Document-Level Abstractive Summarization
CoRR(2022)
摘要
The task of automatic text summarization produces a concise and fluent text summary while preserving key information and overall meaning. Recent approaches to document-level summarization have seen significant improvements in recent years by using models based on the Transformer architecture. However, the quadratic memory and time complexities with respect to the sequence length make them very expensive to use, especially with long sequences, as required by document-level summarization. Our work addresses the problem of document-level summarization by studying how efficient Transformer techniques can be used to improve the automatic summarization of very long texts. In particular, we will use the arXiv dataset, consisting of several scientific papers and the corresponding abstracts, as baselines for this work. Then, we propose a novel retrieval-enhanced approach based on the architecture which reduces the cost of generating a summary of the entire document by processing smaller chunks. The results were below the baselines but suggest a more efficient memory a consumption and truthfulness.
更多查看译文
关键词
summarization,document-level
AI 理解论文
溯源树
样例
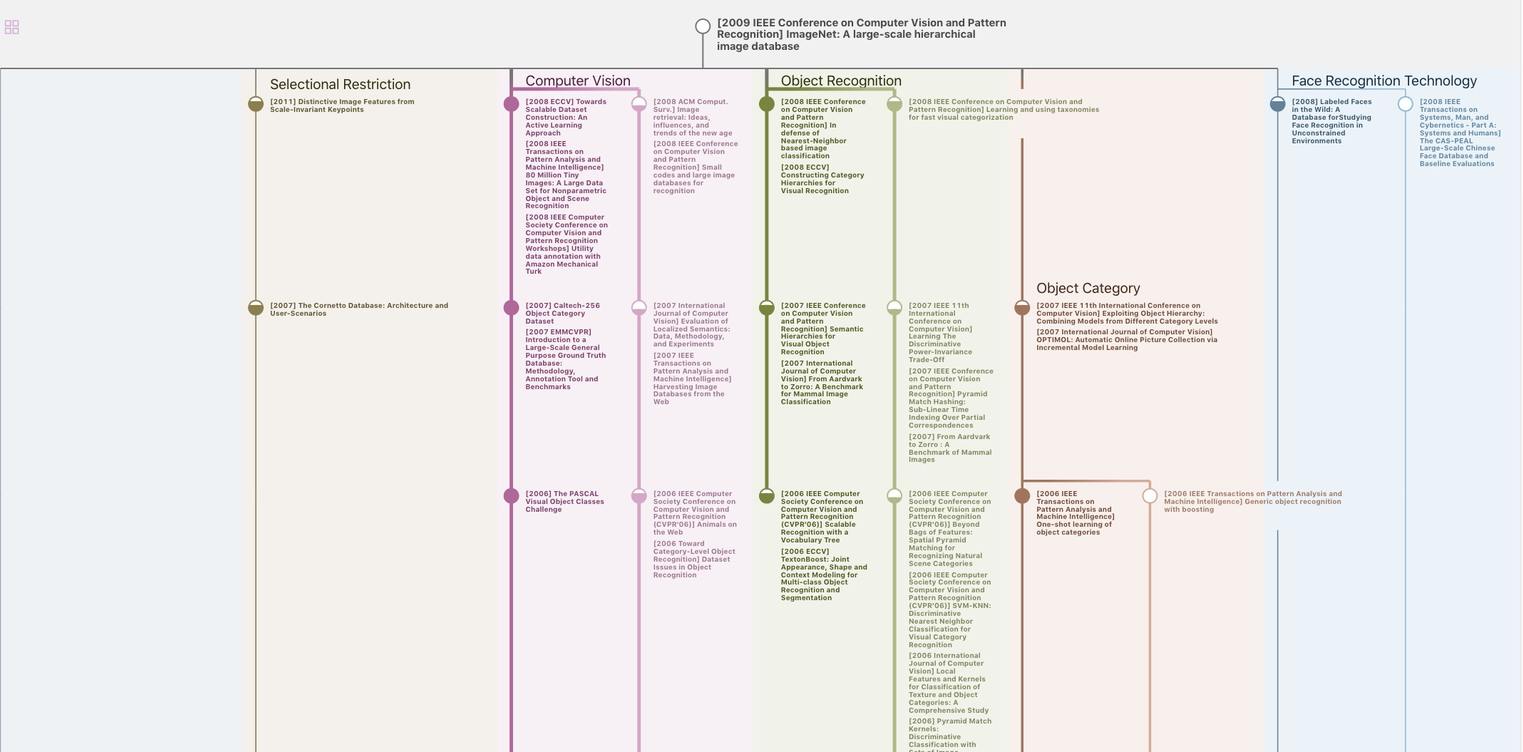
生成溯源树,研究论文发展脉络
Chat Paper
正在生成论文摘要