ChordMixer: A Scalable Neural Attention Model for Sequences with Different Lengths
ICLR 2023(2022)
摘要
Sequential data naturally have different lengths in many domains, with some very long sequences. As an important modeling tool, neural attention should capture long-range interaction in such sequences. However, most existing neural attention models admit only short sequences, or they have to employ chunking or padding to enforce a constant input length. Here we propose a simple neural network building block called ChordMixer which can model the attention for long sequences with variable lengths. Each ChordMixer block consists of a position-wise rotation layer without learnable parameters and an element-wise MLP layer. Repeatedly applying such blocks forms an effective network backbone that mixes the input signals towards the learning targets. We have tested ChordMixer on the synthetic adding problem, long document classification, and DNA sequence-based taxonomy classification. The experiment results show that our method substantially outperforms other neural attention models.
更多查看译文
关键词
Mixer,Attention,Scalable
AI 理解论文
溯源树
样例
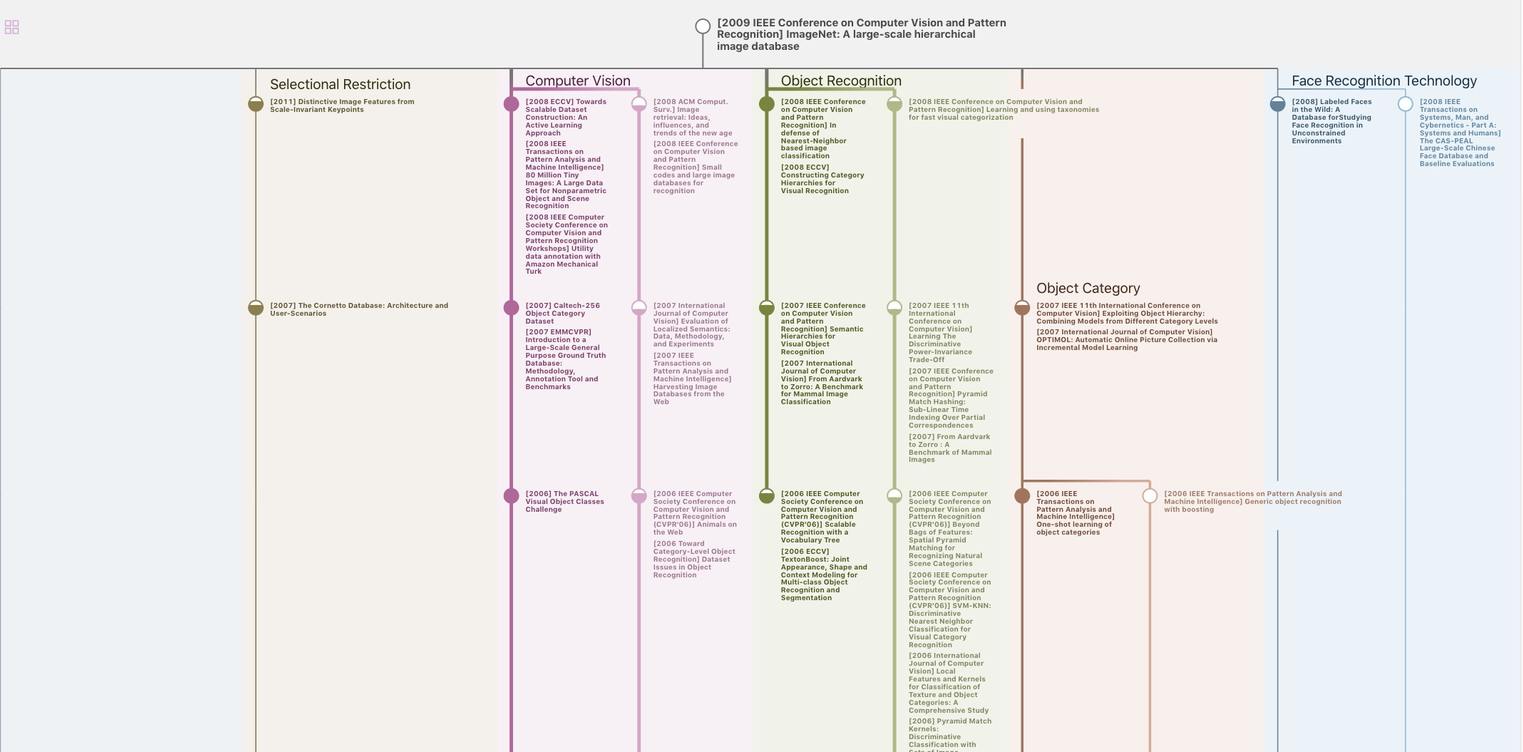
生成溯源树,研究论文发展脉络
Chat Paper
正在生成论文摘要