Revisiting Negative Sampling VS. Non-Sampling in Implicit Recommendation
ACM Transactions on Information Systems(2022)
摘要
Recommendation systems play a vital role in alleviating information overload. Generally, a recommendation model is trained to discern between positive (liked) and negative (disliked) instances for each user. However, under the open-world assumption, there are only positive instances but no negative instances from users’ implicit feedback, which poses the imbalanced learning challenge of lacking negative samples. To address this, two types of training strategies have been proposed in previous studies, which are 1) negative sampling strategy and 2) non-sampling strategy. The first strategy samples negative instances from missing data (i.e., unlabeled data), while the second one regards all the missing data as negative. Although training strategies are known to be essential for algorithm performance, the in-depth comparison of negative sampling and non-sampling is still left insufficiently explored by far. To bridge this gap, in this paper we systematically analyze the role of negative sampling and non-sampling for implicit recommendation. Specifically, we first theoretically revisit the objection of negative sampling and non-sampling. Then, with a careful setup of various representative recommendation methods, we explore the performance of negative sampling and non-sampling in different scenarios. Our results empirically show that although negative sampling has been widely applied to recent recommendation models, it is non-trivial for uniform sampling methods to show comparable performance to non-sampling learning methods. Finally, we discuss the scalability and complexity of negative sampling and non-sampling, and present some open problems and future research topics that worth to be further explored.
更多查看译文
关键词
Recommender systems,non-sampling,negative sampling,implicit feedback
AI 理解论文
溯源树
样例
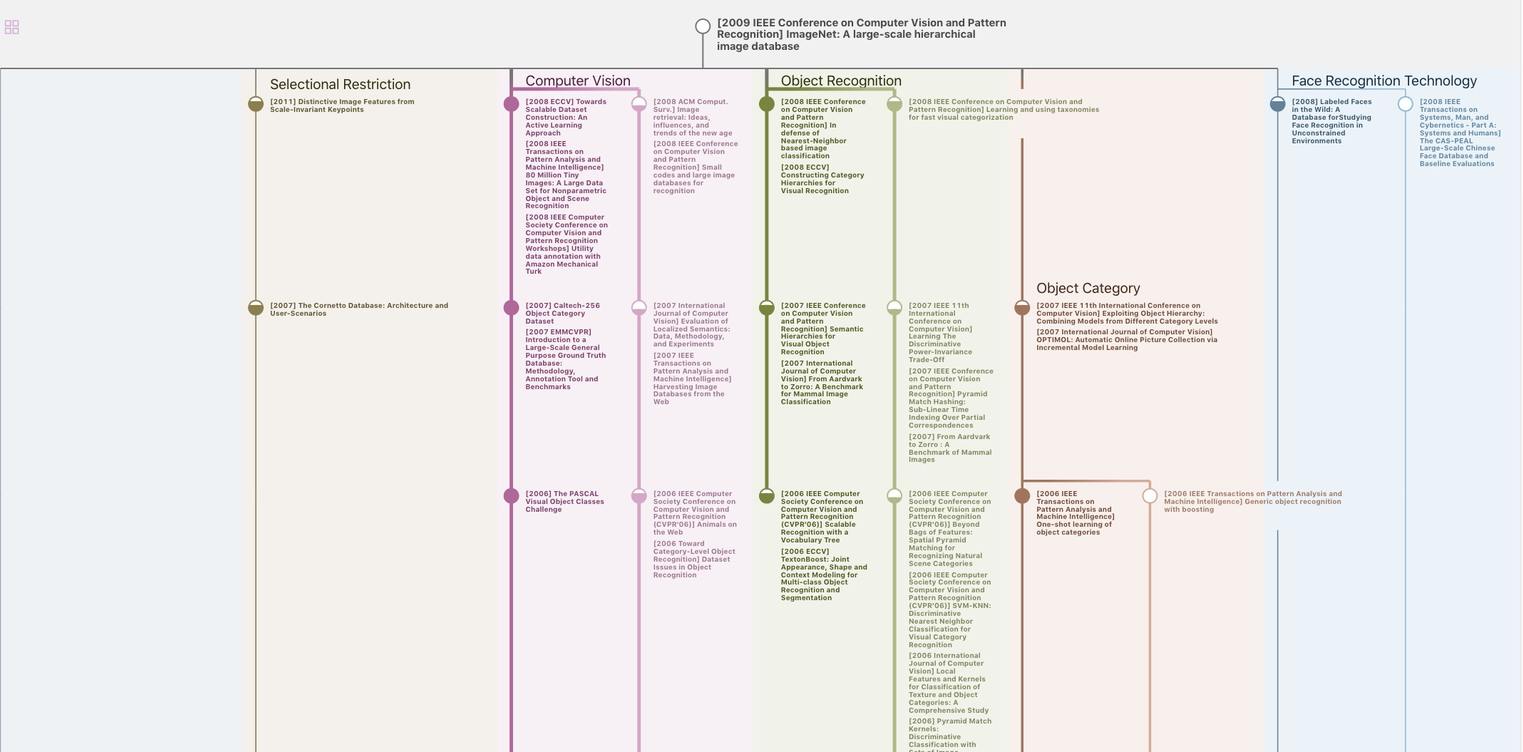
生成溯源树,研究论文发展脉络
Chat Paper
正在生成论文摘要