Single-cell Multi-Omics Integration for Unpaired Data by a Siamese Network with Graph-Based Contrastive Loss
BMC Bioinform(2023)
摘要
BACKGROUND:Single-cell omics technology is rapidly developing to measure the epigenome, genome, and transcriptome across a range of cell types. However, it is still challenging to integrate omics data from different modalities. Here, we propose a variation of the Siamese neural network framework called MinNet, which is trained to integrate multi-omics data on the single-cell resolution by using graph-based contrastive loss. RESULTS:By training the model and testing it on several benchmark datasets, we showed its accuracy and generalizability in integrating scRNA-seq with scATAC-seq, and scRNA-seq with epitope data. Further evaluation demonstrated our model's unique ability to remove the batch effect, a common problem in actual practice. To show how the integration impacts downstream analysis, we established model-based smoothing and cis-regulatory element-inferring method and validated it with external pcHi-C evidence. Finally, we applied the framework to a COVID-19 dataset to bolster the original work with integration-based analysis, showing its necessity in single-cell multi-omics research. CONCLUSIONS:MinNet is a novel deep-learning framework for single-cell multi-omics sequencing data integration. It ranked top among other methods in benchmarking and is especially suitable for integrating datasets with batch and biological variances. With the single-cell resolution integration results, analysis of the interplay between genome and transcriptome can be done to help researchers understand their data and question.
更多查看译文
关键词
Single-cell sequencing analysis,Data integration,Deep learning,COVID-19
AI 理解论文
溯源树
样例
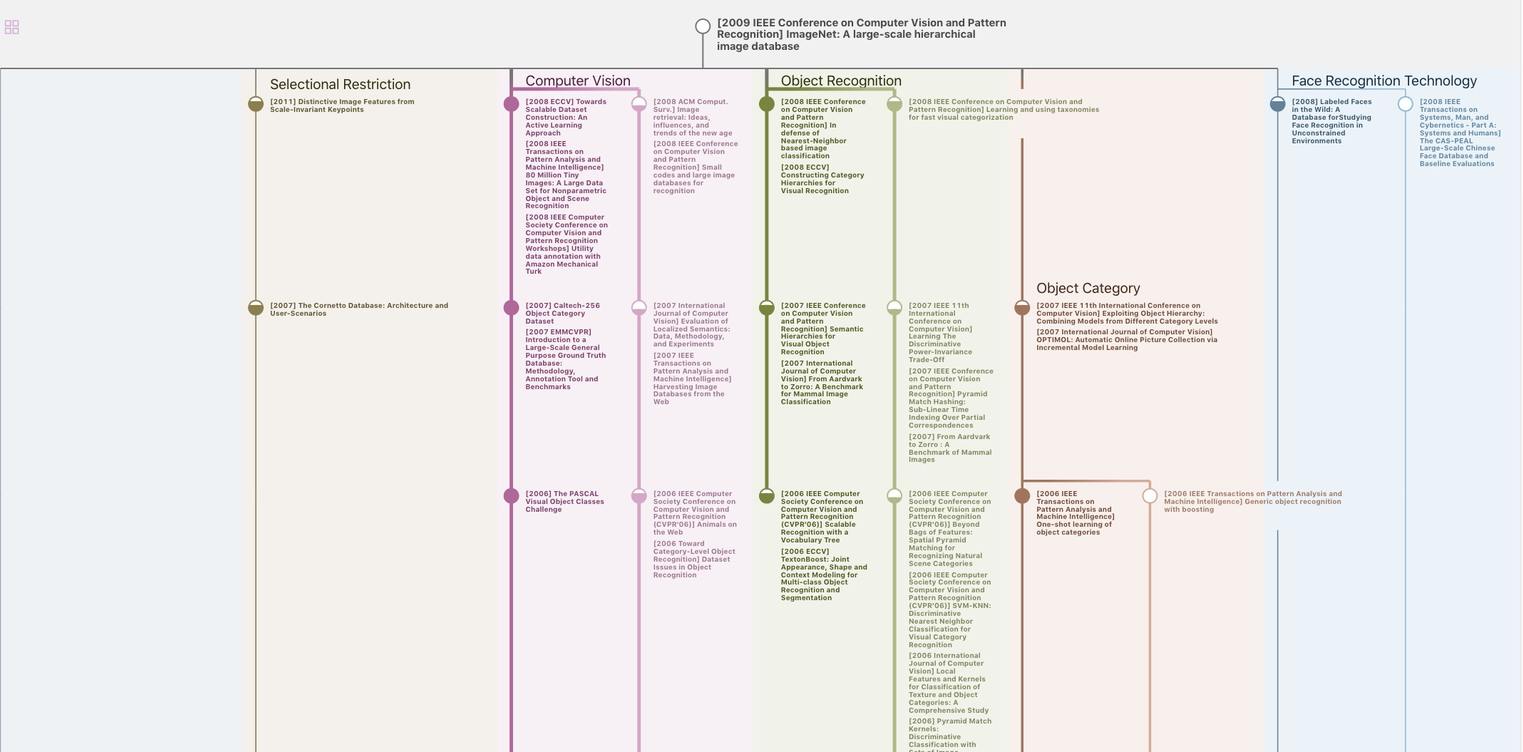
生成溯源树,研究论文发展脉络
Chat Paper
正在生成论文摘要