Probabilistic Conformal Prediction Using Conditional Random Samples
arxiv(2022)
摘要
This paper proposes probabilistic conformal prediction (PCP), a predictive inference algorithm that estimates a target variable by a discontinuous predictive set. Given inputs, PCP construct the predictive set based on random samples from an estimated generative model. It is efficient and compatible with either explicit or implicit conditional generative models. Theoretically, we show that PCP guarantees correct marginal coverage with finite samples. Empirically, we study PCP on a variety of simulated and real datasets. Compared to existing methods for conformal inference, PCP provides sharper predictive sets.
更多查看译文
关键词
conditional random samples,prediction,probabilistic
AI 理解论文
溯源树
样例
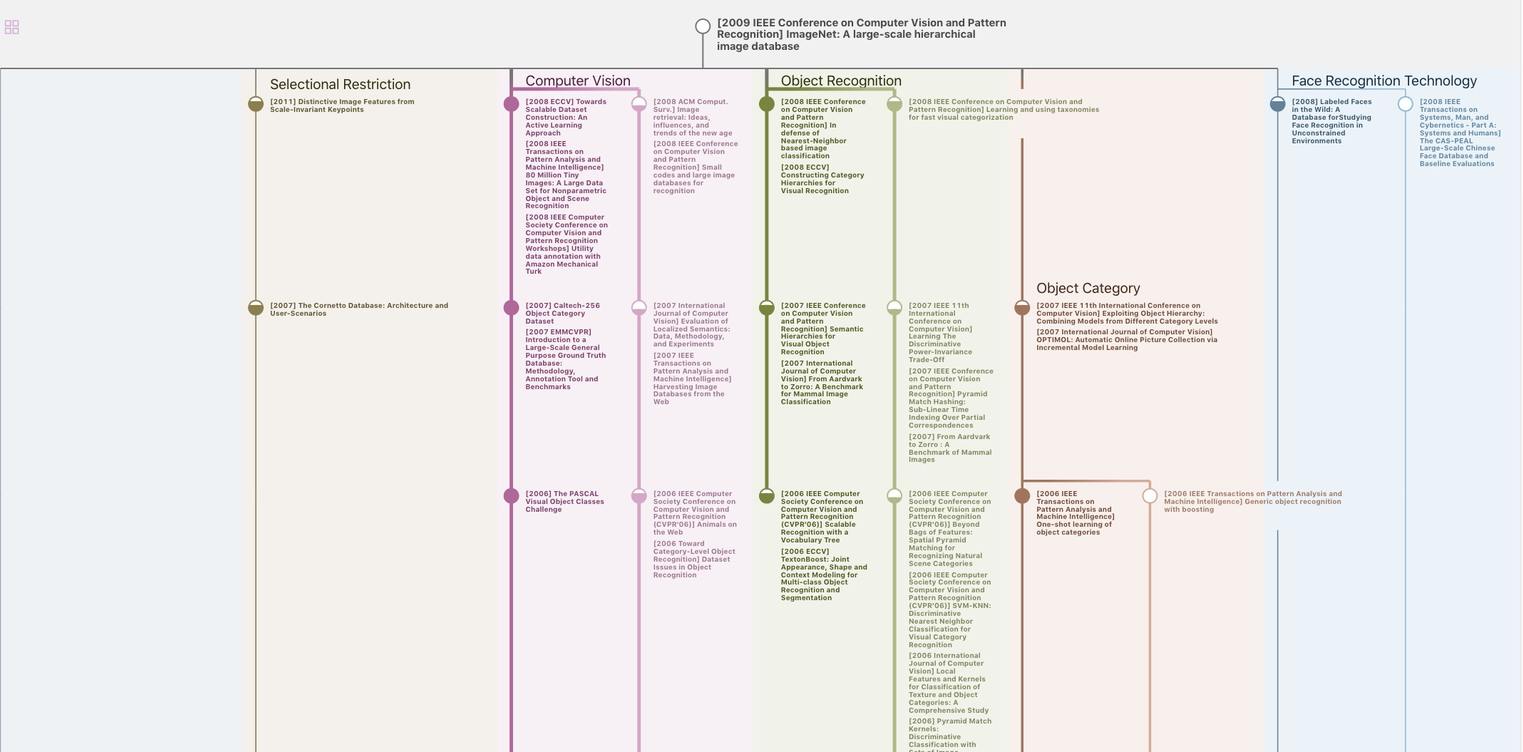
生成溯源树,研究论文发展脉络
Chat Paper
正在生成论文摘要