Does local pruning offer task-specific models to learn effectively?
Proceedings of the Student Research Workshop Associated with RANLP 2021(2021)
摘要
The need to deploy large-scale pre-trained models on edge devices under limited computational resources has led to substantial research to compress these large models. However, less attention has been given to compress the task-specific models. In this work, we investigate the different methods of unstructured pruning on task-specific models for Aspect-based Sentiment Analysis (ABSA) tasks. Specifically, we analyze differences in the learning dynamics of pruned models by using the standard pruning techniques to achieve high-performing sparse networks. We develop a hypothesis to demonstrate the effectiveness of local pruning over global pruning considering a simple CNN model. Later, we utilize the hypothesis to demonstrate the efficacy of the pruned state-of-the-art model compared to the over-parameterized state-of-the-art model under two settings, the first considering the baselines for the same task used for generating the hypothesis, i.e., aspect extraction and the second considering a different task, i.e., sentiment analysis. We also provide discussion related to the generalization of the pruning hypothesis.
更多查看译文
关键词
models,learn,task-specific
AI 理解论文
溯源树
样例
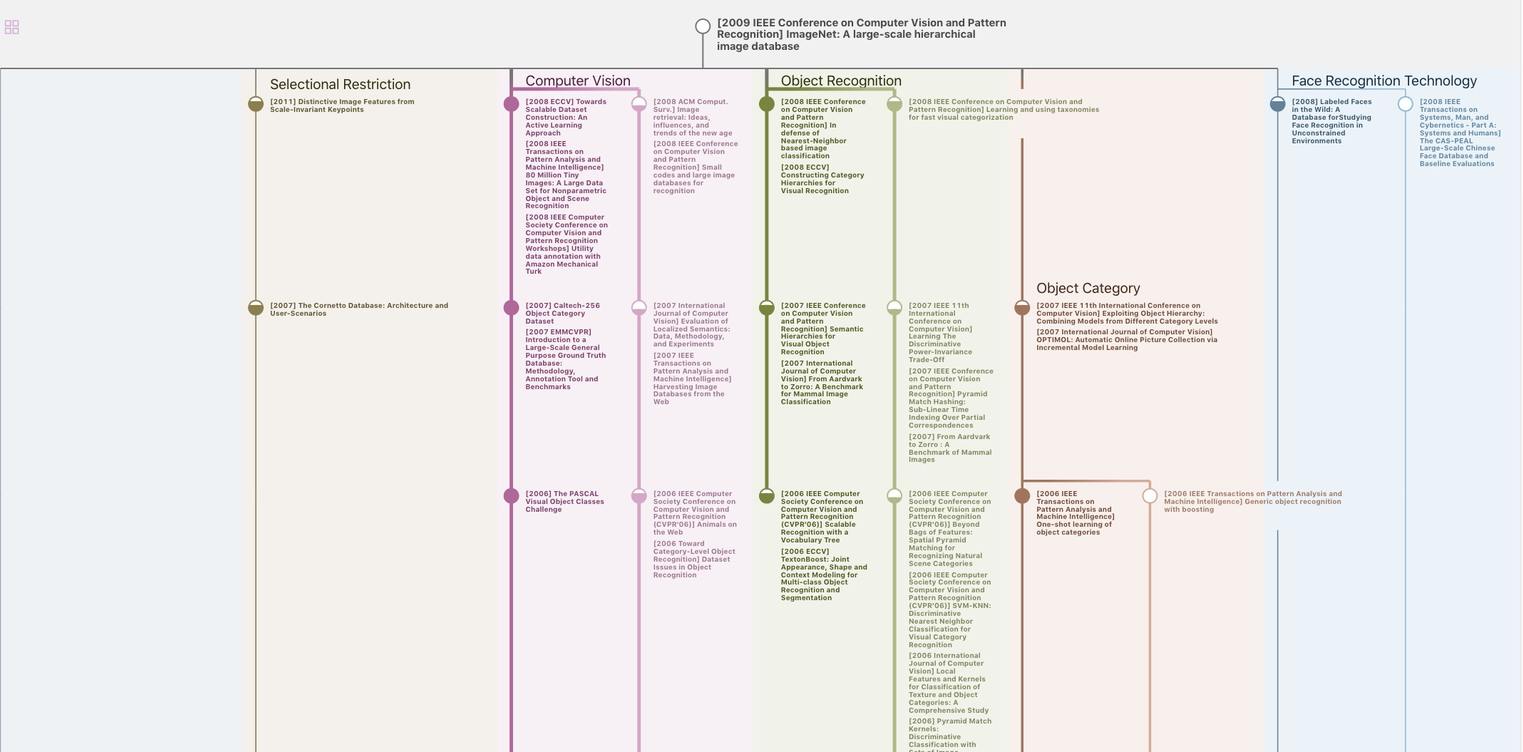
生成溯源树,研究论文发展脉络
Chat Paper
正在生成论文摘要