Noisy Learning for Neural ODEs Acts as a Robustness Locus Widening
arxiv(2022)
摘要
We investigate the problems and challenges of evaluating the robustness of Differential Equation-based (DE) networks against synthetic distribution shifts. We propose a novel and simple accuracy metric which can be used to evaluate intrinsic robustness and to validate dataset corruption simulators. We also propose methodology recommendations, destined for evaluating the many faces of neural DEs' robustness and for comparing them with their discrete counterparts rigorously. We then use this criteria to evaluate a cheap data augmentation technique as a reliable way for demonstrating the natural robustness of neural ODEs against simulated image corruptions across multiple datasets.
更多查看译文
关键词
neural odes,neural odes acts,robustness,learning
AI 理解论文
溯源树
样例
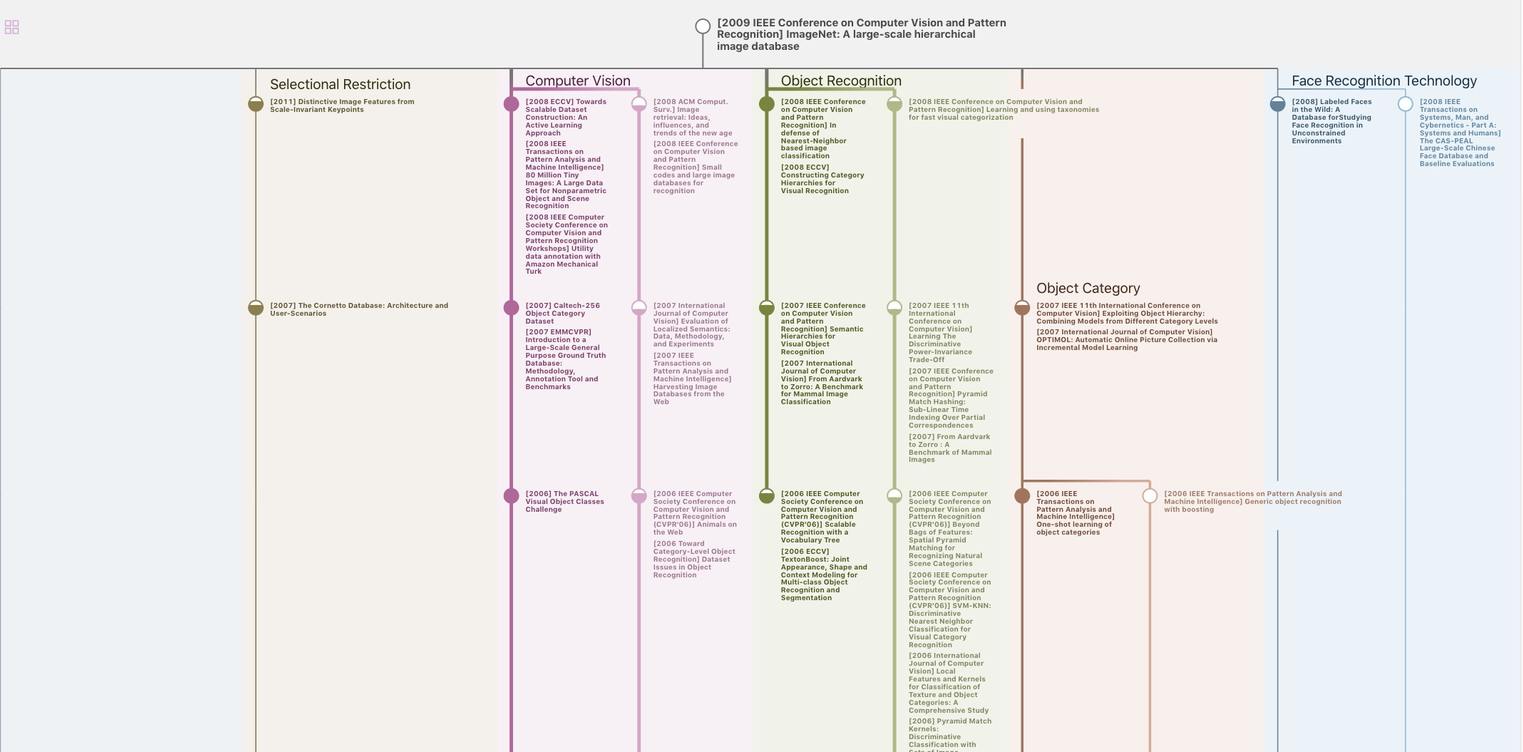
生成溯源树,研究论文发展脉络
Chat Paper
正在生成论文摘要