Handling Massive Proportion of Missing Labels in Multivariate Long-Term Time Series Forecasting
Journal of Physics: Conference Series(2021)
摘要
Abstract Training Deep Learning (DL) models with missing labels is a challenge in diverse engineering applications. Missing value imputation methods have been proposed to try to address this problem, but their performance is affected with Massive Proportion of Missing Labels (MPML). This paper presents a approach for handling MPML in Multivariate Long-Term Time Series Forecasting. It is an two-step process where interpolation (using Gaussian Processes Regression (GPR) and domain knowledge from experts) and prediction model are separated to enable the integration of prior domain knowledge. First, a set of samples of the possible interpolation of the missing outputs are generated by the GPR based on the domain knowledge. Second, the observed input sensor data and interpolated labels from GPR are used to train the prediction model. We evaluated our approach with the development of a soft-sensor with one real datasets to forecast the biomass during recombinant adeno-associated virus (rAAV) production in bioreactors. Our experimental results demonstrate the potential of the approach through quantitative evaluation of the generated forecasts in a case that would be extremely difficult to train a DL model due to MPML.
更多查看译文
AI 理解论文
溯源树
样例
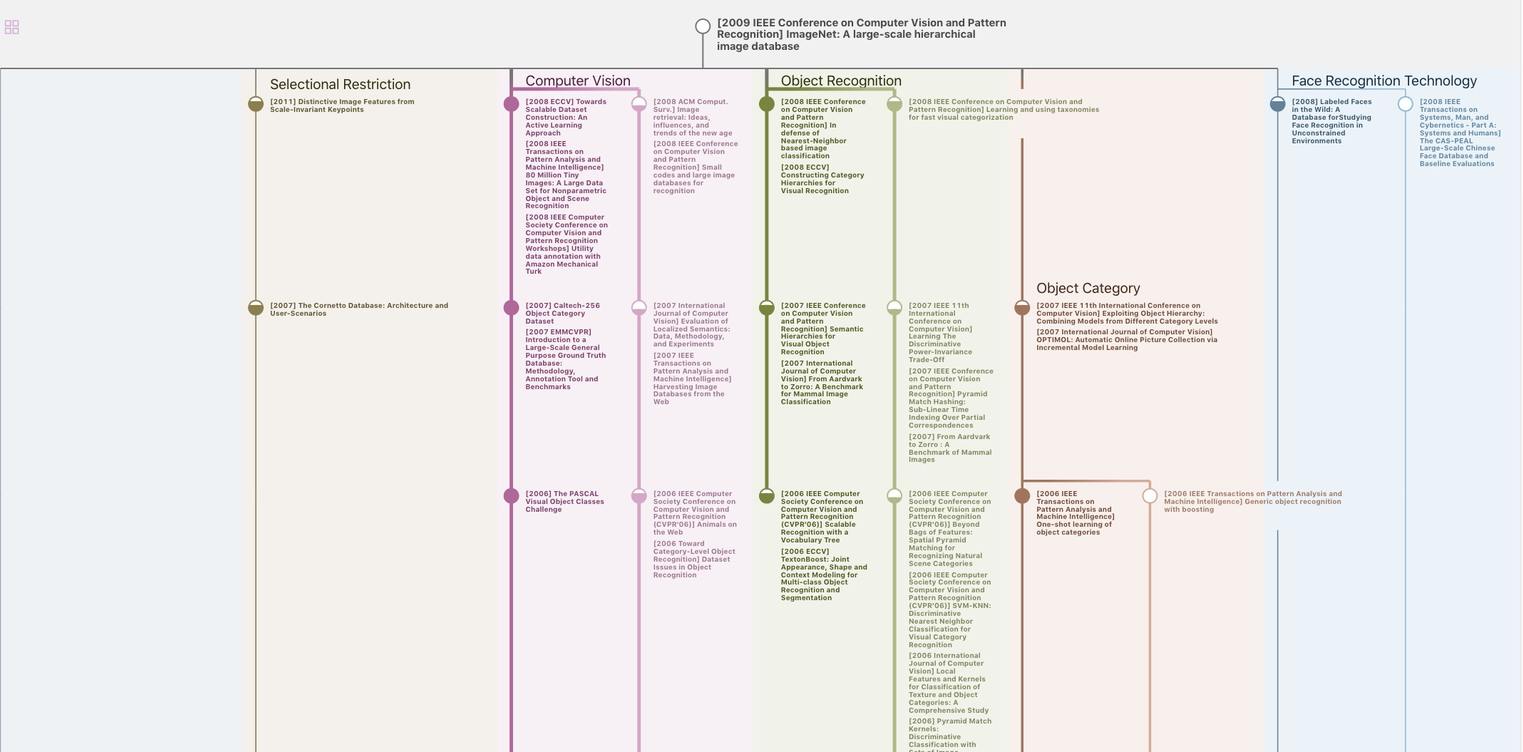
生成溯源树,研究论文发展脉络
Chat Paper
正在生成论文摘要