Squeeze All: Novel Estimator and Self-Normalized Bound for Linear Contextual Bandits
arxiv(2023)
摘要
We propose a linear contextual bandit algorithm with $O(\sqrt{dT\log T})$ regret bound, where $d$ is the dimension of contexts and $T$ isthe time horizon. Our proposed algorithm is equipped with a novel estimator in which exploration is embedded through explicit randomization. Depending on the randomization, our proposed estimator takes contributions either from contexts of all arms or from selected contexts. We establish a self-normalized bound for our estimator, which allows a novel decomposition of the cumulative regret into \textit{additive} dimension-dependent terms instead of multiplicative terms. We also prove a novel lower bound of $\Omega(\sqrt{dT})$ under our problem setting. Hence, the regret of our proposed algorithm matches the lower bound up to logarithmic factors. The numerical experiments support the theoretical guarantees and show that our proposed method outperforms the existing linear bandit algorithms.
更多查看译文
关键词
novel estimator,bound,self-normalized
AI 理解论文
溯源树
样例
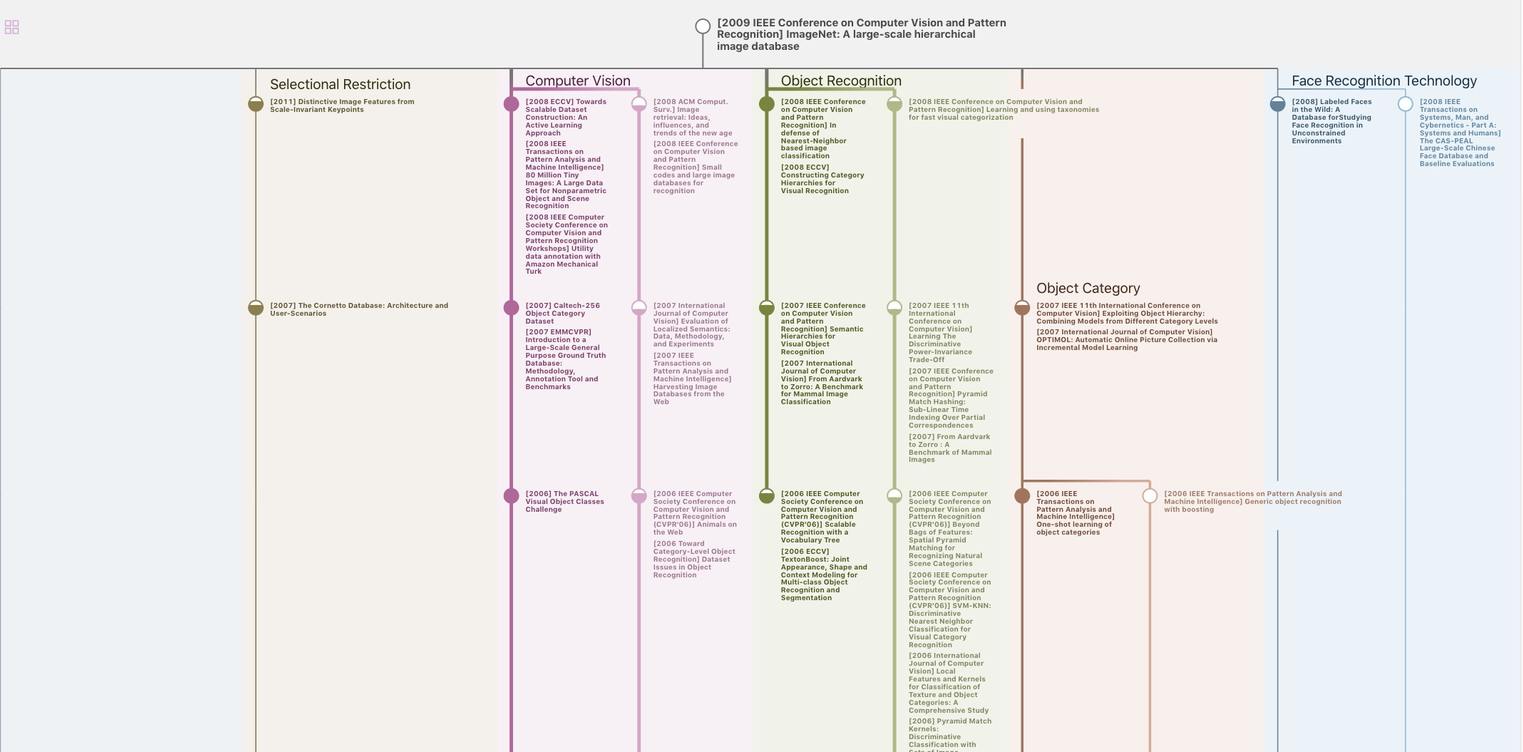
生成溯源树,研究论文发展脉络
Chat Paper
正在生成论文摘要