Probabilistic anomaly detection methods using learned models from time-series data for multimedia self-aware systems
Advanced Methods and Deep Learning in Computer Vision(2022)
摘要
Anomaly detection techniques constitute a fundamental resource in many applications such as medical image analysis, fraud detection or video surveillance. These techniques represent an essential step also for artificial self-aware systems that can continually learn from new situations. In this chapter, we present a semisupervised method for the detection of anomalies for this type of self-aware agents. The described method leverages the message-passing capability of Generalized Dynamic Bayesian Networks (GDBNs) to provide anomalies at different abstraction levels for diverse types of time-series data (i.e., both low-dimensional and high-dimensional). The detected anomalies could consequently be employed to enable the system to evolve by integrating the new acquired knowledge. To present a case study for the description of the anomaly detection method, we propose to use multisensory data from a semiautonomous vehicle performing different tasks in a closed environment.
更多查看译文
关键词
probabilistic anomaly detection methods,multimedia,time-series,self-aware
AI 理解论文
溯源树
样例
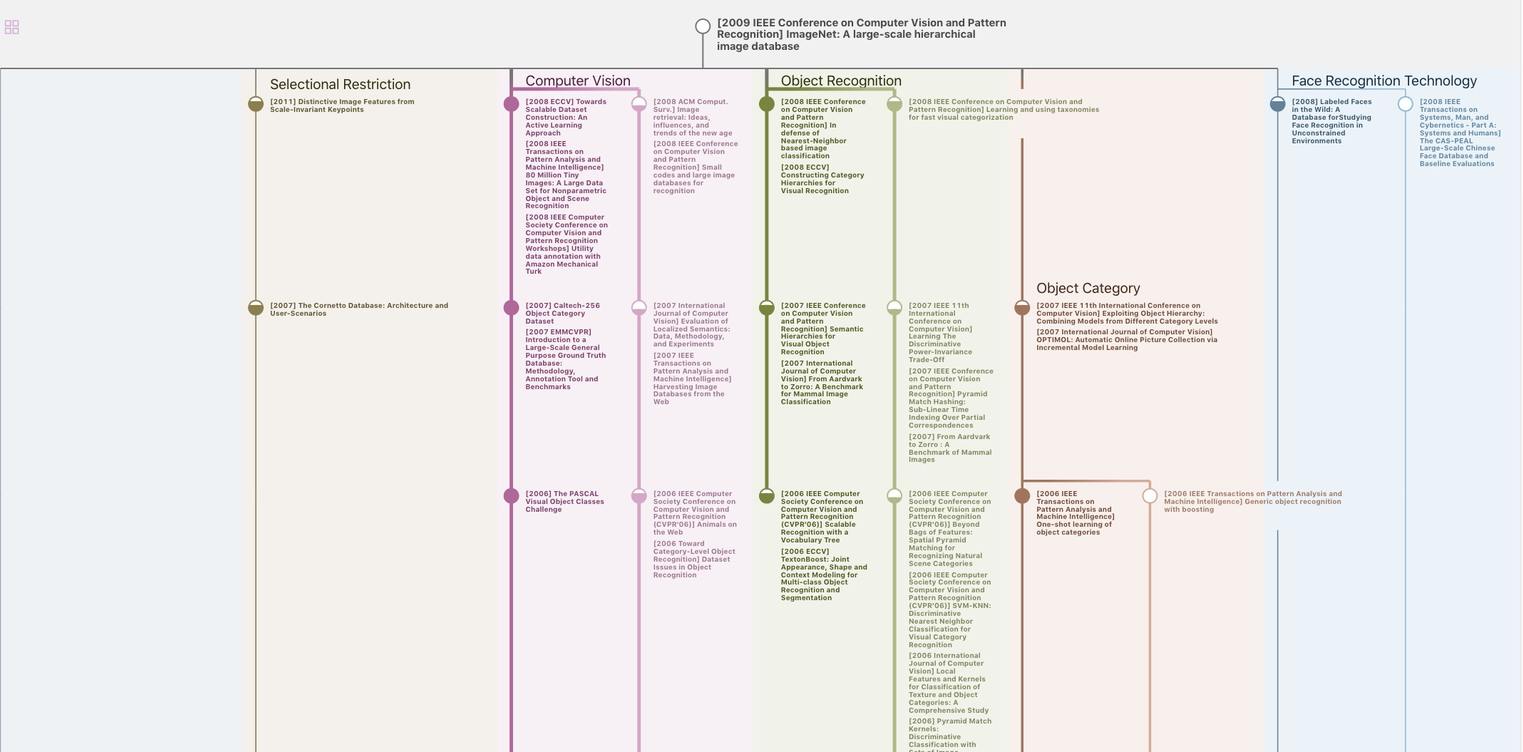
生成溯源树,研究论文发展脉络
Chat Paper
正在生成论文摘要