A Projection-Based K-space Transformer Network for Undersampled Radial MRI Reconstruction with Limited Training Subjects
MEDICAL IMAGE COMPUTING AND COMPUTER ASSISTED INTERVENTION, MICCAI 2022, PT VI(2022)
摘要
The recent development of deep learning combined with compressed sensing enables fast reconstruction of undersampled MR images and has achieved state-of-the-art performance for Cartesian k-space trajectories. However, non-Cartesian trajectories such as the radial trajectory need to be transformed onto a Cartesian grid in each iteration of the network training, slowing down the training process significantly. Multiple iterations of nonuniform Fourier transformation in the networks offset the advantage of fast inference inherent in deep learning. Current approaches typically either work on image-to-image networks or grid the non-Cartesian trajectories before the network training to avoid the repeated gridding process. However, the image-to-image networks cannot ensure the k-space data consistency in the reconstructed images and the pre-processing of non-Cartesian k-space leads to gridding errors which cannot be compensated by the network training. Inspired by the Transformer network to handle long-range dependencies in sequence transduction tasks, we propose to rearrange the radial spokes to sequential data based on the chronological order of acquisition and use the Transformer network to predict unacquired radial spokes from the acquired data. We propose novel data augmentation methods to generate a large amount of training data from a limited number of subjects. The network can be applied to different anatomical structures. Experimental results show superior performance of the proposed framework compared to state-of-the-art deep neural networks.
更多查看译文
关键词
Transformer network, MRI Reconstruction, Radial MRI
AI 理解论文
溯源树
样例
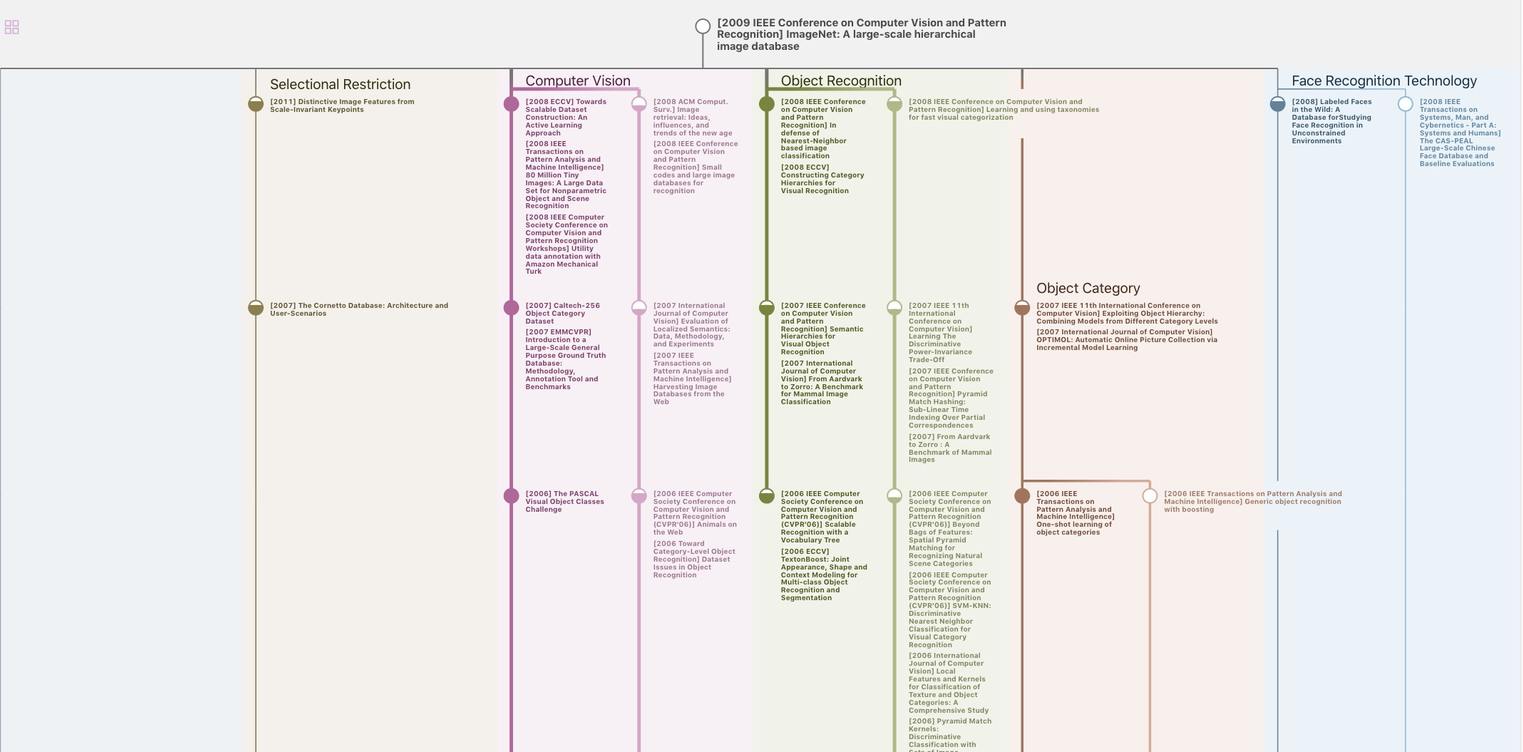
生成溯源树,研究论文发展脉络
Chat Paper
正在生成论文摘要