Deep Learning-based Transfer Learning Model in Diagnosis of Diseases with Brain Magnetic Resonance Imaging
Acta Polytechnica Hungarica(2022)
摘要
Computer-aided diagnosis (CAD) is an effective resource for diagnosing brain disorders rapidly and is also used for reducing human diagnostic errors to enhance and extend the quality of patient life. The deep learning model can self learn and generalize over a huge volume of data, it has recently gained a lot of interest over the research community in classifying medical images. But deep learning model created from the scratch takes more training time as well as a huge amount of data. Using pre-trained networks for a new, similar problem is the fundamental idea of transfer learning. In this work, the survey on disease diagnosis using deep learning-based transfer learning with Brain MRI images alone is carried out over the last 5 years. The inference drawn from this work is that a hybrid architecture based on transfer learning produced more than 90% accuracy in most of the cases with minimal training time. In hybrid architecture, more than one pre-trained models are integrated to extract high-level features. Pre-trained models are good at recognising high-level features like edges, patterns, and so on. The model designed with pre-trained model starts with learned weights rather than assigning a random value. This promotes faster convergence and, as a result, reduces the amount of time required to train the model.
更多查看译文
关键词
Deep learning, Brain MRI, Convolution Neural Network, Deep neural network, Transfer Learning
AI 理解论文
溯源树
样例
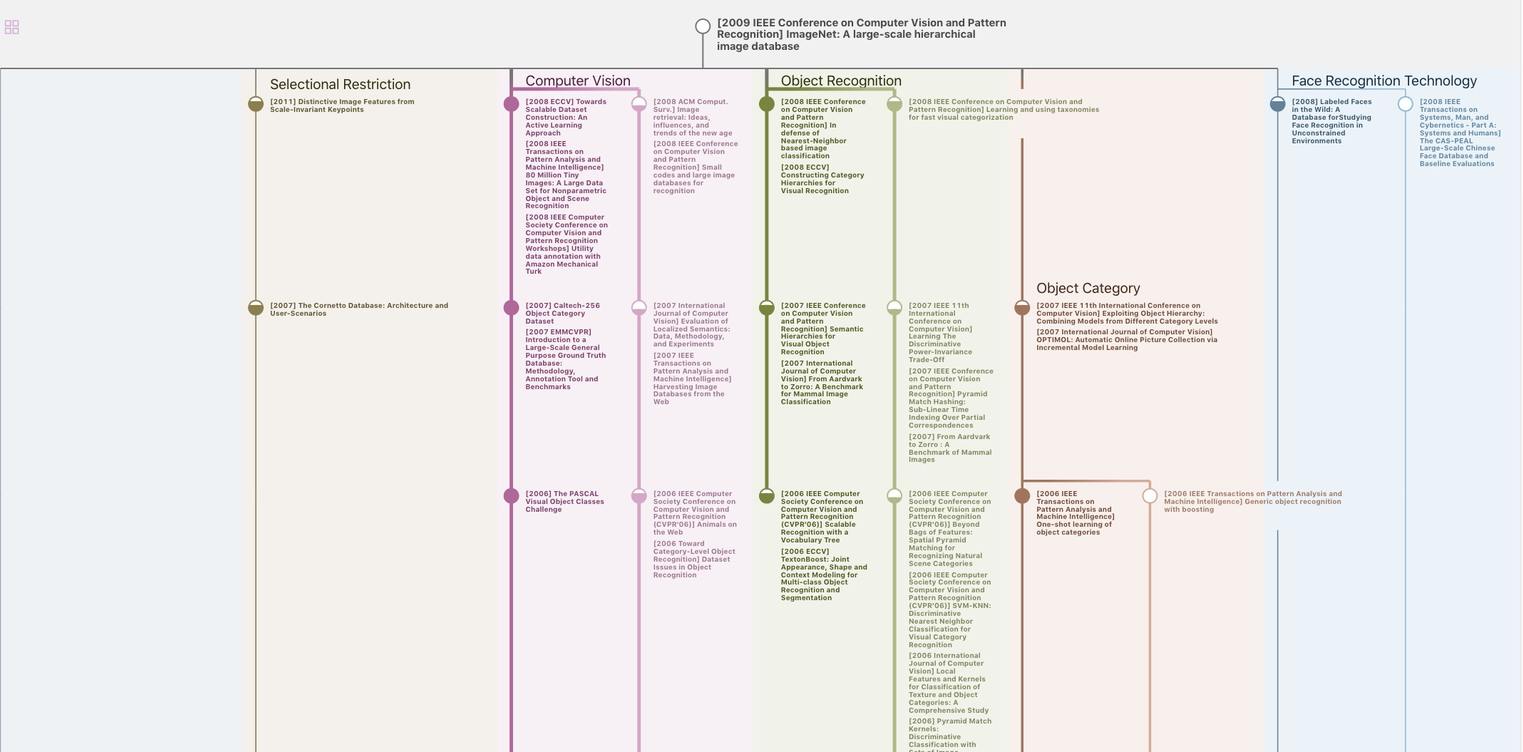
生成溯源树,研究论文发展脉络
Chat Paper
正在生成论文摘要