Amodal Statistical Completion of Occluded Objects
Journal of vision(2021)
摘要
We can easily recognize objects even when they are occluded. Does our perception complete the occluded edges linearly or can it also employ more sophisticated extrapolation? To investigate this, we created displays where each occluded shape had two possible completions. These had identical contours in the unoccluded portion but differed in the occluded portion, such that one appeared to be the more likely shape behind the occluder, and the other was unlikely. Across two sets of such displays, we manipulated either the curvature of the occluded edge (‘curvature’ set) or the numerosity of edges (‘numerosity’ set). We asked whether perceptual dissimilarities, measured using visual search, between pairs of occluded shapes were correlated with those measured between pairs of likely or unlikely shapes. We observed that occluded shape dissimilarities were more correlated with the likely shape dissimilarities (r = 0.85 for ‘curvature’ and r = 0.88 for ‘numerosity’ sets, p < 0.0005) compared to unlikely shape dissimilarities (r = 0.78 for ‘curvature’ and r = 0.67 for ‘numerosity’ sets, p < 0.0005). Next, we asked whether similar patterns are present in deep neural networks trained for object recognition. In deep networks, the occluded shape representation matched better with the likely shape compared to the unlikely shape for the curvature set (r = 0.92 for likely shapes and r = 0.81 for unlikely shapes, p < 0.0005) but not for the numerosity set (r = 0.77 for likely shapes and r = 0.77 for unlikely shapes, p < 0.0005). Thus, deep neural networks failed to correctly extrapolate the statistics of the visible portion. Taken together, our results show that amodal completion is more complex than simple extrapolation of edges, relying on statistical properties to complete occluded objects.
更多查看译文
AI 理解论文
溯源树
样例
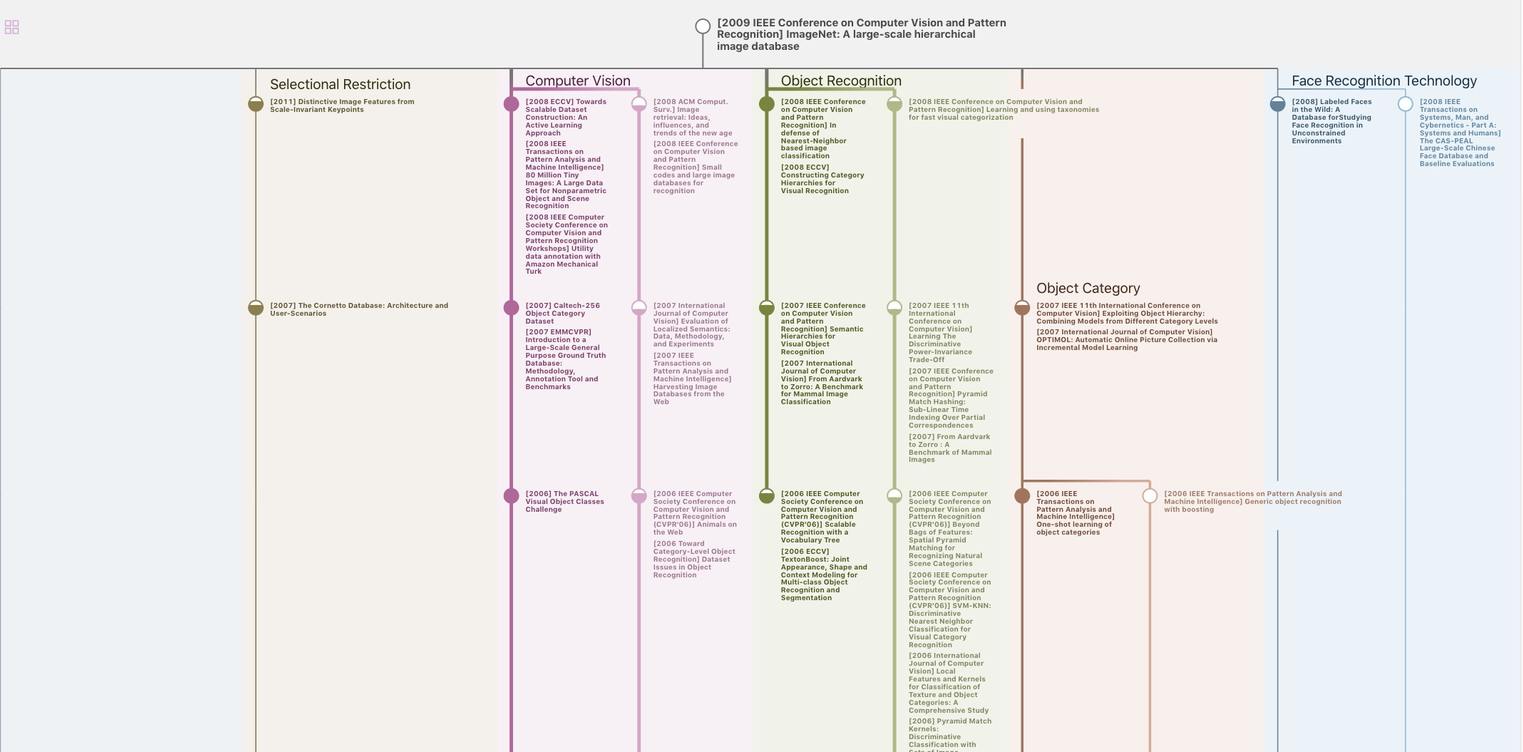
生成溯源树,研究论文发展脉络
Chat Paper
正在生成论文摘要