Spatial data analytics for optimum data declustering in shale systems
Unconventional Shale Gas Development(2022)
摘要
Virtually all sparsely sampled, spatial data sets are sampled in a biased manner. Preferential sampling can result in biased statistics, for example, high mean porosity, resulting in overestimation of the oil in place. Cell-based declustering (CBD) for data weighting is commonly applied to mitigate bias due to spatial sample clustering, preferential sampling over regions with low or high feature value trends. CBD results are sensitive to cell-size selection and the current practice for choice of cell size is irrational, subjective and overly conservative. Hence, it is crucial to adopt a new workflow utilizing spatial data analytics, with spatial statistics to predict cell size rationally and objectively. The proposed SSADC workflow uses nearest neighbors and Ripley’s K to characterize the multiscale intersample distance, and based on a proposed criteria, a rational choice for cell size. The proposed spatial data analytics approach for cell size selection results in improved performance for sparsely sampled, spatial data declustering.
更多查看译文
关键词
shale systems,spatial data analytics,optimum data
AI 理解论文
溯源树
样例
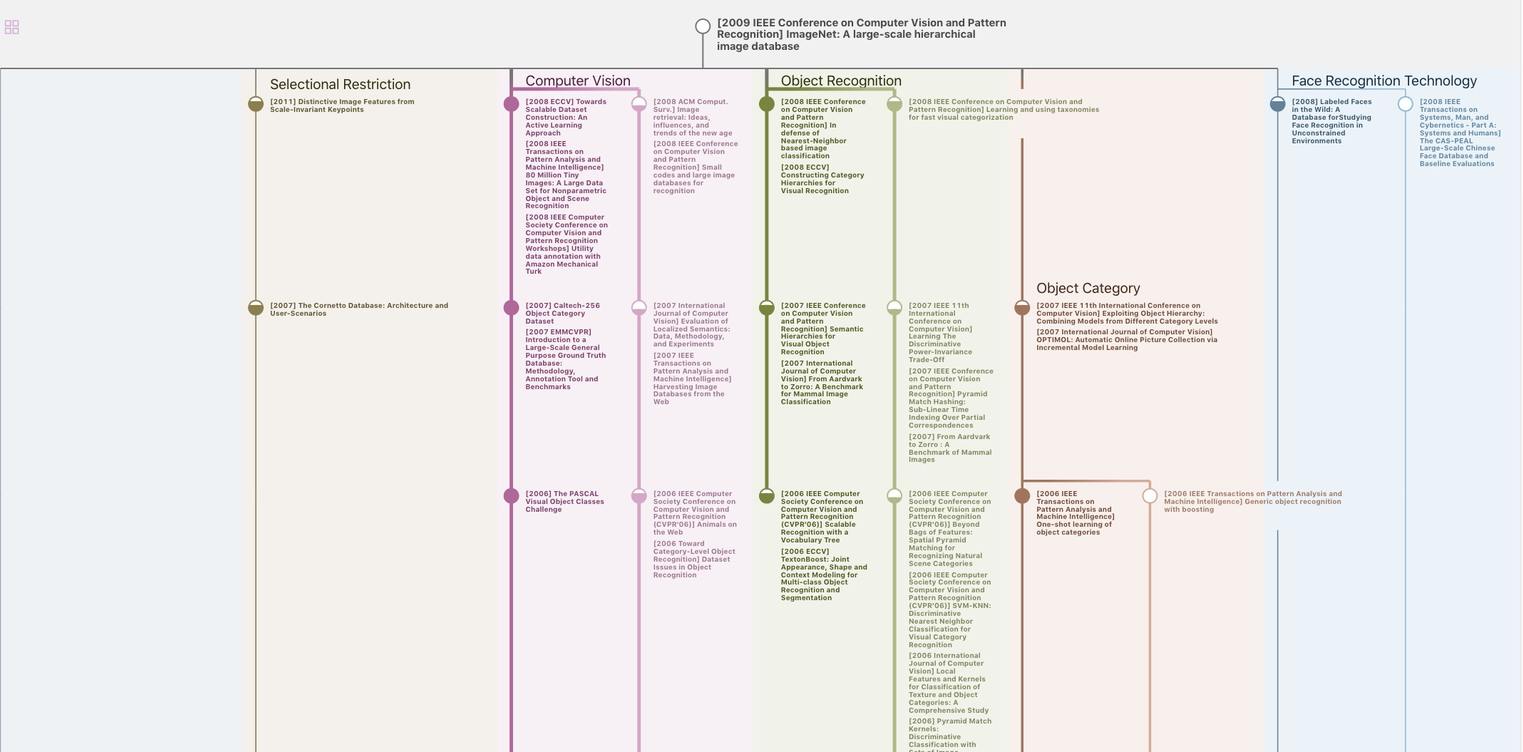
生成溯源树,研究论文发展脉络
Chat Paper
正在生成论文摘要