Reinforced-LSTM Trajectory Prediction-Driven Dynamic Service Migration: A Case Study
IEEE Transactions on Network Science and Engineering(2022)
摘要
Predicting user behavior is the cornerstone of intelligent services and applications for providing and optimizing services over mobile networks. In modern edge computing scenarios, contents and services will be ordered close to end-users and will be highly sensitive to user mobility. Deep Learning models have had significant success in performing prediction tasks. However, providing reliable predictions for real-world networks in scale requires the Neural Architecture Search to be optimized on a user basis. In this work, we present an LSTM-based mobility predictor to improve the trajectory prediction accuracy. To speed up the model convergence rate, we employ a reinforcement learning technique to automate the selection procedure of the best neural network architecture. To further accelerate the reinforcement learning environmental search procedure, we transfer the architecture knowledge learned from a teacher LSTM to a student LSTM via a transfer learning framework. Furthermore, we showcase the possible improvements of edge-computing enabled networks, in the form of a predictive handover algorithm that applies the prediction information to reduce the handover-failure rate, as well as handover-triggered service migration in edge computing layer of the network. Experiment results prove the efficiency of the proposal, its impacts on improving ping-pong handover, and the service migration.
更多查看译文
关键词
Service migration,handover optimization,trajectory prediction,recurrent neural network,reinforcement learning,transfer learning
AI 理解论文
溯源树
样例
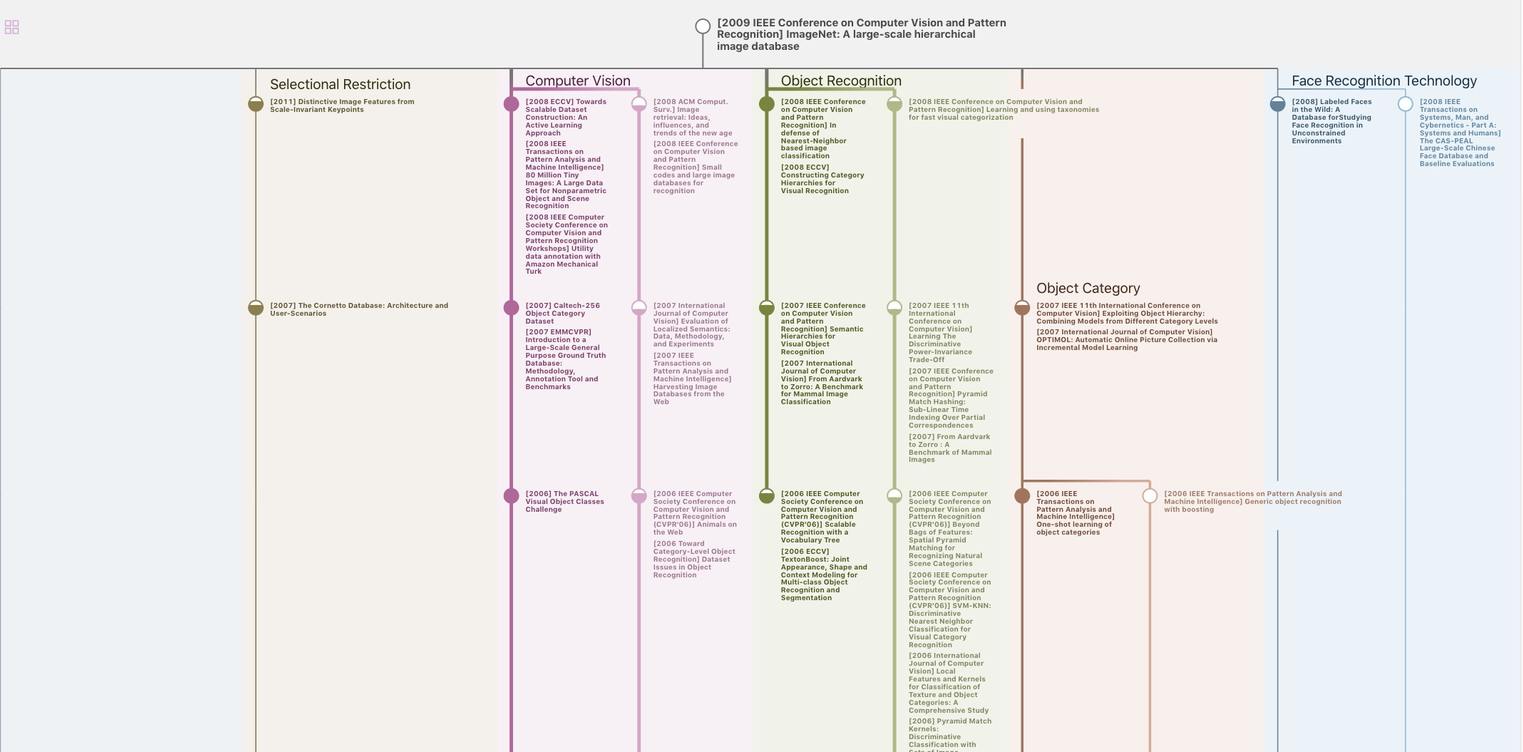
生成溯源树,研究论文发展脉络
Chat Paper
正在生成论文摘要