Significance tests for R2 of out-of-sample prediction using polygenic scores
American journal of human genetics(2022)
摘要
The coefficient of determination (R2) is a well-established measure to indicate the predictive ability of polygenic scores (PGS). However, the sampling variance of R2 is rarely considered so that 95% confidence intervals (CI) are not usually reported. Moreover, when comparisons are made between PGS based on different discovery samples, the sampling covariance of R2 is necessary to test the difference between them. Here, we show how to estimate the variance and covariance of R2 values to assess the 95% CI and p-value of the R2 difference. We apply this approach to real data to predict into 28,880 European participants using UK Biobank (UKBB) and Biobank Japan (BBJ) GWAS summary statistics for cholesterol and BMI. We quantify the significantly higher predictive ability of UKBB PGS compared to BBJ PGS (p-value 7.6e-31 for cholesterol and 1.4e-50 for BMI). A joint model of UKBB and BBJ PGS significantly improves the predictive ability, compared to a model of UKBB PGS only (p-value 3.5e-05 for cholesterol and 1.3e-28 for BMI). The proposed approach can also be applied to testing a significant difference between R2 values across different p-value thresholds. We also show that the predictive ability of regulatory SNPs is significantly enriched than non-regulatory SNPs for cholesterol (p-value 2.6e-19 for UKBB and 8.7e-08 for BBJ). We suggest that the proposed approach (available in R package r2redux) should be used to test the statistical significance of difference between pairs of PGS, which may help to draw a correct conclusion about the predictive ability of PGS.
### Competing Interest Statement
The authors have declared no competing interest.
更多查看译文
关键词
coefficient of determination, R(2), polygenic scores, PGSs, sampling variance, sampling covariance, significance test, non-nested model comparison, R(2)-based genomic partitioning
AI 理解论文
溯源树
样例
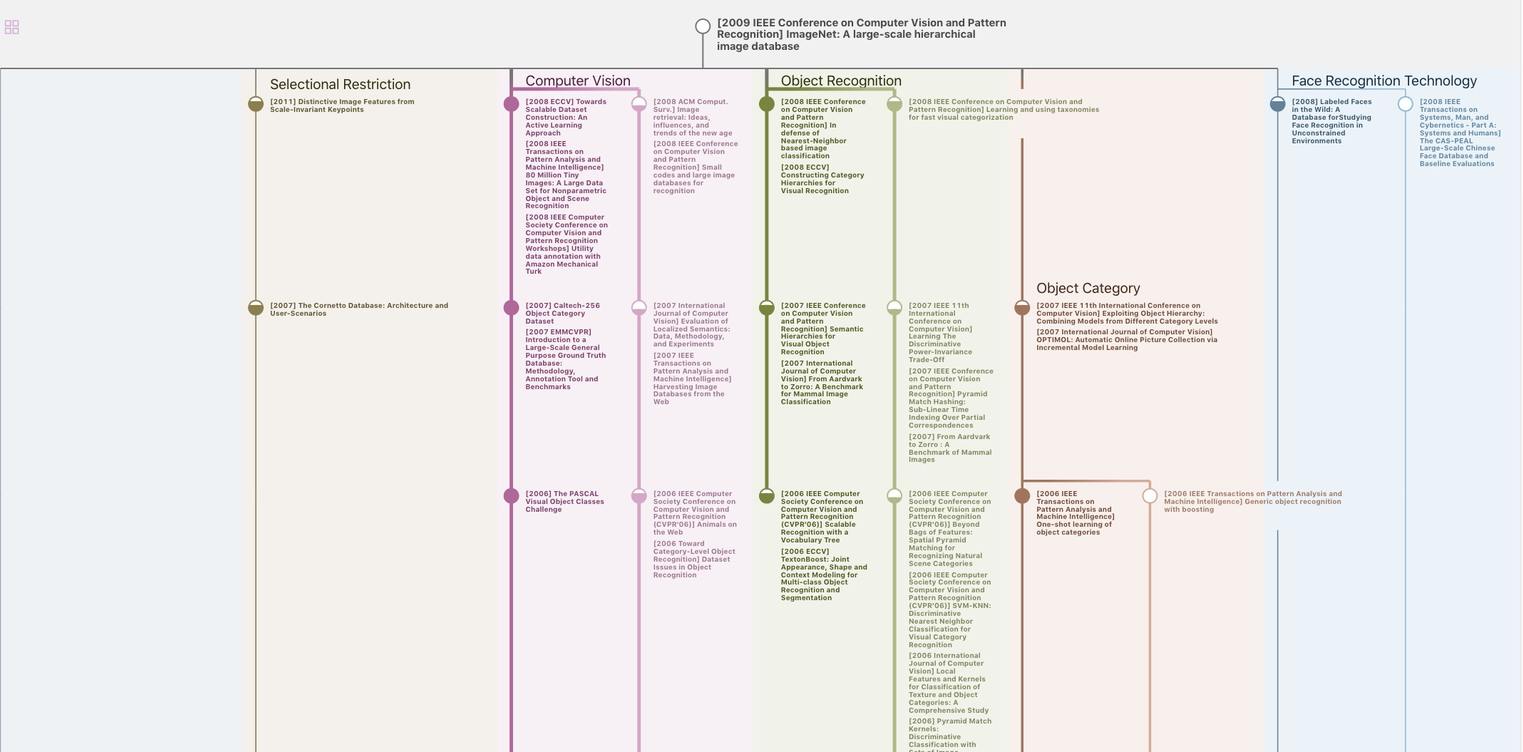
生成溯源树,研究论文发展脉络
Chat Paper
正在生成论文摘要