Fast computation of cluster validity measures for bregman divergences and benefits
SSRN Electronic Journal(2023)
摘要
Partitional clustering is one of the most relevant unsupervised learning and pattern recognition tech-niques. Unfortunately, one of the main drawbacks of these methodologies refer to the fact that the num-ber of clusters is generally assumed to be known beforehand and automating its selection is not straight-forward. On the same token, internal validity measures, such as the Silhouette index, Davies-Bouldin and Caliski-Harabasz measures have emerged as the standard techniques to be used when comparing the goodness of clustering results obtained via different clustering methods. These measures take into con-sideration both the inter and intra-cluster simmilarities and can be adapted to different metrics. Unfortu-nately, their used has been hindered due to their large computational complexities, which are commonly quadratic with respect to the number of instances of the data set. In this work, we show that the time complexity of computing the most popular internal validity measures can be utterly reduced by making used of the within-cluster errors and different properties of the Bregman divergences. This contribution ultimately allows us to massively speed-up the selection of an adequate number of clusters for a given data set as verified with extensive empirical comparisons.(c) 2023 Published by Elsevier B.V.
更多查看译文
关键词
Partitional clustering,Number of clusters,Silhouette index,Davies-Bouldin,Calinski-Harabasz,Bregman divergences
AI 理解论文
溯源树
样例
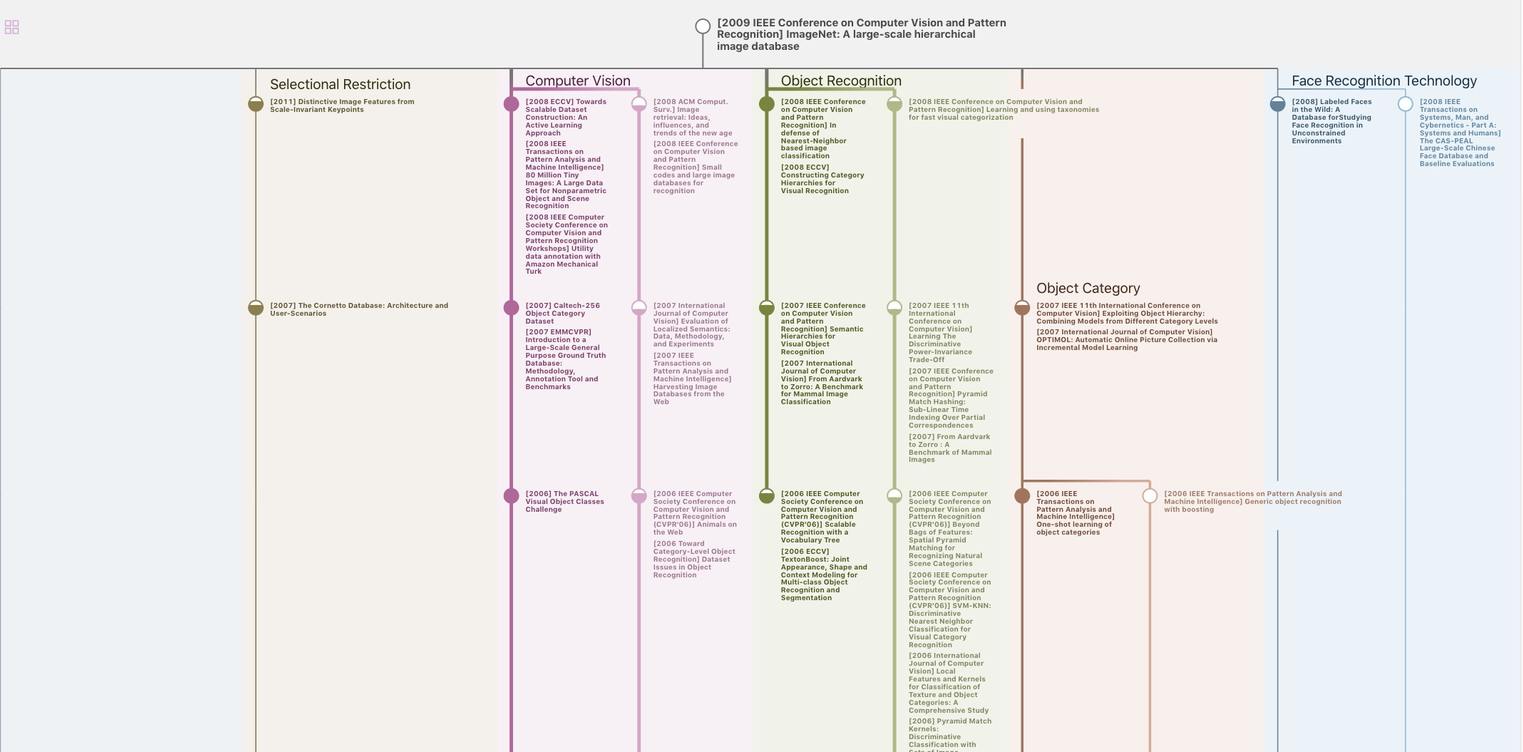
生成溯源树,研究论文发展脉络
Chat Paper
正在生成论文摘要