SIXO: Smoothing Inference with Twisted Objectives
NeurIPS 2022(2022)
摘要
Sequential Monte Carlo (SMC) is an inference algorithm for state space models that approximates the posterior by sampling from a sequence of intermediate target distributions. The target distributions are often chosen to be the filtering distributions, but these ignore information from future observations, leading to practical and theoretical limitations in inference and model learning. We introduce SIXO, a method that instead learns targets that approximate the smoothing distributions, incorporating information from all observations. The key idea is to use density ratio estimation to fit functions that warp the filtering distributions into the smoothing distributions. We then use SMC with these learned targets to define a variational objective for model and proposal learning. SIXO yields provably tighter log marginal lower bounds and offers significantly more accurate posterior inferences and parameter estimates in a variety of domains.
更多查看译文
关键词
smoothing,variational,objectives,fivo,sequential Monte Carlo,inference,twisted,time series
AI 理解论文
溯源树
样例
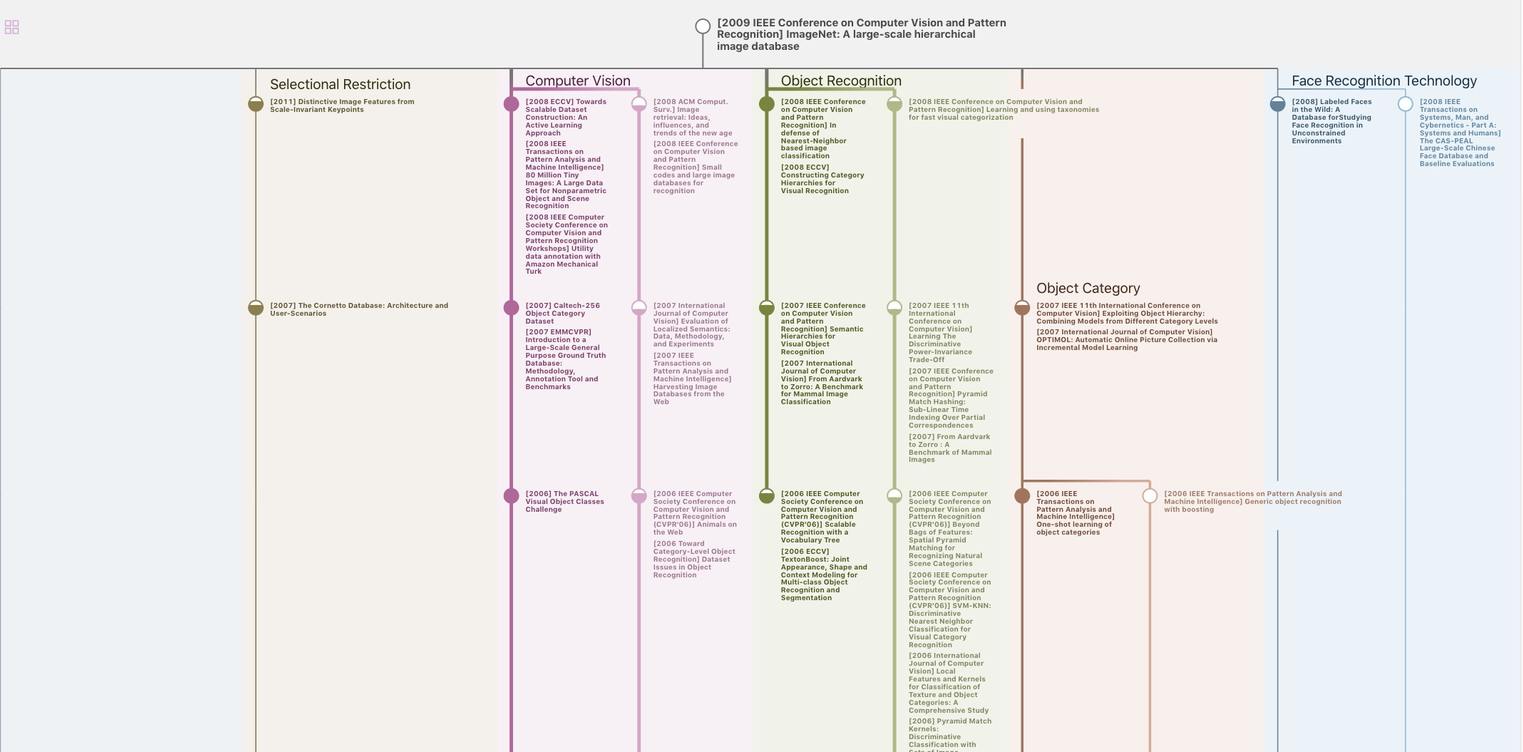
生成溯源树,研究论文发展脉络
Chat Paper
正在生成论文摘要