Adapting Self-Supervised Vision Transformers by Probing Attention-Conditioned Masking Consistency
NeurIPS 2022(2022)
摘要
Visual domain adaptation (DA) seeks to transfer trained models to unseen, unlabeled domains across distribution shift, but approaches typically focus on adapting convolutional neural network architectures initialized with supervised ImageNet representations. In this work, we shift focus to adapting modern architectures for object recognition -- the increasingly popular Vision Transformer (ViT) -- and modern pretraining based on self-supervised learning (SSL). Inspired by the design of recent SSL approaches based on learning from partial image inputs generated via masking or cropping -- either by learning to predict the missing pixels, or learning representational invariances to such augmentations -- we propose PACMAC, a simple two-stage adaptation algorithm for self-supervised ViTs. PACMAC first performs in-domain SSL on pooled source and target data to learn task-discriminative features, and then probes the model's predictive consistency across a set of partial target inputs generated via a novel attention-conditioned masking strategy, to identify reliable candidates for self-training. Our simple approach leads to consistent performance gains over competing methods that use ViTs and self-supervised initializations on standard object recognition benchmarks. Code available at https://github.com/virajprabhu/PACMAC
更多查看译文
关键词
domain adaptation,self-supervised learning,vision transformer,object recognition
AI 理解论文
溯源树
样例
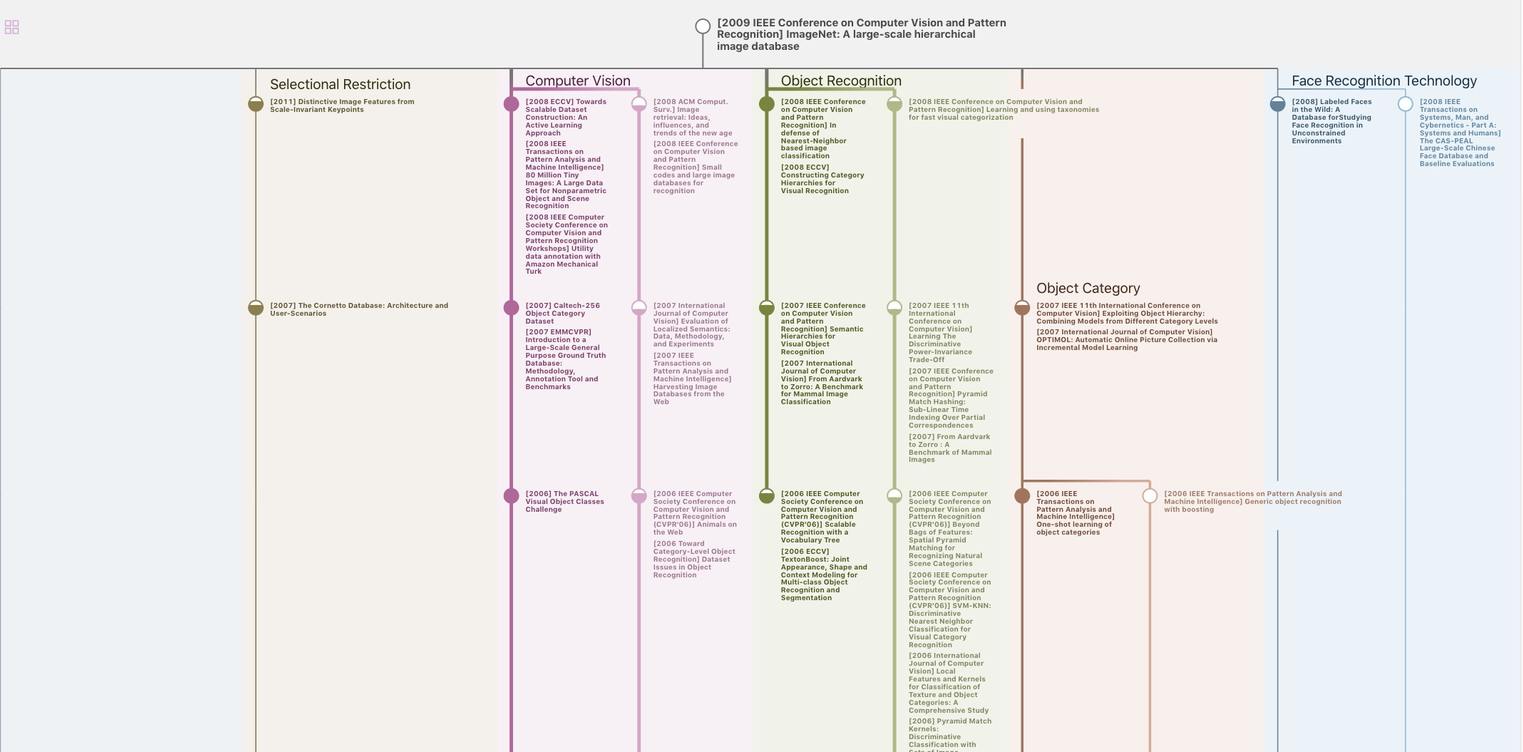
生成溯源树,研究论文发展脉络
Chat Paper
正在生成论文摘要