Quality-Driven Online Task-Bundling-Based Incentive Mechanism for Mobile Crowdsensing
IEEE Transactions on Vehicular Technology(2022)
摘要
With the advancement of wireless communication technologies and rich embedded sensors in smart mobile devices, mobile crowdsensing has become an attractive paradigm for collecting sensing data from surrounding environments. Effective incentive mechanisms often play an important role in guaranteeing high quality of services and wide involvement of workers. Much work has been carried out in this aspect to encourage workers to strenuously and truthfully provide high quality data. However, task quality does not only depend on workers’ subjective effort or truthfulness, but also workers’ distribution and task quality growth law. In this paper, we consider a scenario where worker arrivals are unevenly distributed and the growth of task quality conforms to the law of diminishing margin of workers’ efforts. We propose a Quality-driven Online Task-Bundling-based incentive mechanism (QOTB). The design objective is to maximize the social welfare while maximally satisfying the task quality requirements. In QOTB, we introduce Mental accounting Theory to build accounts for task execution profit and bonus, respectively, which are then used to derive the participation willingness of workers. We adopt task bundling to stimulate workers to change their original travel schedules for balancing the task participations according to the popularities of task locations and also the traveling cost. We present the detailed mechanism design of QOTB. We prove that the QOTB mechanism has desired properties of willingness truthfulness, individual rationality, and computation efficiency. We conduct extensive simulations and the results show that QOTB can effectively improve the social welfare while satisfying the task quality requirements.
更多查看译文
关键词
Data quality,incentive mechanism,mobile crowdsensing,mobile wireless networks,route planning,task bundling
AI 理解论文
溯源树
样例
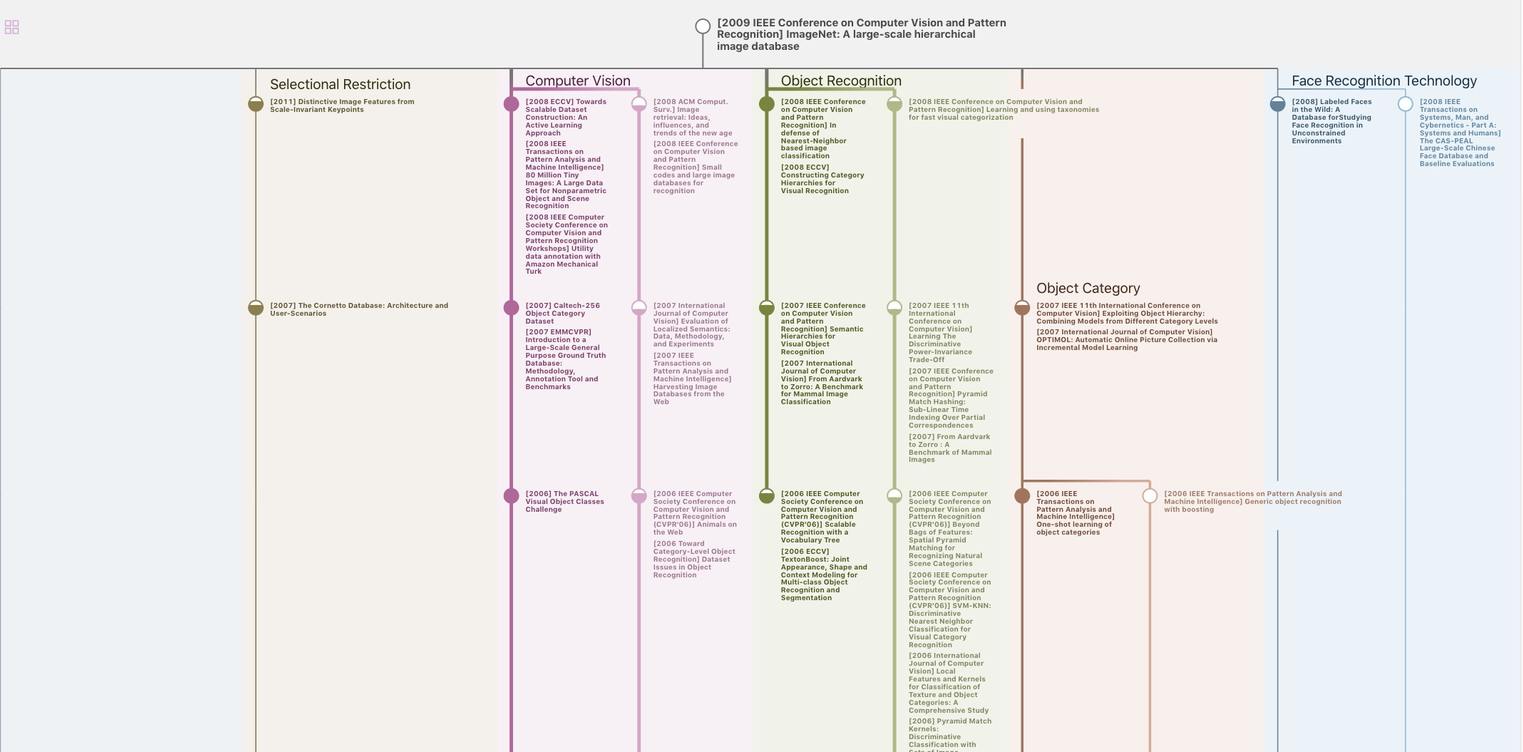
生成溯源树,研究论文发展脉络
Chat Paper
正在生成论文摘要