Persistent Homology with k-nearest-neighbor Filtrations reveals Topological Convergence of PageRank
arxiv(2022)
摘要
Graph-based representations of point-cloud data are widely used in data science and machine learning, including epsilon-graphs that contain edges between pairs of data points that are nearer than epsilon and kNN-graphs that connect each point to its k-nearest neighbors. Recently, topological data analysis has emerged as a family of mathematical and computational techniques to investigate topological features of data using simplicial complexes. These are a higher-order generalization of graphs and many techniques such as Vietoris-Rips (VR) filtrations are also parameterized by a distance epsilon. Here, we develop kNN complexes as a generalization of kNN graphs, leading to kNN-based persistent homology techniques for which we develop stability and convergence results. We apply this technique to characterize the convergence properties PageRank, highlighting how the perspective of discrete topology complements traditional geometrical-based analyses of convergence. Specifically, we show that convergence of relative positions (i.e., ranks) is captured by kNN persistent homology, whereas persistent homology with VR filtrations coincides with vector-norm convergence. Beyond PageRank, kNN-based persistent homology is expected to be useful to other data-science applications in which the relative positioning of data points is more important than their precise locations.
更多查看译文
AI 理解论文
溯源树
样例
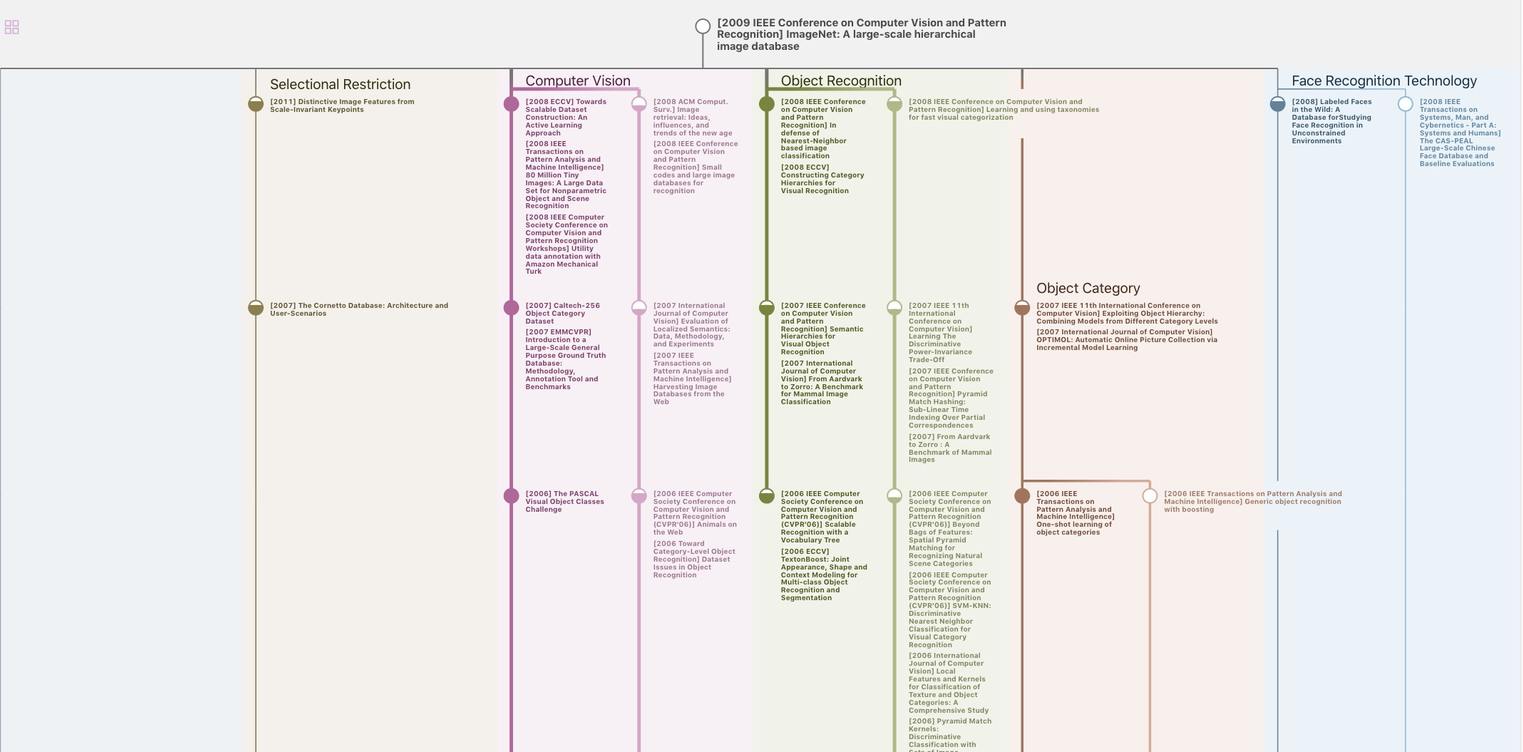
生成溯源树,研究论文发展脉络
Chat Paper
正在生成论文摘要