Why segmentation matters: a Machine Learning approach for predicting loan defaults in the Peer-to-Peer (P2P) Financial Ecosystem
Risk Management Magazine(2021)
摘要
Peer-to-Peer (P2P) lending is an online lending process allowing individuals to obtain or concede loans without the interference of traditional financial intermediaries. It has grown quickly the last years, with some platforms reaching billions of dollars of loans in principal in a short amount of time. Since each loan is associated with the probability of loss due to a borrower's failure, this paper addresses the borrower's default prediction problem in the P2P financial ecosystem. The main assumption, which makes this study different from the available literature, is that borrowers sharing the same homeownership status display similar risk profile, thus a model per segment should be developed. We estimate the Probability of Default (PD) of a borrower by using Logistic Regression (LR) coupled with Weight of Evidence encoding. The features set is identified via the Sequential Feature Selection (SFS). We compare the forward against the backward SFS, in terms of the Area Under the Curve (AUC), and we choose the one that maximizes this statistic. Finally, we compare the results of the chosen LR approach against two other popular Machine Learning (ML) techniques: the k Nearest Neighbors (k-NN) and the Random Forest (RF).
更多查看译文
关键词
p2p lending,risk prediction,machine learning,loan defaults.
AI 理解论文
溯源树
样例
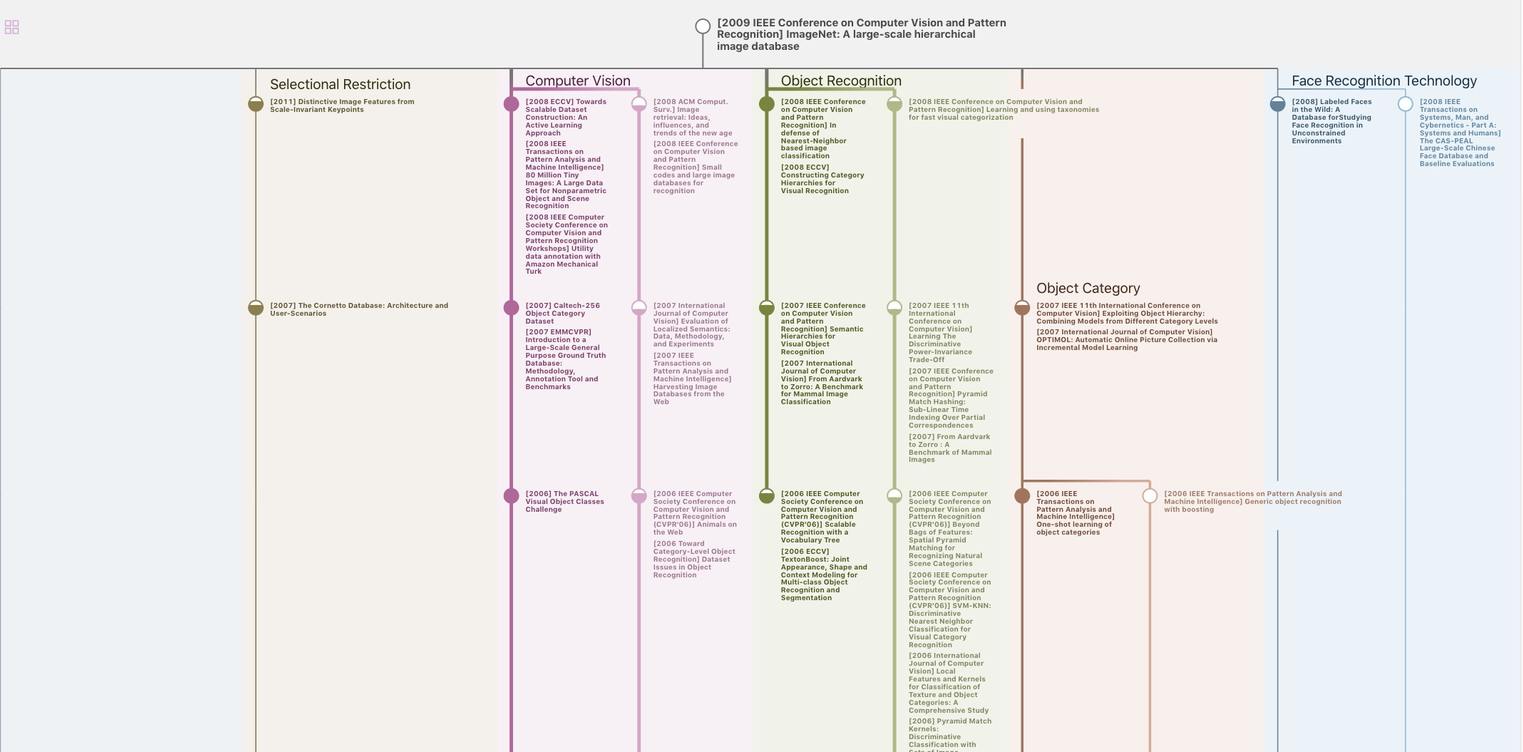
生成溯源树,研究论文发展脉络
Chat Paper
正在生成论文摘要