Computerized Classification Method for 1p/19q Codeletion in Low-Grade Glioma on Brain MRI Using Multi-Scale 3D-CNNs with Attention Mechanism
IEEJ Transactions on Electronics, Information and Systems(2022)
摘要
The purpose of this study was to develop a computerized classification method for low-grade gliomas (LGGs) with/without 1p/19q codeletion on brain MRI using multi-scale 3D-convolutional neural networks (Multi-scale 3D-CNNs) with an attention mechanism that analyzes only the tumor region. Our database consisted of brain T2-weighted MRI images for 159 patients (102 LGGs with 1p/19q codeletion and 57 LGGs without it) from The Cancer Imaging Archive. The proposed method was constructed from a feature extractor, an attention mechanism, and a perception branch. In the feature extractor, the feature maps were extracted from input images. The attention mechanism generated the attention maps focusing on a tumor region from those feature maps. The feature maps on the tumor region were then obtained using an attention pooling with the attention maps. In the perception branch, the likelihood of LGG with 1p/19q codeletion was evaluated based on the feature maps of the tumor region. The classification accuracy, the sensitivity, the specificity, and the area under the receiver operating characteristic curve for the proposed method were 78.0%, 82.4%, 70.2%, and 0.838, showing a significant improvement when compared with the multi-scale 3D-CNNs without the attention mechanism (69.8%, 75.5%, 59.6%, and 0.717; p = 0.001).
更多查看译文
关键词
brain mri,attention mechanism,low-grade,multi-scale,d-cnns
AI 理解论文
溯源树
样例
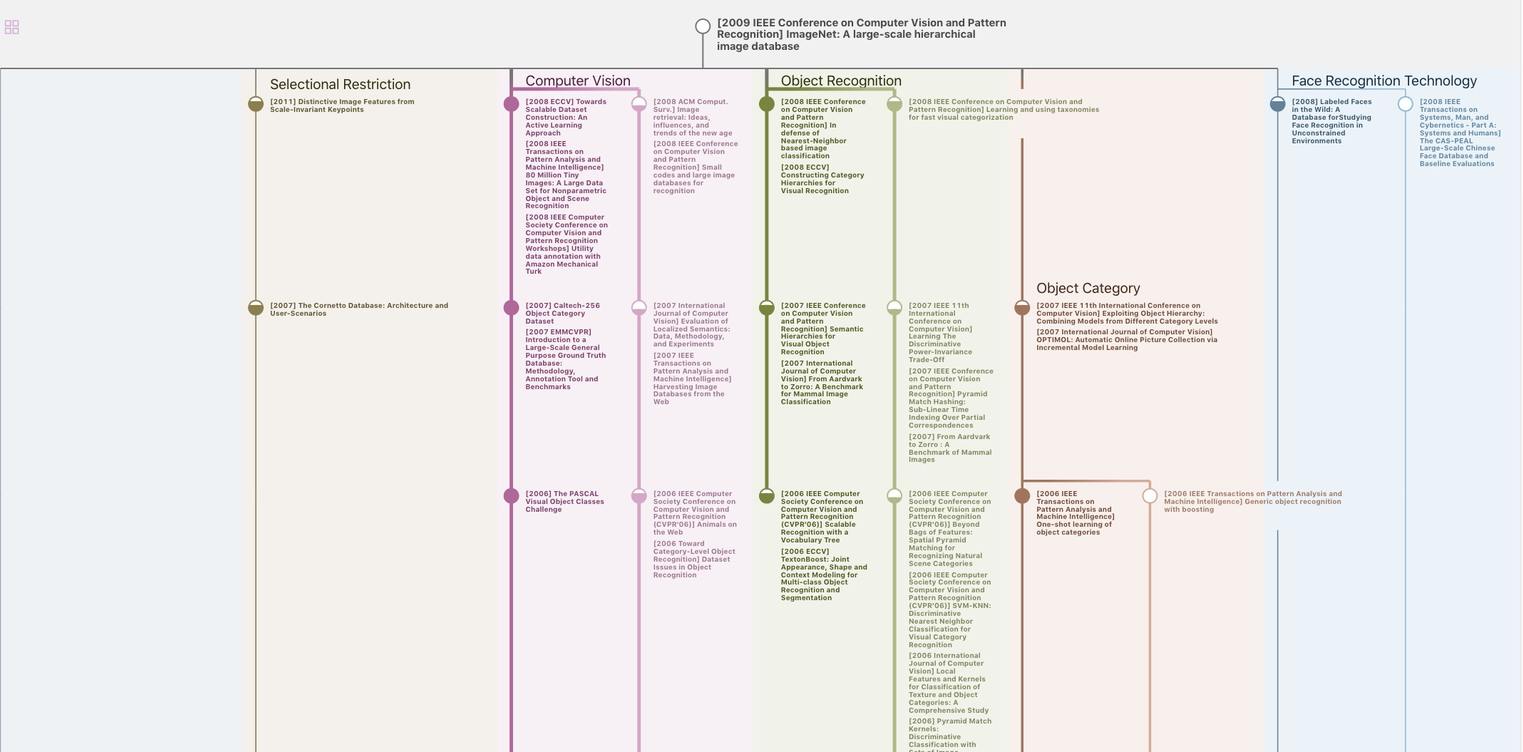
生成溯源树,研究论文发展脉络
Chat Paper
正在生成论文摘要