Classification of COVID-19 in Chest X-ray Images Using Fusion of Deep Features and LightGBM
2022 IEEE World AI IoT Congress (AIIoT)(2022)
摘要
The COVID-19 disease was first discovered in Wuhan, China, and spread quickly worldwide. After the COVID-19 pandemic, many researchers have begun to identify a way to diagnose the COVID-19 using chest X-ray images. The early diagnosis of this disease can significantly impact the treatment process. In this article, we propose a new technique that is faster and more accurate than the other methods reported in the literature. The proposed method uses a combination of DenseNet169 and MobileNet Deep Neural Networks to extract the features of the patient's X-ray images. Using the univariate feature selection algorithm, we refined the features for the most important ones. Then we applied the selected features as input to the LightGBM (Light Gradient Boosting Machine) algorithm for classification. To assess the effectiveness of the proposed method, the ChestX-ray8 dataset, which includes 1125 X-ray images of the patient's chest, was used. The proposed method achieved 98.54% and 91.11% accuracies in the two-class (COVID-19, Healthy) and multi-class (COVID-19, Healthy, Pneumonia) classification problems, respectively. It is worth mentioning that we have used Gradient-weighted Class Activation Mapping (Grad-CAM) for future analysis.
更多查看译文
关键词
COVID-19,DenseNet169,MobileNet,Light-GBM,Univariate Feature Selection,GradCAM
AI 理解论文
溯源树
样例
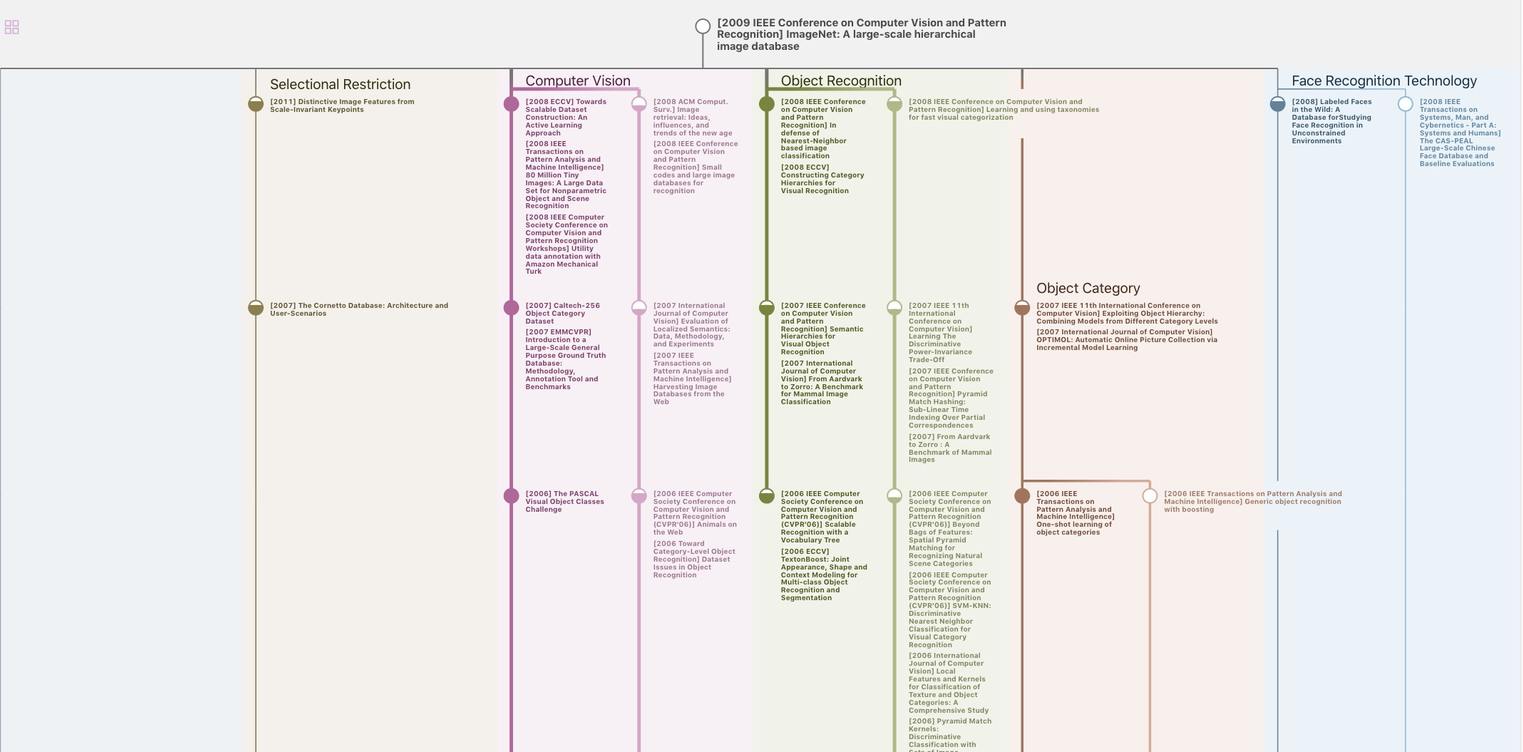
生成溯源树,研究论文发展脉络
Chat Paper
正在生成论文摘要