Global Analysis of Deep Learning Prediction Using Large-Scale In-House Kinome-Wide Profiling Data
ACS OMEGA(2022)
摘要
In drug discovery, the prediction of activity and absorption, distribution, metabolism, excretion, and toxicity parameters is one of the most important approaches in determining which compound to synthesize next. In recent years, prediction methods based on deep learning as well as non-deep learning approaches have been established, and a number of applications to drug discovery have been reported by various companies and organizations. In this research, we performed activity prediction using deep learning and non-deep learning methods on in-house assay data for several hundred kinases and compared and discussed the prediction results. We found that the prediction accuracy of the single-task graph neural network (GNN) model was generally lower than that of the non-deep learning model (LightGBM), but the multitask GNN model, which combined data from other kinases, comprehensively outperformed LightGBM. In addition, the extrapolative validity of the multitask model was verified by using it for prediction on known kinase ligands. We observed an overlap between characteristic protein-ligand interaction sites and the atoms that are important for prediction. By building appropriate models based on the conditions of the data set and analyzing the feature importance of the prediction results, a ligand-based prediction method may be used not only for activity prediction but also for drug design.
更多查看译文
AI 理解论文
溯源树
样例
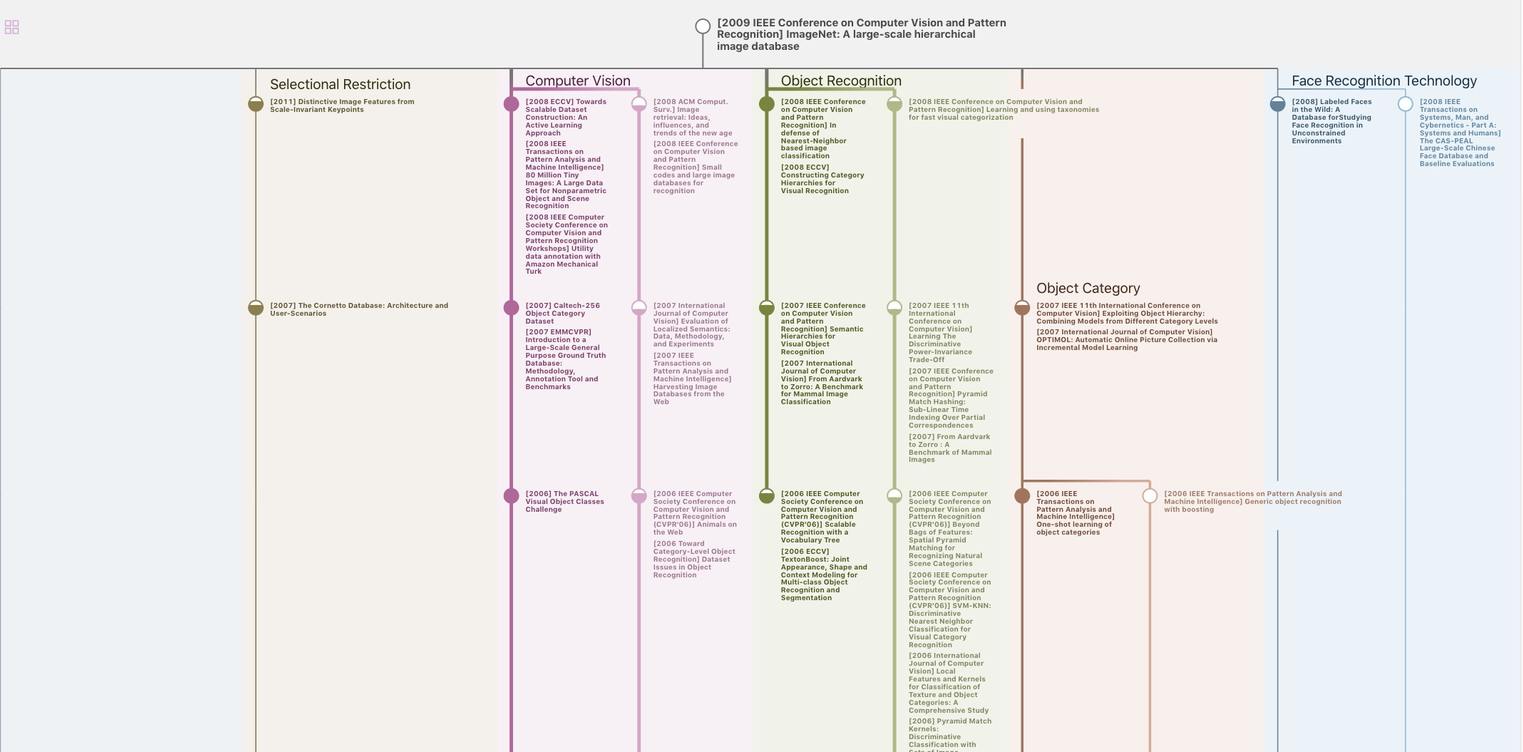
生成溯源树,研究论文发展脉络
Chat Paper
正在生成论文摘要