Added Value of Viscoelasticity for MRI-Based Prediction of Ki-67 Expression of Hepatocellular Carcinoma Using a Deep Learning Combined Radiomics (DLCR) Model
CANCERS(2022)
摘要
Simple Summary This study aimed to explore the added value of magnetic resonance elastography (MRE) in the prediction of Ki-67 expression in hepatocellular carcinoma (HCC) using a deep learning combined radiomics (DLCR) model. A total of 108 histopathology-proven HCC patients who underwent preoperative MRI and MR elastography were included. All the patients were divided into training and validation cohorts. An independent cohort including 43 patients was included for testing. A DLCR model was proposed to predict the expression of Ki-67 with conventional MRI (cMRI) as inputs. The images of shear wave speed (c-map) and phase angle (phi-map) derived from MRE were also fed into the DLCR model. Experimental results show that both c and phi values were ranked within the top six features for Ki-67 prediction with random forest selection, which revealed the value of MRE-based viscosity for the assessment of the tumor proliferation status in HCC. The model with all modalities (MRE, AFP, and cMRI) as inputs achieved the highest AUC of 0.90 +/- 0.03 (CI: 0.89-0.91) in the validation cohort. The same finding was observed in the independent testing cohort with an AUC of 0.83 +/- 0.03 (CI: 0.82-0.84). MRE-based c and phi-maps can serve as important parameters to assess the tumor proliferation status in HCC. This study aimed to explore the added value of viscoelasticity measured by magnetic resonance elastography (MRE) in the prediction of Ki-67 expression in hepatocellular carcinoma (HCC) using a deep learning combined radiomics (DLCR) model. This retrospective study included 108 histopathology-proven HCC patients (93 males; age, 59.6 +/- 11.0 years) who underwent preoperative MRI and MR elastography. They were divided into training (n = 87; 61.0 +/- 9.8 years) and testing (n = 21; 60.6 +/- 10.1 years) cohorts. An independent validation cohort including 43 patients (60.1 +/- 11.3 years) was included for testing. A DLCR model was proposed to predict the expression of Ki-67 with cMRI, including T2W, DW, and dynamic contrast enhancement (DCE) images as inputs. The images of the shear wave speed (c-map) and phase angle (phi-map) derived from MRE were also fed into the DLCR model. The Ki-67 expression was classified into low and high groups with a threshold of 20%. Both c and phi values were ranked within the top six features for Ki-67 prediction with random forest selection, which revealed the value of MRE-based viscosity for the assessment of tumor proliferation status in HCC. When comparing the six CNN models, Xception showed the best performance for classifying the Ki-67 expression, with an AUC of 0.80 +/- 0.03 (CI: 0.79-0.81) and accuracy of 0.77 +/- 0.04 (CI: 0.76-0.78) when cMRI were fed into the model. The model with all modalities (MRE, AFP, and cMRI) as inputs achieved the highest AUC of 0.90 +/- 0.03 (CI: 0.89-0.91) in the validation cohort. The same finding was observed in the independent testing cohort, with an AUC of 0.83 +/- 0.03 (CI: 0.82-0.84). The shear wave speed and phase angle improved the performance of the DLCR model significantly for Ki-67 prediction, suggesting that MRE-based c and phi-maps can serve as important parameters to assess the tumor proliferation status in HCC.
更多查看译文
关键词
Ki-67, hepatocellular carcinoma (HCC), conventional MRI (cMRI), magnetic resonance elastography (MRE), deep learning combined radiomics (DLCR)
AI 理解论文
溯源树
样例
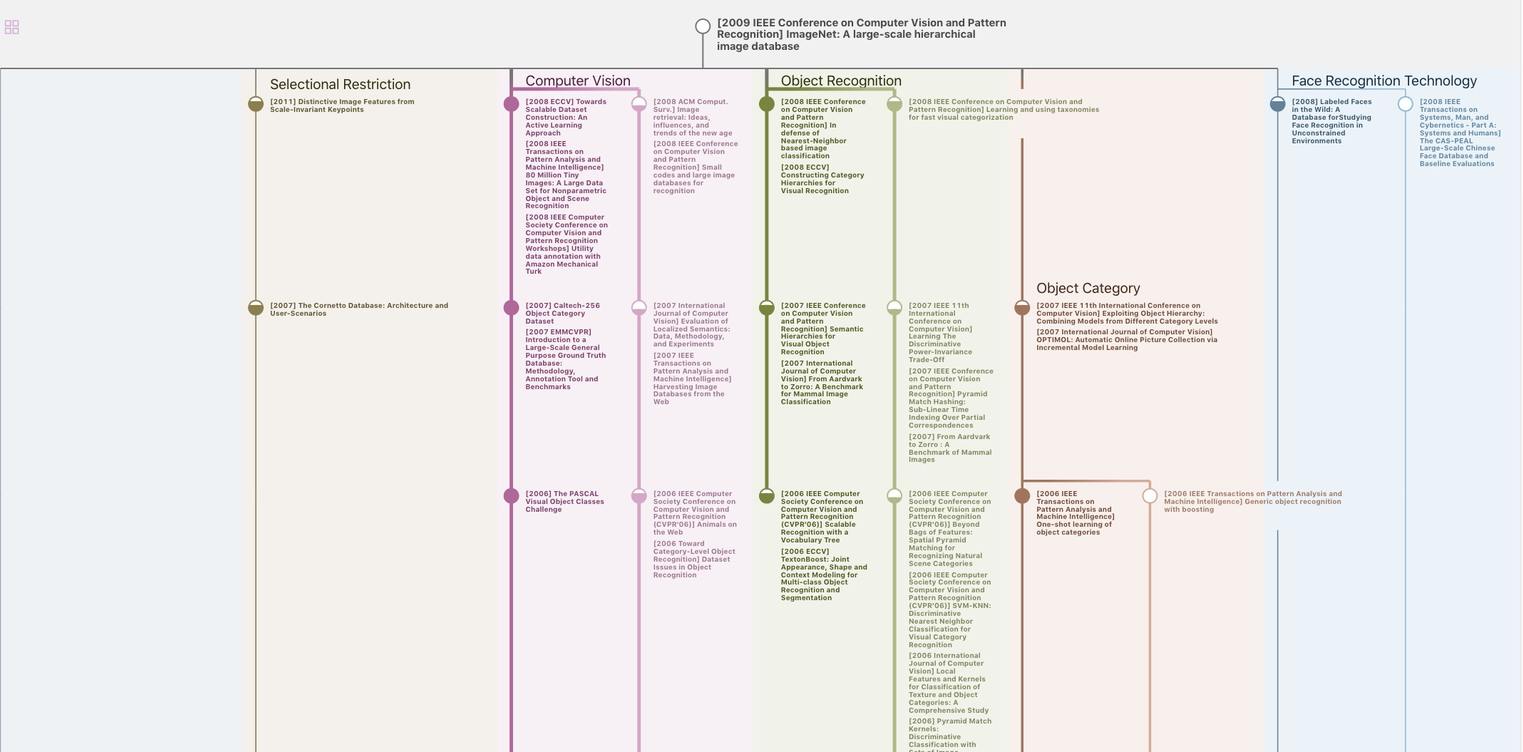
生成溯源树,研究论文发展脉络
Chat Paper
正在生成论文摘要