Coreference Resolution Through a Seq2seq Transition-Based System
TRANSACTIONS OF THE ASSOCIATION FOR COMPUTATIONAL LINGUISTICS(2023)
摘要
Most recent coreference resolution systems use search algorithms over possible spans to identify mentions and resolve coreference. We instead present a coreference resolution system that uses a text-to-text (seq2seq) paradigm to predict mentions and links jointly. We implement the coreference system as a transition system and use multilingual T5 as an underlying language model. We obtain state-of-the-art accuracy on the CoNLL-2012 datasets with 83.3 F1-score for English (a 2.3 higher F1-score than previous work [Dobrovolskii, 2021]) using only CoNLL data for training, 68.5 F1-score for Arabic (+4.1 higher than previous work), and 74.3 F1-score for Chinese (+5.3). In addition we use the SemEval-2010 data sets for experiments in the zero-shot setting, a few-shot setting, and supervised setting using all available training data. We obtain substantially higher zero-shot F1-scores for 3 out of 4 languages than previous approaches and significantly exceed previous supervised state-of-the-art results for all five tested languages. We provide the code and models as open source.1
更多查看译文
关键词
Sequence-to-Sequence Learning,Topic Modeling,Syntax-based Translation Models,Language Modeling,Semantic Similarity
AI 理解论文
溯源树
样例
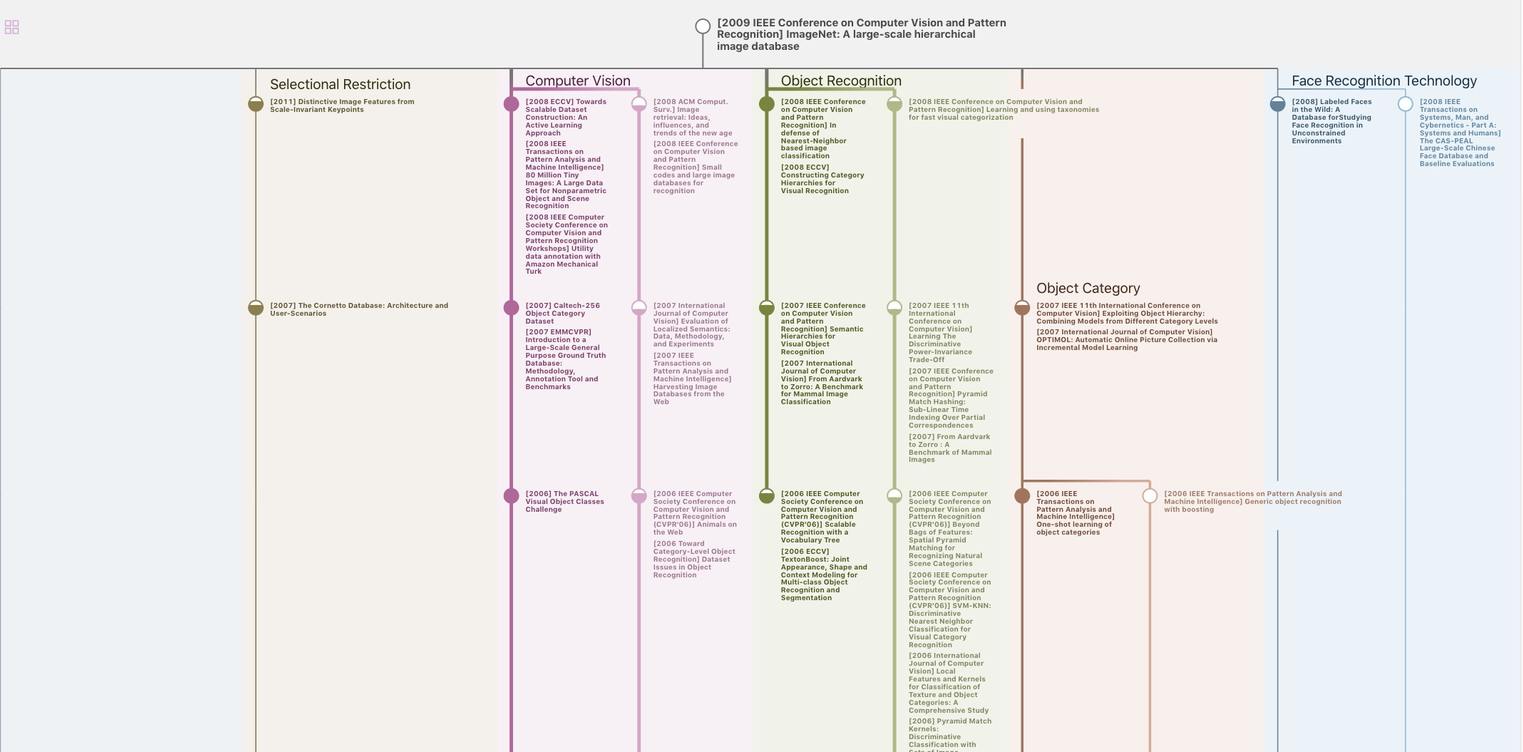
生成溯源树,研究论文发展脉络
Chat Paper
正在生成论文摘要