Physics-Based Feature Extraction from Bulk Time-Series PMU Datasets for Event Detection
2021 IEEE Power & Energy Society General Meeting (PESGM)(2021)
摘要
In this work, two physics-based feature extraction techniques are developed for bulk time-series phasor measurement unit (PMU) datasets collected from the field to train the machine learning model for anomaly detection. Two approaches have been developed to extract useful features for different types of events. An admittance-based feature extraction technique is developed to detect events that involve line outages and system topology variations. The developed algorithm extracts the system equivalent admittance variation. Additionally, Fielder's Theory is utilized to further reduce the potential computation burden by sectionalizing large-scale grids and datasets into smaller areas. Second, an oscillation-based feature extraction technique is developed to detect low-frequency oscillations in power grids. The dominant oscillation modes in the grids are extracted using energy-sorted Prony analysis. The extracted dominant oscillation modes by the developed work exhibit a high fitting resolution. Finally, the developed techniques have been validated using large-scale and real-world datasets.
更多查看译文
关键词
Event detection,feature extraction,machine learning,PMU,Prony analysis
AI 理解论文
溯源树
样例
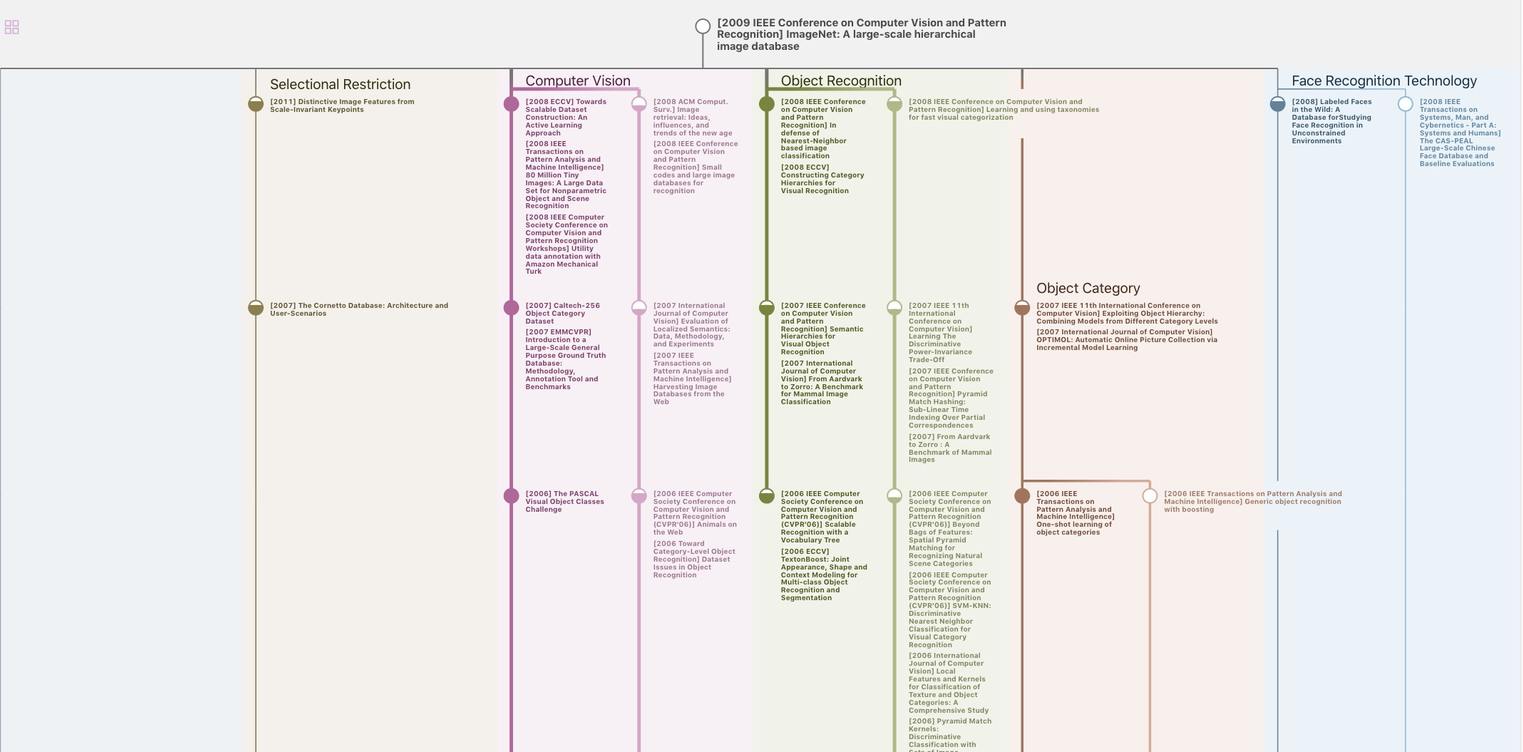
生成溯源树,研究论文发展脉络
Chat Paper
正在生成论文摘要