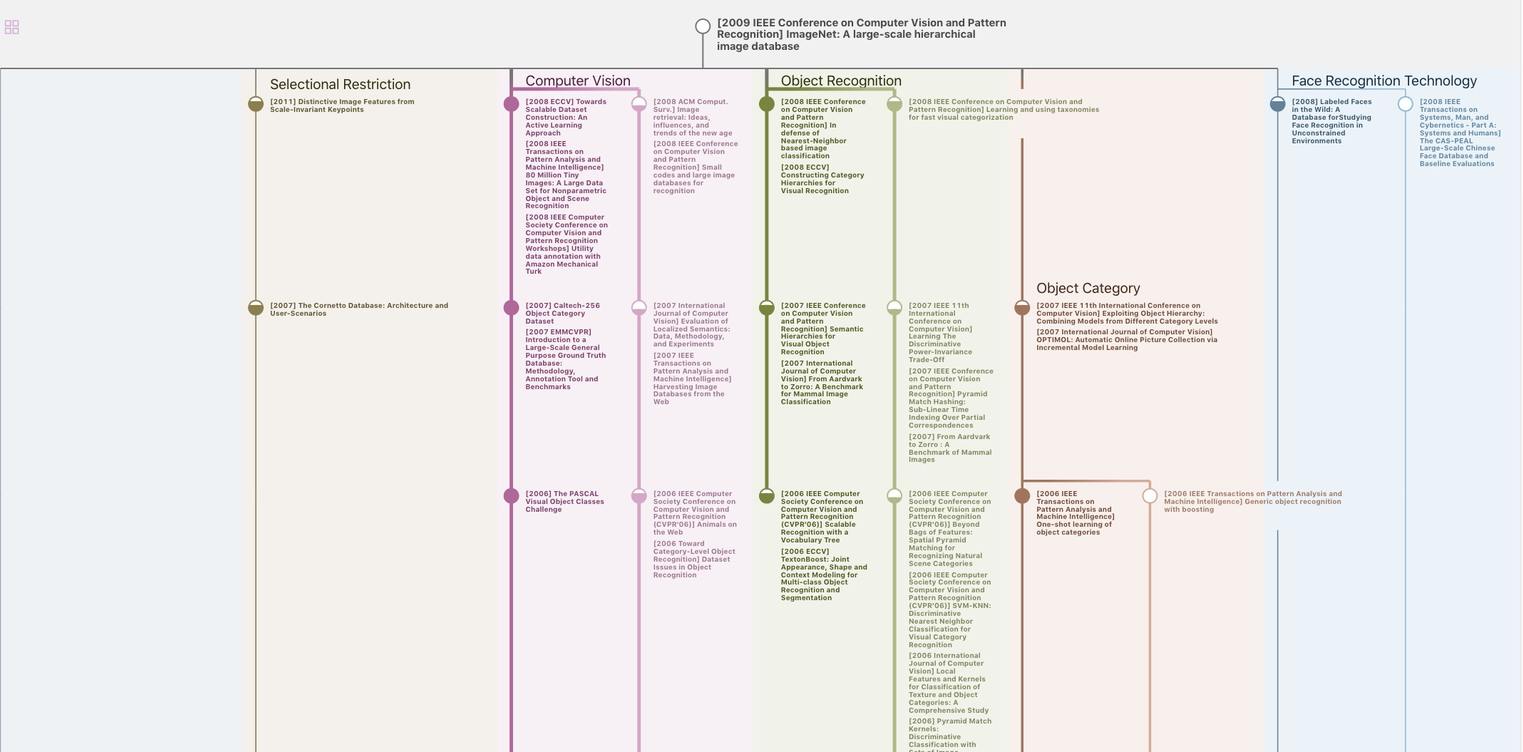
生成溯源树,研究论文发展脉络
Chat Paper
正在生成论文摘要