Deep Neural Network Pruning for Nuclei Instance Segmentation in Hematoxylin and Eosin-Stained Histological Images
APPLICATIONS OF MEDICAL ARTIFICIAL INTELLIGENCE, AMAI 2022(2022)
摘要
Recently, pruning deep neural networks (DNNs) has received a lot of attention for improving accuracy and generalization power, reducing network size, and increasing inference speed on specialized hardwares. Although pruning was mainly tested on computer vision tasks, its application in the context of medical image analysis has hardly been explored. This work investigates the impact of well-known pruning techniques, namely layer-wise and network-wide magnitude pruning, on the nuclei instance segmentation performance in histological images. Our utilised instance segmentation model consists of two main branches: (1) a semantic segmentation branch, and (2) a deep regression branch. We investigate the impact of weight pruning on the performance of both branches separately, and on the final nuclei instance segmentation result. Evaluated on two publicly available datasets, our results show that layer-wise pruning delivers slightly better performance than network-wide pruning for small compression ratios (CRs) while for large CRs, network-wide pruning yields superior performance. For semantic segmentation, deep regression and final instance segmentation, 93.75%, 95%, and 80% of the model weights can be pruned by layer-wise pruning with less than 2% reduction in the performance of respective models.
更多查看译文
关键词
Neural networks, Pruning, Nuclei segmentation, Machine learning, Deep learning, Medical imaging
AI 理解论文
溯源树
样例
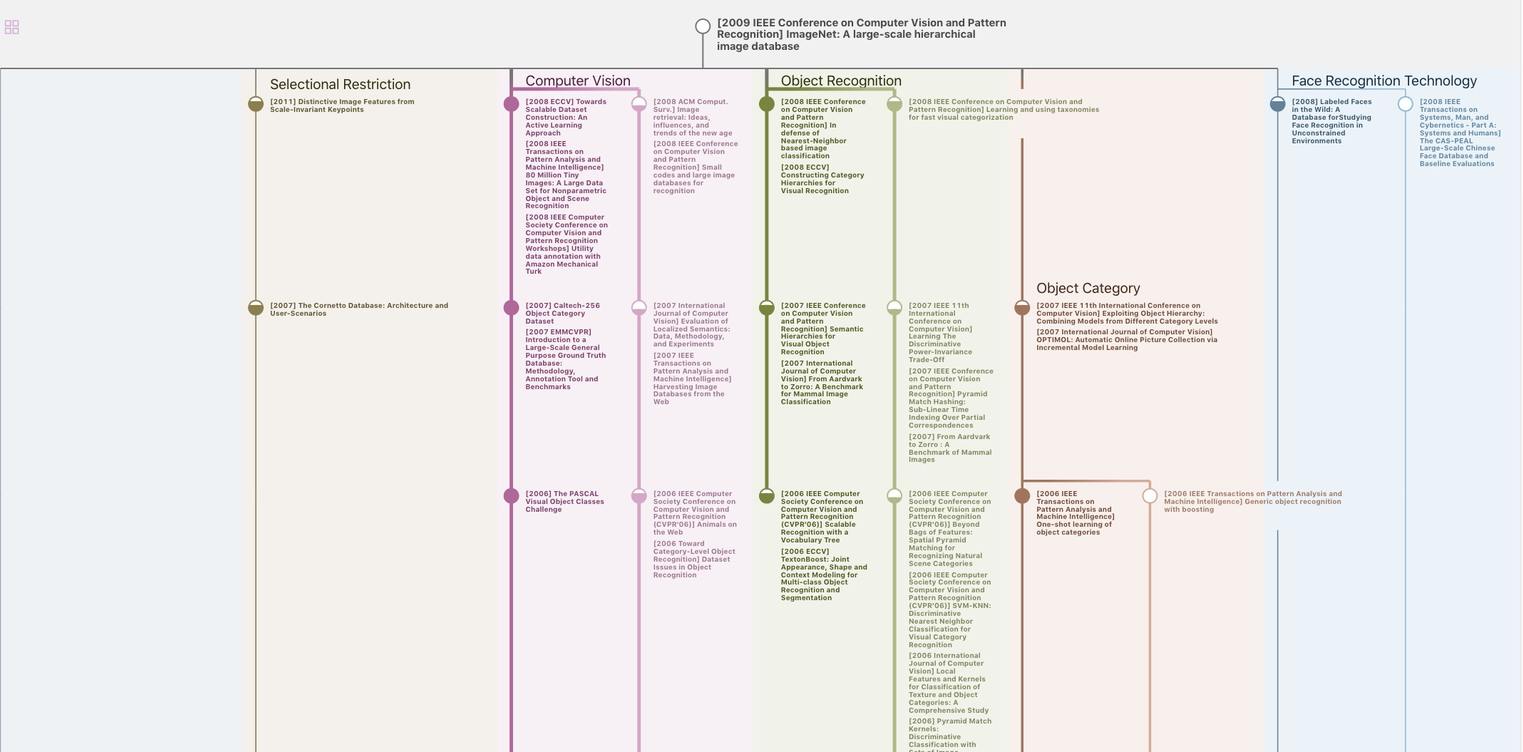
生成溯源树,研究论文发展脉络
Chat Paper
正在生成论文摘要