Machine Learning Study of Metabolic Networks vs ChEMBL Data of Antibacterial Compounds.
Molecular pharmaceutics(2022)
摘要
Antibacterial drugs (AD) change the metabolic status of bacteria, contributing to bacterial death. However, antibiotic resistance and the emergence of multidrug-resistant bacteria increase interest in understanding metabolic network (MN) mutations and the interaction of AD MN. In this study, we employed the IFPTML = Information Fusion (IF) + Perturbation Theory (PT) + Machine Learning (ML) algorithm on a huge dataset from the ChEMBL database, which contains >155,000 AD assays >40 MNs of multiple bacteria species. We built a linear discriminant analysis (LDA) and 17 ML models centered on the linear index and based on atoms to predict antibacterial compounds. The IFPTML-LDA model presented the following results for the training subset: specificity (Sp) = 76% out of 70,000 cases, sensitivity (Sn) = 70%, and Accuracy (Acc) = 73%. The same model also presented the following results for the validation subsets: Sp = 76%, Sn = 70%, and Acc = 73.1%. Among the IFPTML nonlinear models, the k nearest neighbors (KNN) showed the best results with Sn = 99.2%, Sp = 95.5%, Acc = 97.4%, and Area Under Receiver Operating Characteristic (AUROC) = 0.998 in training sets. In the validation series, the Random Forest had the best results: Sn = 93.96% and Sp = 87.02% (AUROC = 0.945). The IFPTML linear and nonlinear models regarding the ADs MNs have good statistical parameters, and they could contribute toward finding new metabolic mutations in antibiotic resistance and reducing time/costs in antibacterial drug research.
更多查看译文
关键词
ChEMBL,antibacterial compounds,complex networks,information fusion,machine learning,multidrug-resistant,perturbation theory
AI 理解论文
溯源树
样例
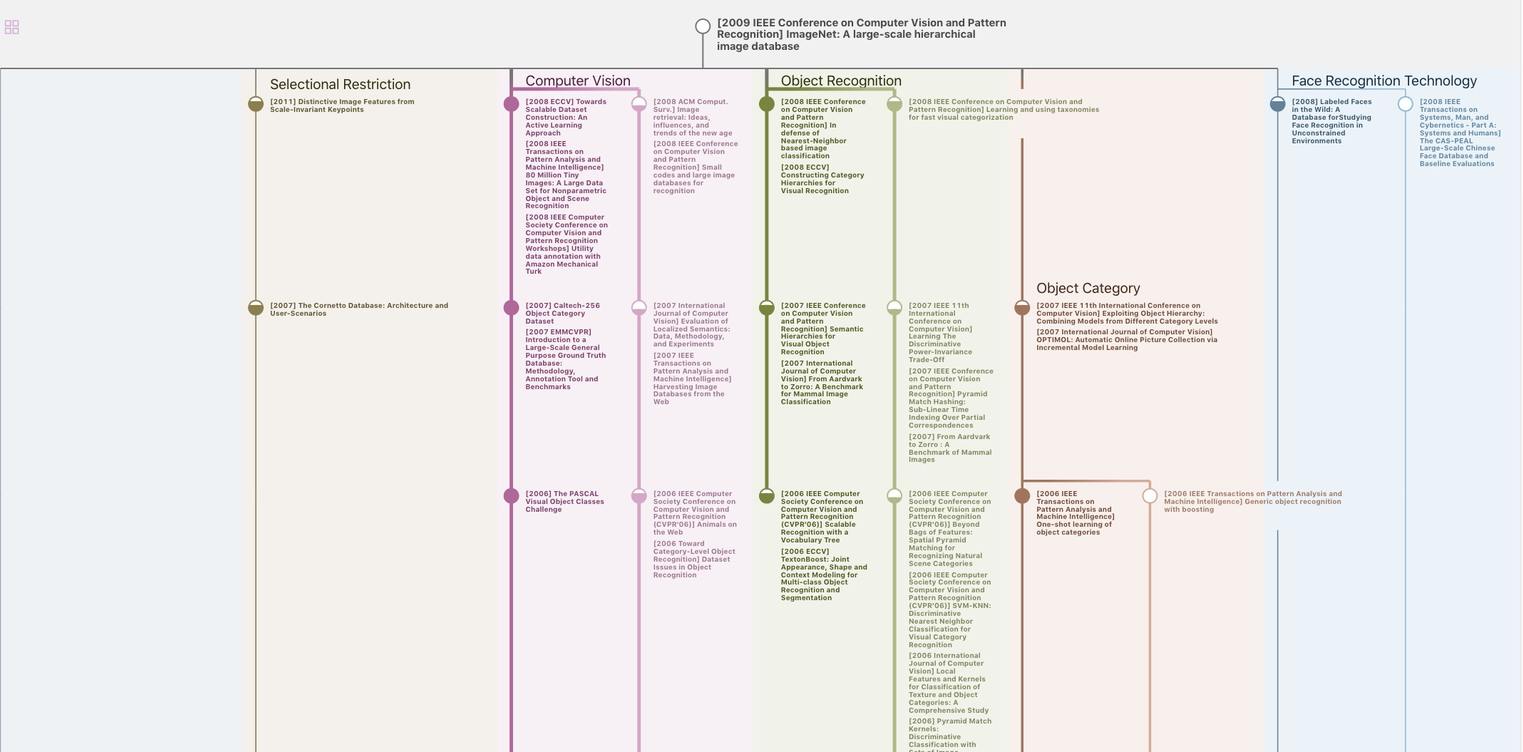
生成溯源树,研究论文发展脉络
Chat Paper
正在生成论文摘要