A Survey of Graph-Based Deep Learning for Anomaly Detection in Distributed Systems
IEEE TRANSACTIONS ON KNOWLEDGE AND DATA ENGINEERING(2024)
摘要
Anomaly detection is a crucial task in complex distributed systems. A thorough understanding of the requirements and challenges of anomaly detection is pivotal to the security of such systems, especially for real-world deployment. While there are many works and application domains that deal with this problem, few have attempted to provide an in-depth look at such systems. In this survey, we explore the potentials of graph-based algorithms to identify anomalies in distributed systems. These systems can be heterogeneous or homogeneous, which can result in distinct requirements. One of our objectives is to provide an in-depth look at graph-based approaches to conceptually analyze their capability to handle real-world challenges such as heterogeneity and dynamic structure. This study gives an overview of the State-of-the-Art (SotA) research articles in the field and compare and contrast their characteristics. To facilitate a more comprehensive understanding, we present three systems with varying abstractions as use cases. We examine the specific challenges involved in anomaly detection within such systems. Subsequently, we elucidate the efficacy of graphs in such systems and explicate their advantages. We then delve into the SotA methods and highlight their strength and weaknesses, pointing out the areas for possible improvements and future works.
更多查看译文
关键词
Anomaly detection,deep learning,distributed systems,dynamic systems,graphs,heterogeneous systems
AI 理解论文
溯源树
样例
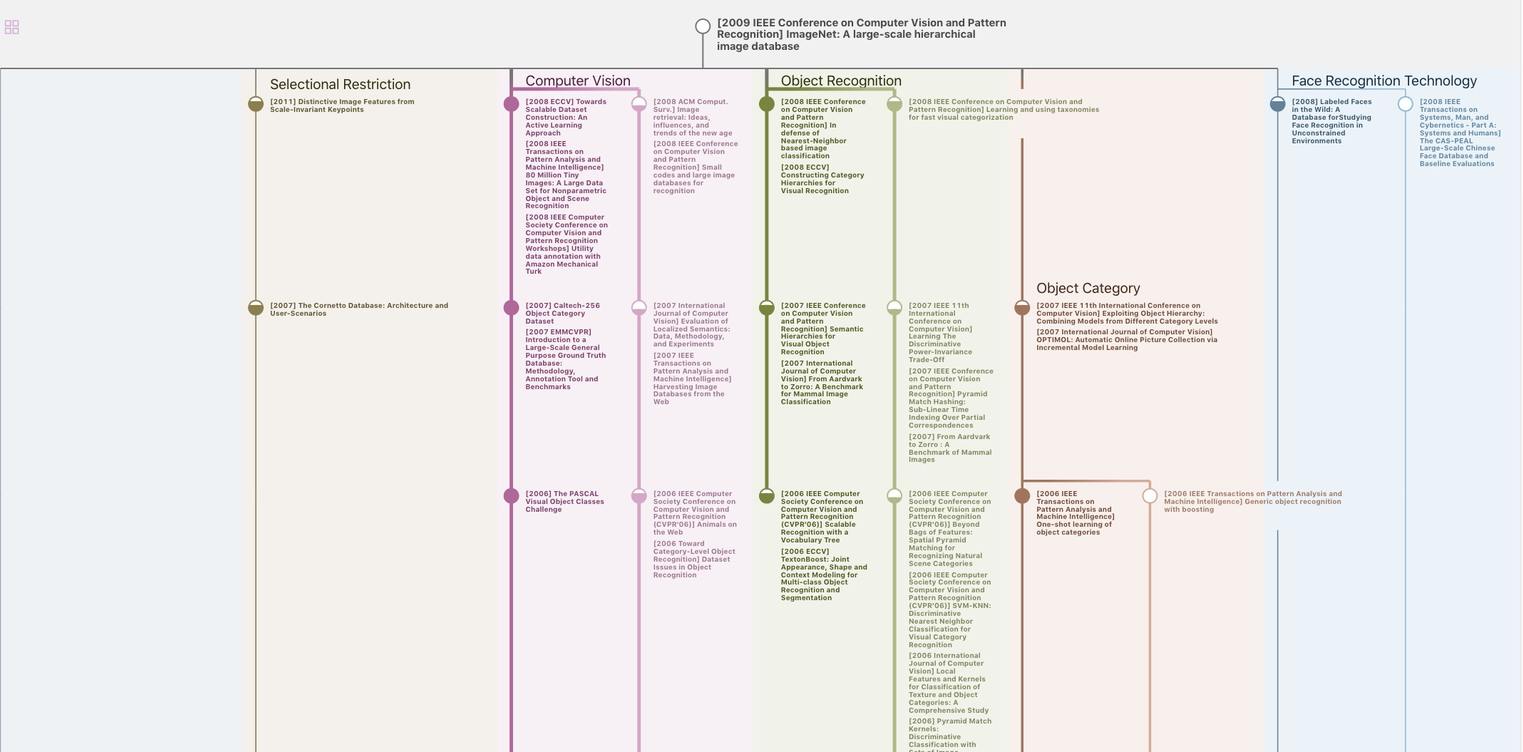
生成溯源树,研究论文发展脉络
Chat Paper
正在生成论文摘要