Building subsurface models with horizon-guided interpolation and deep learning: Application to the Volve field data
GEOPHYSICS(2022)
摘要
Subsurface modeling plays an important role in hydrocarbon exploration but remains a challenging task that typically re-quires a full and reasonable integration of geophysical observa-tions and geologic constraints. We have developed a workflow to fully use seismic amplitudes, well-log properties, and inter-preted seismic structures to build geologically reasonable mod-els. We take the Volve field data as an example and apply our workflow step by step as follows. First, we perform some pre-processing on the provided Volve seismic data, horizons, and well logs to remove anomalous values and adjust seismic-well ties in the depth domain. Second, we use a dynamic-program-ming-based method to infill gaps and refine the vertical posi-tions of the provided horizons and efficiently pick more horizons. We further use the horizon surfaces to interpolate a relative geologic time (RGT) volume which can be considered as an implicit structural model representing seismic structural and stratigraphic features. Third, we integrate the provided well logs and the computed RGT volume to interpolate a subsurface model that conforms to well-log properties and seismic struc-tural and stratigraphic features. Finally, we propose a multiscale neural network to predict a final subsurface model by using a combination of the seismic data and the interpolated model as inputs and using the well-log data as training labels. Inputting the interpolated model to the network helps to provide a low-frequency control for obtaining a more stable prediction. The results indicate that our workflow is able to produce geologi-cally reasonable subsurface models with high lateral continuity and vertical resolution.
更多查看译文
关键词
subsurface models,deep learning,interpolation,horizon-guided
AI 理解论文
溯源树
样例
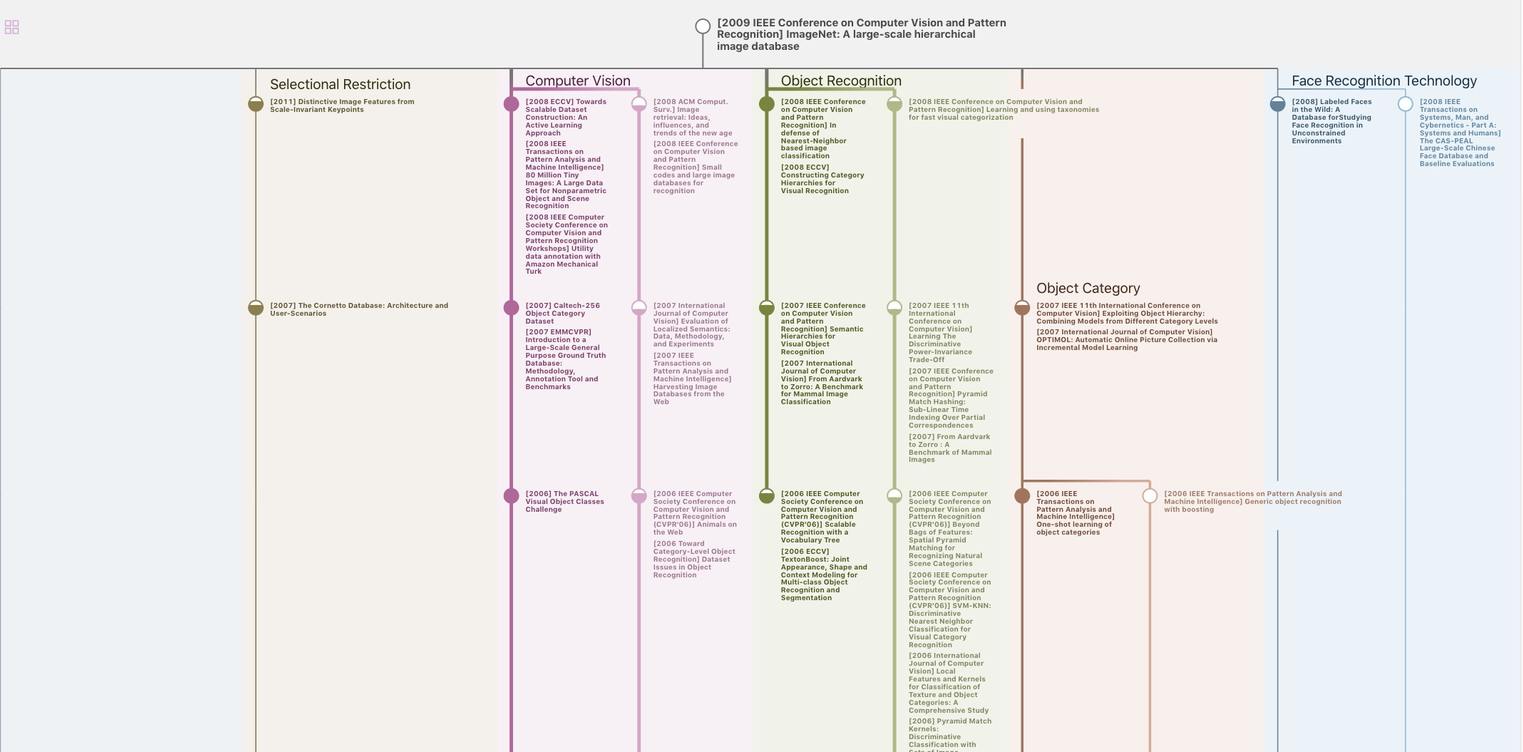
生成溯源树,研究论文发展脉络
Chat Paper
正在生成论文摘要