Convolutional Neural Network Accelerator for Compression Based on Simon K-Means
IEEE International Joint Conference on Neural Network(2022)
摘要
Convolutional Neural Networks (CNN) are popular models widely used in image classification, target recognition, and other fields. FPGA-based accelerators for CNN are a standard method in recent years to reduce CNN's inference time and energy efficiency. However, the limitations of on-chip storage space and computing resources introduce deep compression. Contrary to most compression algorithms that pay no attention to the underlying hardware acceleration strategy and hardware-only accelerators, this paper introduces a novel model compression scheme with software and hardware collaboration for accelerating inference. First, we propose a pre-processing algorithm named Simon k-means based on clustering to quantify trained weight to speed up inference. Next, we propose a new encoding method for the quantized weight, significantly reducing the model's storage size. Finally, we give the architecture design of the accelerator using the quantized weight to accelerate the convolution. We have evaluated many popular CNNs in image classification tasks on various data sets. Experiments show that the number of multiply-accumulate operations on the convolutional layer can be reduced 66.6% with a slight loss of precision.
更多查看译文
关键词
convolutional neural networks,deep learning,k-means,model compression,weight quantization
AI 理解论文
溯源树
样例
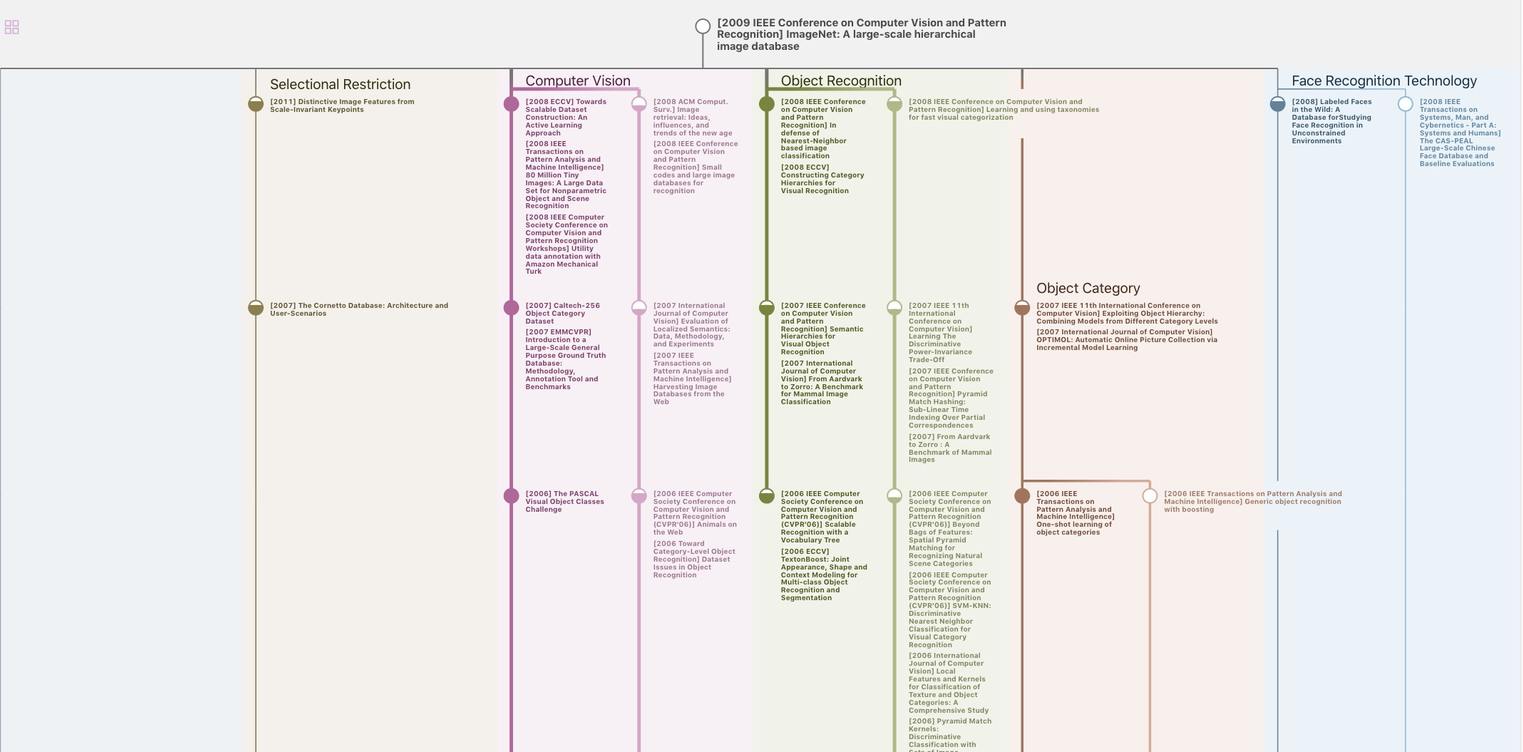
生成溯源树,研究论文发展脉络
Chat Paper
正在生成论文摘要