Towards Understanding How Machines Can Learn Causal Overhypotheses
ICLR 2023(2022)
摘要
Recent work in machine learning and cognitive science has suggested that understanding causal information is essential to the development of intelligence. The extensive literature in cognitive science using the ``blicket detector'' environment shows that children are adept at many kinds of causal inference and learning. We propose to adapt that environment for machine learning agents. One of the key challenges for current machine learning algorithms is modeling and understanding causal overhypotheses: transferable abstract hypotheses about sets of causal relationships. In contrast, even young children spontaneously learn and use causal overhypotheses. In this work, we present a new benchmark -- a flexible environment which allows for the evaluation of existing techniques under variable causal overhypotheses -- and demonstrate that many existing state-of-the-art methods have trouble generalizing in this environment. The code and resources for this benchmark are available at https://github.com/CannyLab/casual_overhypotheses.
更多查看译文
关键词
causal reasoning,intervention,causal overhypotheses,Reinforcement learning,gpt-3
AI 理解论文
溯源树
样例
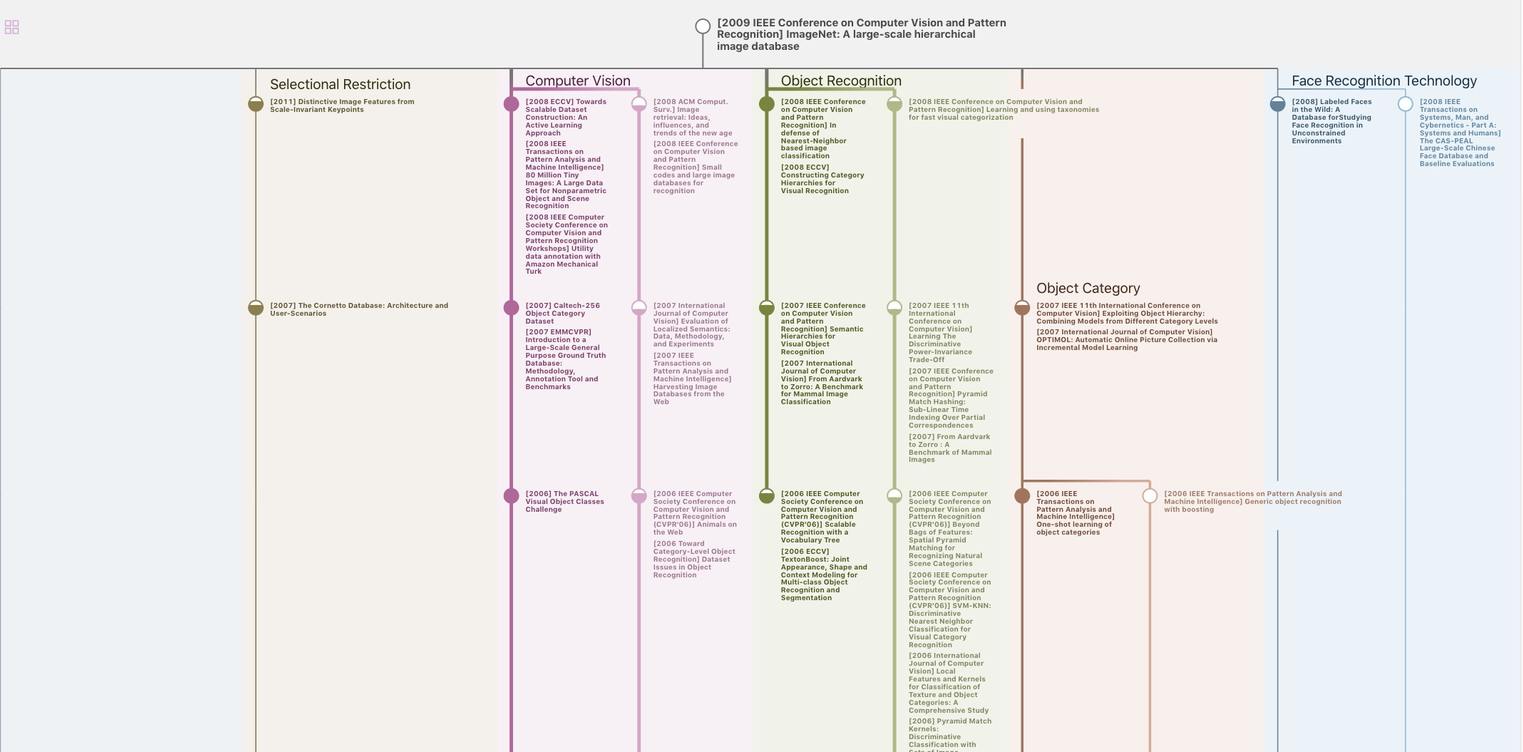
生成溯源树,研究论文发展脉络
Chat Paper
正在生成论文摘要