A Novel Key Features Screening Method Based on Extreme Learning Machine for Alzheimer’s Disease Study
Frontiers in aging neuroscience(2022)
摘要
The Extreme Learning Machine (ELM) is a simple and efficient Single Hidden Layer Feedforward Neural Network(SLFN) algorithm. In recent years, it has been gradually used in the study of Alzheimer’s disease (AD). When using ELM to diagnose AD based on high-dimensional features, there are often some features that have no positive impact on the diagnosis, while others have a significant impact on the diagnosis. In this paper, a novel Key Features Screening Method based on Extreme Learning Machine (KFS-ELM) is proposed. It can screen for key features that are relevant to the classification (diagnosis). It can also assign weights to key features based on their importance. We designed an experiment to screen for key features of AD. A total of 920 key functional connections screened from 4005 functional connections. Their weights were also obtained. The results of the experiment showed that: (1) Using all (4,005) features to diagnose AD, the accuracy is 95.33%. Using 920 key features to diagnose AD, the accuracy is 99.20%. The 3,085 (4,005 - 920) features that were screened out had a negative effect on the diagnosis of AD. This indicates the KFS-ELM is effective in screening key features. (2) The higher the weight of the key features and the smaller their number, the greater their impact on AD diagnosis. This indicates that the KFS-ELM is rational in assigning weights to the key features for their importance. Therefore, KFS-ELM can be used as a tool for studying features and also for improving classification accuracy.
更多查看译文
关键词
fMRI,brain functional connectivity,extreme learning machine,AD,KFS-ELM
AI 理解论文
溯源树
样例
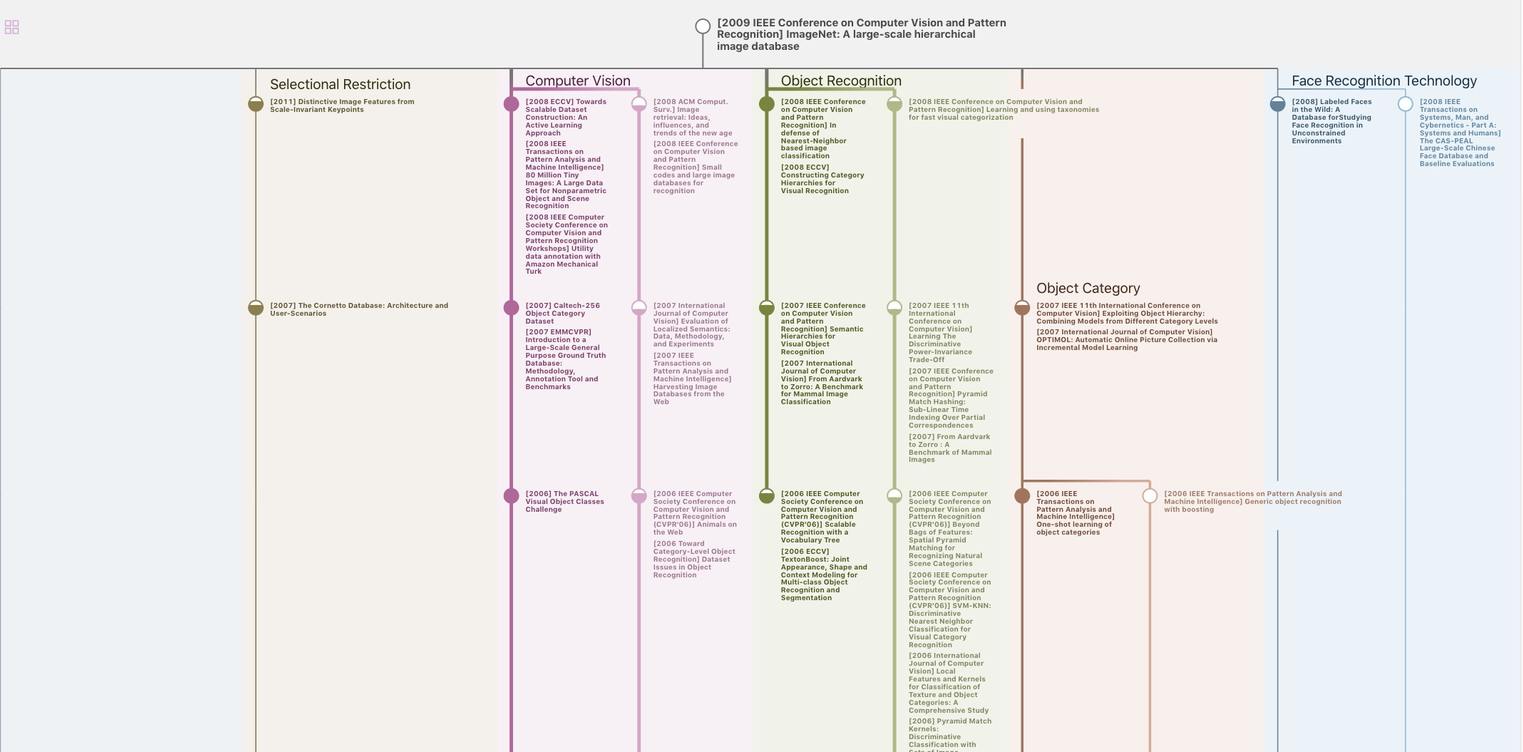
生成溯源树,研究论文发展脉络
Chat Paper
正在生成论文摘要