Comparison of Bagging and Boosting Ensemble Machine Learning Methods for Face Recognition
Procedia Computer Science(2021)
摘要
Since biometric features are unique to each person and deliver precise identification, they are preferred for applications where security is very important recently. Recognition and verification processes using biometric features are increasing day by day. The biometry of the face has very good for keeping information about the face the quality of low intrusiveness and advanced exactness. There are different biometric techniques as hand geometry, iris recognition, as well as fingerprint recognition, which are proposed for the same purpose. But face recognition is the most efficient and widely-employed biometric technique among them. This biometric method has been at the root of numerous innovations such as Cloud Computing, Social Networks and Big Data analysis over the past decades. An effective face recognition in big dataset is challenging and imperative tasks. In this paper, face recognition is done by using ensemble learning methods which are a part of the proposed intelligent face recognition system. In this paper, we presented different algorithms employing skin color for face detection, histogram tor the feature extraction and ensemble methods for recognition part. Hence, the developed Bagging and Boosting has thoroughly presented their superiority over FERET database. Advanced classification capabilities of the ensemble classifiers are having better accuracy than the existing methods in the face recognition. Experimental results employing a FERET benchmark data set show that the developed ensemble methods have improved the recognition accuracy. The Bagging with Random Forest decision tree has reached 99% correct classification on FERET face database.
更多查看译文
关键词
Skin Color Detection,Face Recognition,Histogram,Bagging,Adaboost,Multiboosting
AI 理解论文
溯源树
样例
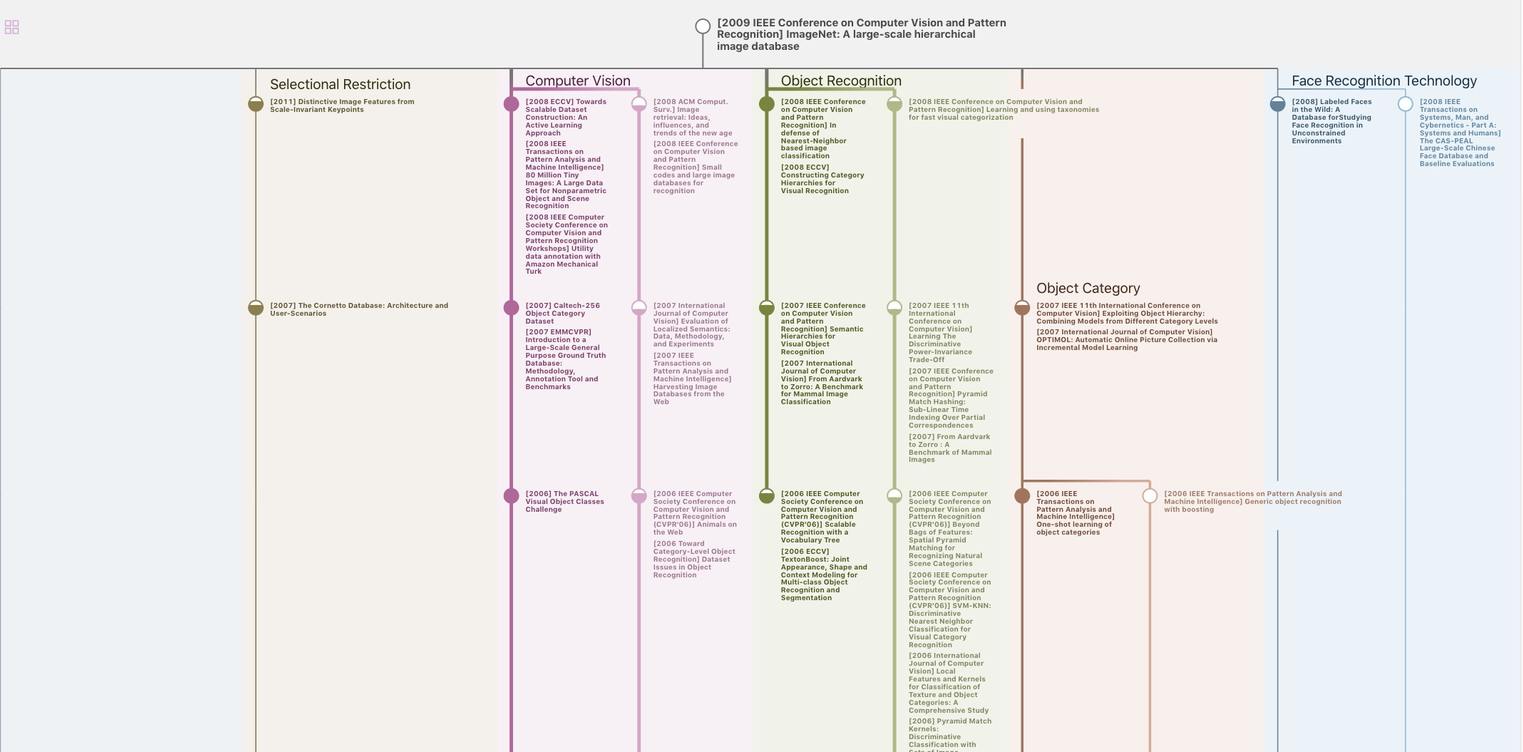
生成溯源树,研究论文发展脉络
Chat Paper
正在生成论文摘要