Machine-Learning-Based Opportunistic Spectrum Access in Cognitive Radio Networks
IEEE Wireless Communications(2020)
摘要
The explosive growth of wireless devices and data rate demands makes spectrum scarcity a serious problem. A promising solution is to employ OSA, which enables SUs to seek and opportunistically exploit the underutilized spectrum without interrupting the data transmission of PUs. However, the real-world implementation of OSA still faces several critical challenges including lack of global information, the dilemma of exploration and exploitation, and channel access competition. In this article, we propose a machine-learning-based OSA framework by integrating MAB and matching theory. First, we start from the single-SU scenario without global information while considering the volatility of channel availability. We propose an occurrence-aware OSA (OA-OSA) framework based on the UCB algorithm, which can achieve long-term optimal network throughput performance and a well-balanced trade-off between exploration and exploitation based on only local information. Then we extend OA-OSA to the multi- SU scenario with channel access competitions, and derive an OCA-OSA framework by integrating OA-OSA and the Gale-Shapley algorithm. Simulation results demonstrate that the proposed frameworks achieve superior performance in network throughput and less deviation from optimal performance with global information.
更多查看译文
关键词
Throughput,Sensors,Estimation,Decision making,Uncertainty,Cognitive radio
AI 理解论文
溯源树
样例
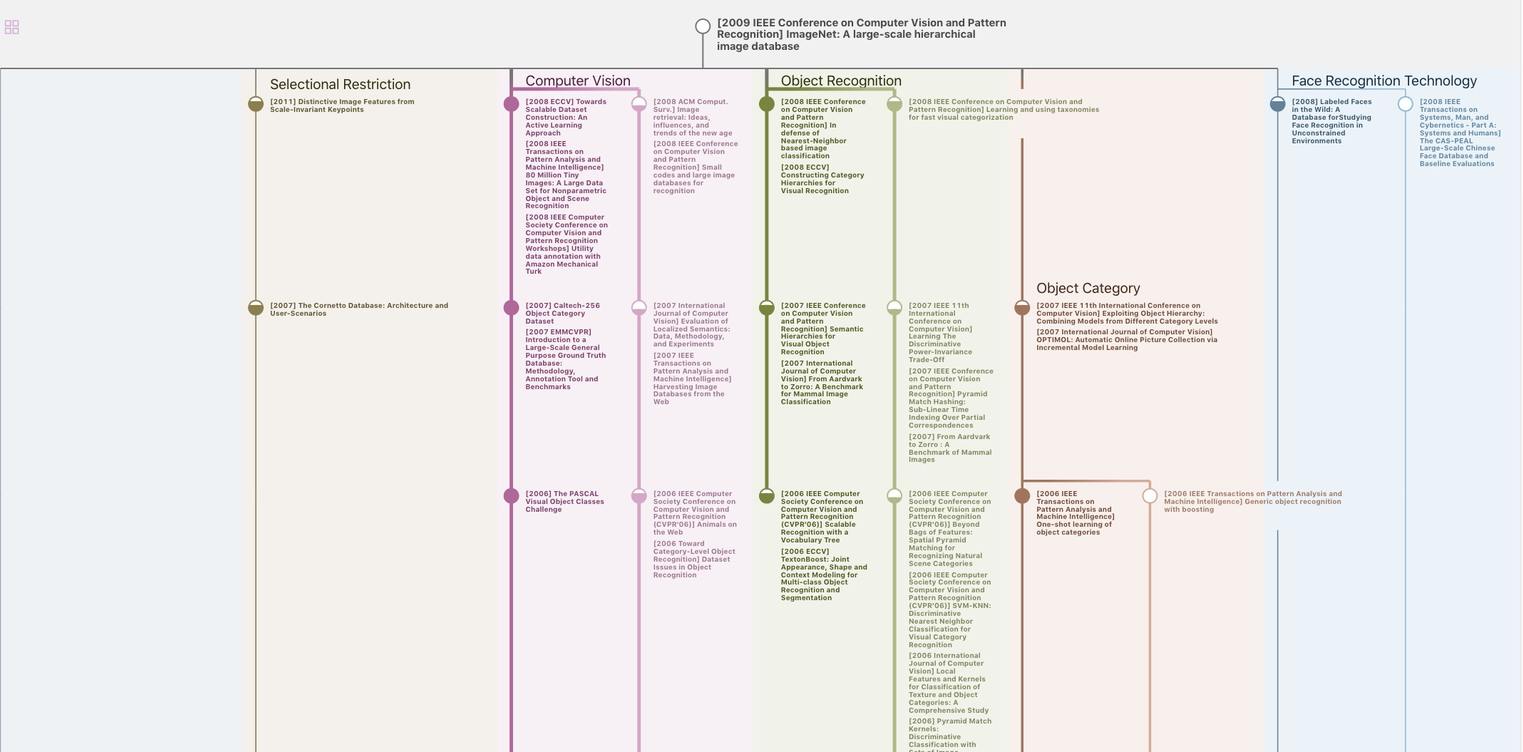
生成溯源树,研究论文发展脉络
Chat Paper
正在生成论文摘要