Linearization Autoencoder: an autoencoder-based regression model with latent space linearization
biorxiv(2022)
摘要
Regression analysis is one of the most widely applied methods in many fields including bio-medical study. Dimensionality reduction is also widely used for data preprocessing and feature selection analysis, to extract high-impact features from the predictions. As the complexity of both data and prediction models increases, it becomes important and difficult to interpret the model. We suggested a novel method, linearizing autoencoder, for regression analysis with high-dimensional data. Based on the autoencoder model, we introduce a novel loss function to make data points aggregate corresponding to their known labels and align them preserving linear relations of the known feature. This model can align data points to the linear relations of labels, and achieve both the prediction and feature selection performances by extracting features that are important to the label we want to predict. Also, we applied this method to the real-world data and the result indicates that this method can successfully disentangle the latent space with given centroids in a supervised manner. This method can be applied to various prediction problems in biomedical fields.
### Competing Interest Statement
The authors have declared no competing interest.
更多查看译文
关键词
latent space linearization,regression model,autoencoder-based
AI 理解论文
溯源树
样例
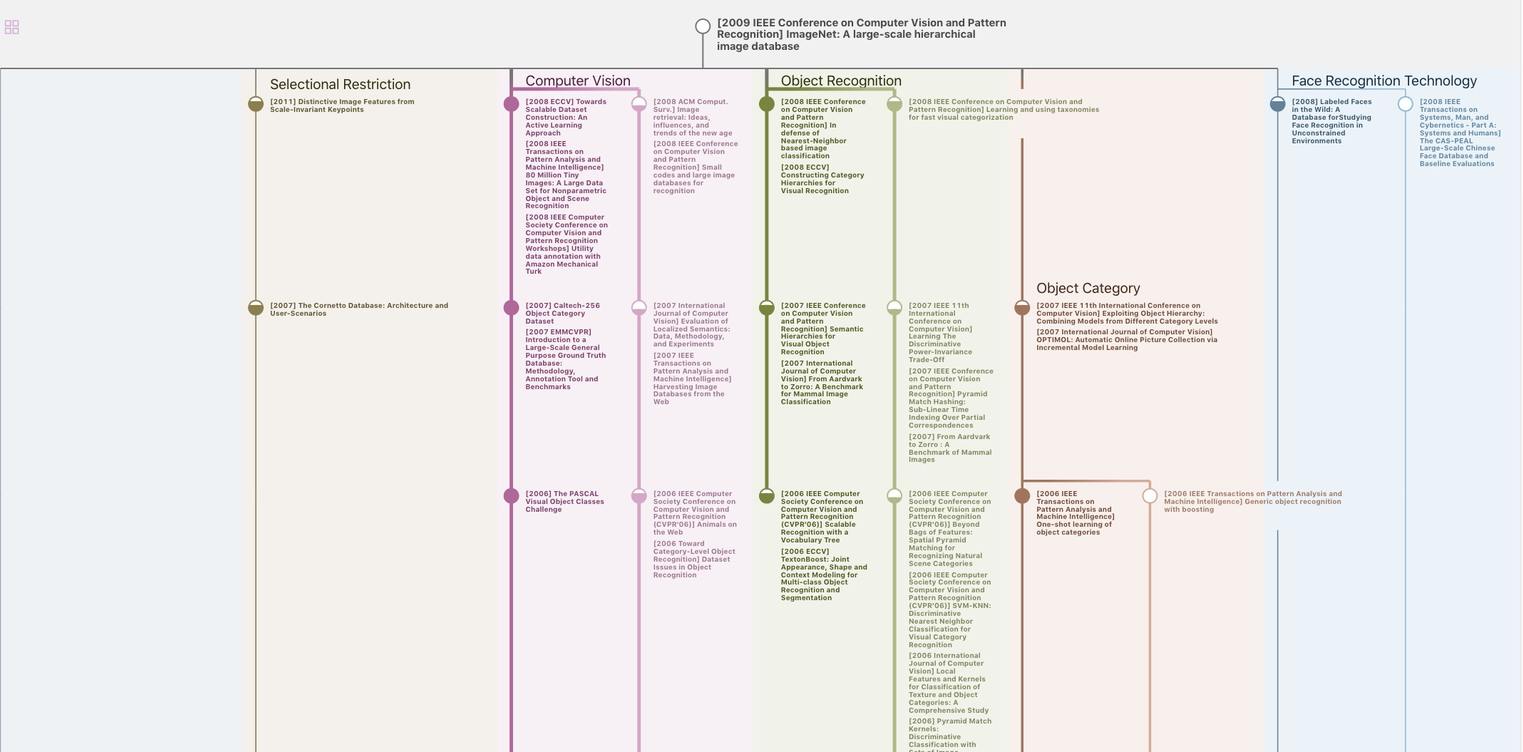
生成溯源树,研究论文发展脉络
Chat Paper
正在生成论文摘要