Data-Driven Online Recommender Systems With Costly Information Acquisition
IEEE Transactions on Services Computing(2023)
摘要
In numerous recommender systems, collecting useful information from users is costly, implying that the recommender system has to make active choices by simultaneously learning the observations of the features' states to make useful recommendations to users among available products and services. This article integrates information acquisition decisions into recommender system. To solve the aforementioned dual learning problem, we propose two different algorithms, namely Sim-OOS and Seq-OOS, where observations are made simultaneously and sequentially, respectively. We prove that both algorithms guarantee a sublinear regret. The developed recommender system can be applied to a variety of real-world applications, including medical informatics, smart transportation, finance, and cyber-security where collecting information before making decisions results in an excessive cost. We validate and evaluate our proposed policies in a medical decision-support system that recommends tests and treatments for breast cancer patients.
更多查看译文
关键词
Recommender system,decision-making,online learning,contextual multi-armed bandits,costly information acquisition
AI 理解论文
溯源树
样例
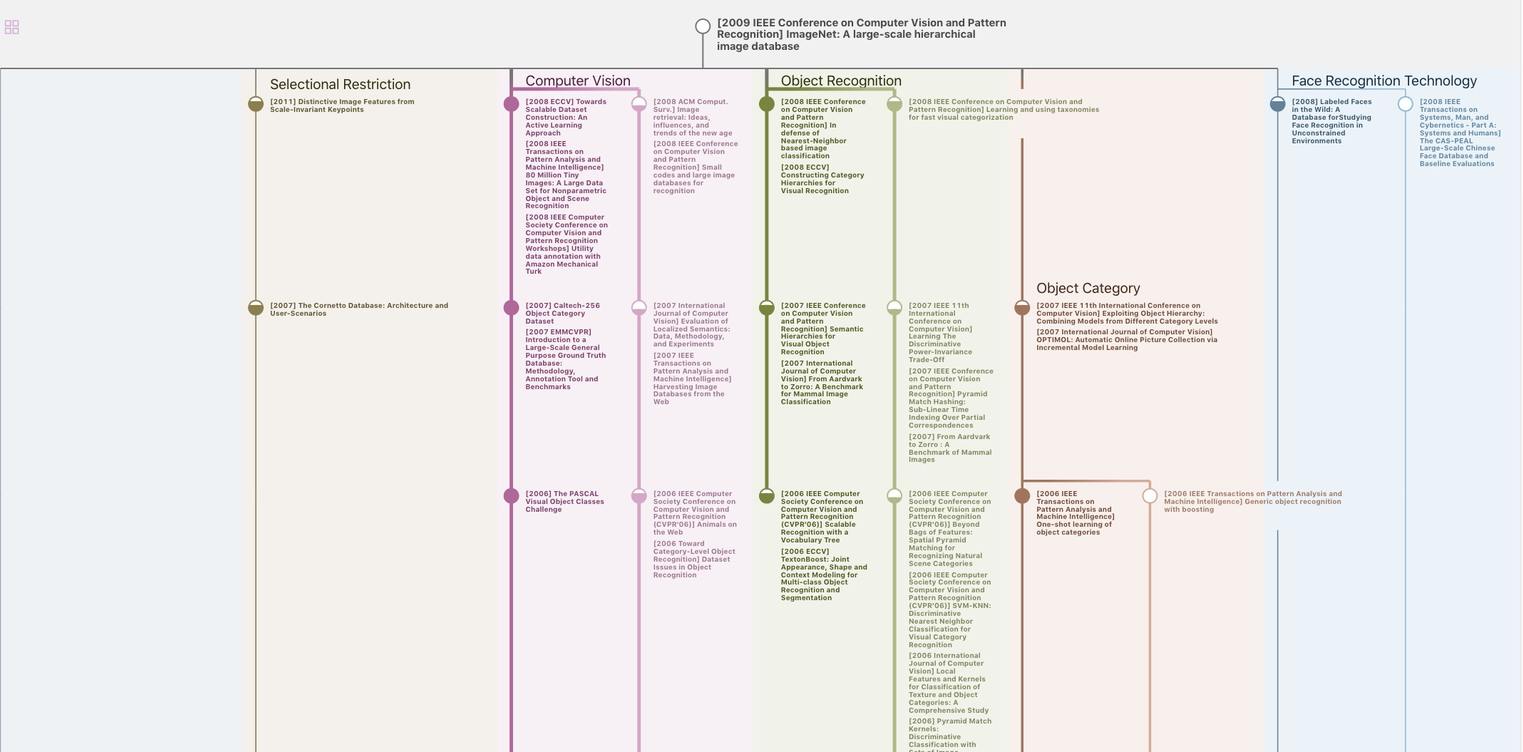
生成溯源树,研究论文发展脉络
Chat Paper
正在生成论文摘要