Fine-Grained Classification of Colposcopic Images with Multi-Modality
2021 8th International Conference on Biomedical and Bioinformatics Engineering(2021)
摘要
Computer aided diagnosis (CAD) has gained popularity in medical image processing over the last decade. As with image based manual diagnosis, CAD system should base its results on specific lesions. In the case of colposcopic images, two modalities are collected for diagnosis, namely Lugol’s Iodine (LI) and Acetic Acid (A). In practice, classification performance may suffer from barely distinguishable lesions in mono-modality. To tackle this problem, we present in this paper a framework with fine-grained strategy and further boost its performance with multi-modality. Specifically, our proposed framework consists of two branches, Local Lesion Branch and Global Branch, to implement a fine-grained strategy. Local Lesion Branch indicates existence and severity of lesion in different modalities on patch level. Global Branch extracts features on whole image level, and uses our proposed Cross Stage Modality Fusion (CSMF) Blocks to enable feature exchange and fusion between two modalities. Features from two branches are then concatenated for final prediction. We conduct experiments on clinical dataset of colposcopic images. The result shows that our method achieves an accuracy of 83.39%, outperforming mono-modal baseline model by over 7.24%.
更多查看译文
AI 理解论文
溯源树
样例
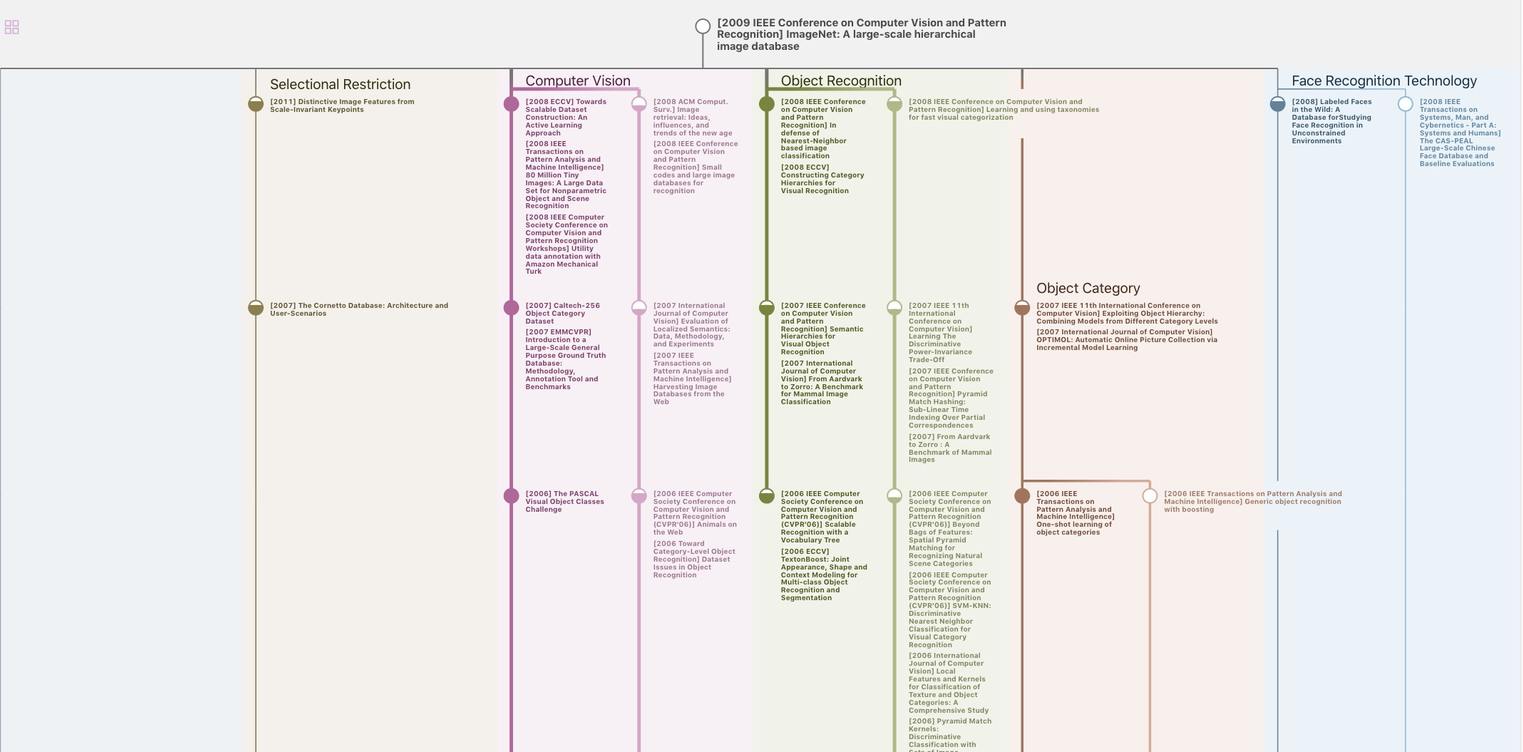
生成溯源树,研究论文发展脉络
Chat Paper
正在生成论文摘要