Impact of Self-Learning Based High-Frequency Traders on the Stock Market
SSRN Electronic Journal(2021)
摘要
In this paper we investigate the role of self-learning agents in multi-agent models of financial markets. We develop an agent-based simulation model of a financial market and, in addition to the agents with fixed strategies used in previous research, we introduce an agent with a self-learning strategy. To model the behavior of such an agent, we use deep reinforcement learning algorithms, namely deep deterministic policy gradient (DDPG). Next, we conduct a comparative analysis of the results of the constructed model with outcomes of previously proposed models, as well as with the characteristics of real market. To conduct comparative analysis, we use stylized facts of asset returns that allow us to evaluate and compare the characteristics of the markets. Our results show that a model with a self-learning agent gives a better approximation of the real market than a model with classic agents. In particular, unlike the model with classical agents, the model with a self-learning agent turns out to be not so heavy-tailed. Thus, we demonstrate that for a complete understanding of market processes simulation models should take into account self-learning agents that have a significant presence at modern financial markets.
更多查看译文
关键词
Agent-based model, Stylized facts, Reinforcement learning, Market simulations
AI 理解论文
溯源树
样例
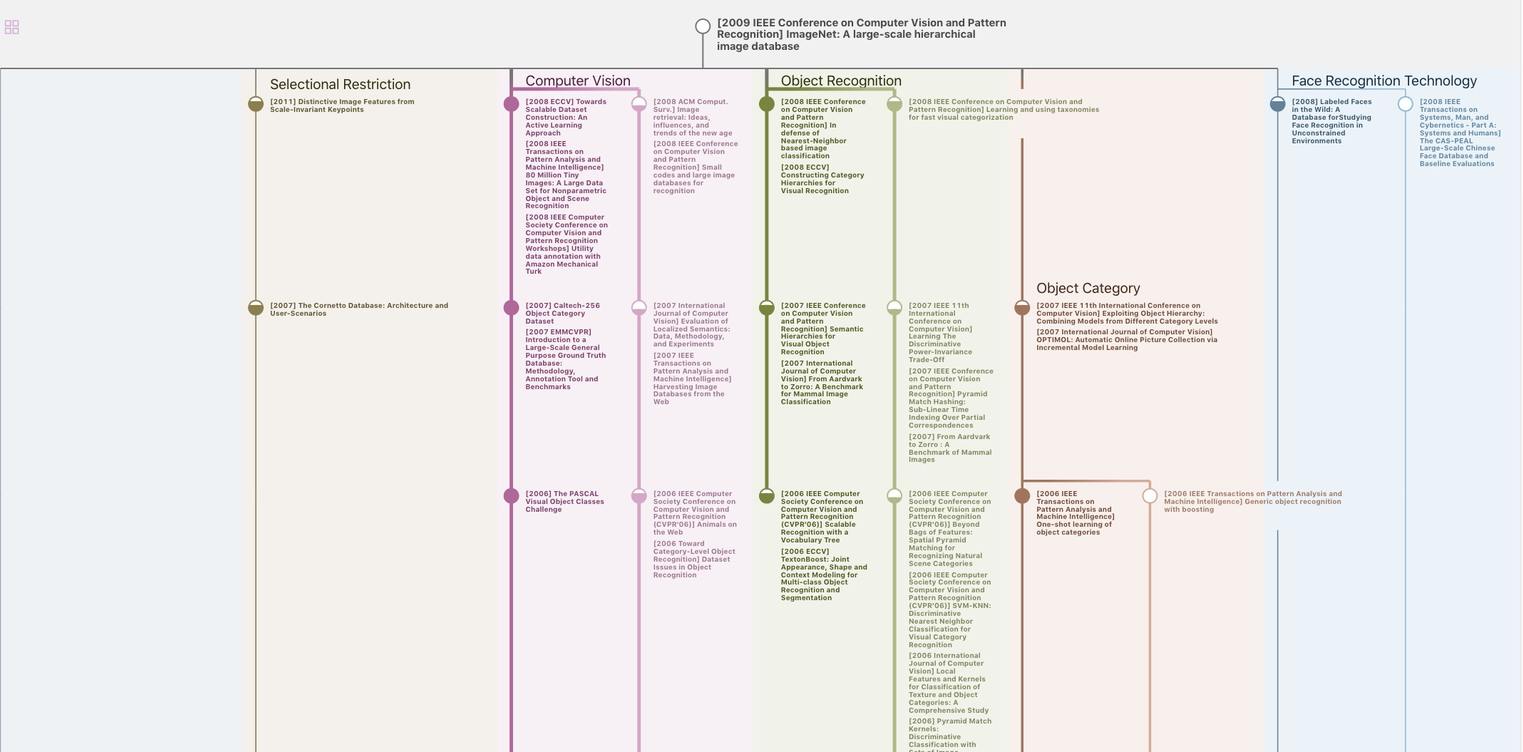
生成溯源树,研究论文发展脉络
Chat Paper
正在生成论文摘要