Time Series Forecasting in Retail Sales Using LSTM and Prophet
Advances in Business Information Systems and AnalyticsHandbook of Research on Applied Data Science and Artificial Intelligence in Business and Industry(2021)
摘要
Data science highlights fields of study and research such as time series, which, although widely explored in the past, gain new perspectives in the context of this discipline. This chapter presents two approaches to time series forecasting, long short-term memory (LSTM), a special kind of recurrent neural network (RNN), and Prophet, an open-source library developed by Facebook for time series forecasting. With a focus on developing forecasting processes by data mining or machine learning experts, LSTM uses gating mechanisms to deal with long-term dependencies, reducing the short-term memory effect inherent to the traditional RNN. On the other hand, Prophet encapsulates statistical and computational complexity to allow broad use of time series forecasting, prioritizing the expert's business knowledge through exploration and experimentation. Both approaches were applied to a retail time series. This case study comprises daily and half-hourly forecasts, and the performance of both methods was measured using the standard metrics.
更多查看译文
关键词
time series forecasting,retail sales,lstm,time series,prophet
AI 理解论文
溯源树
样例
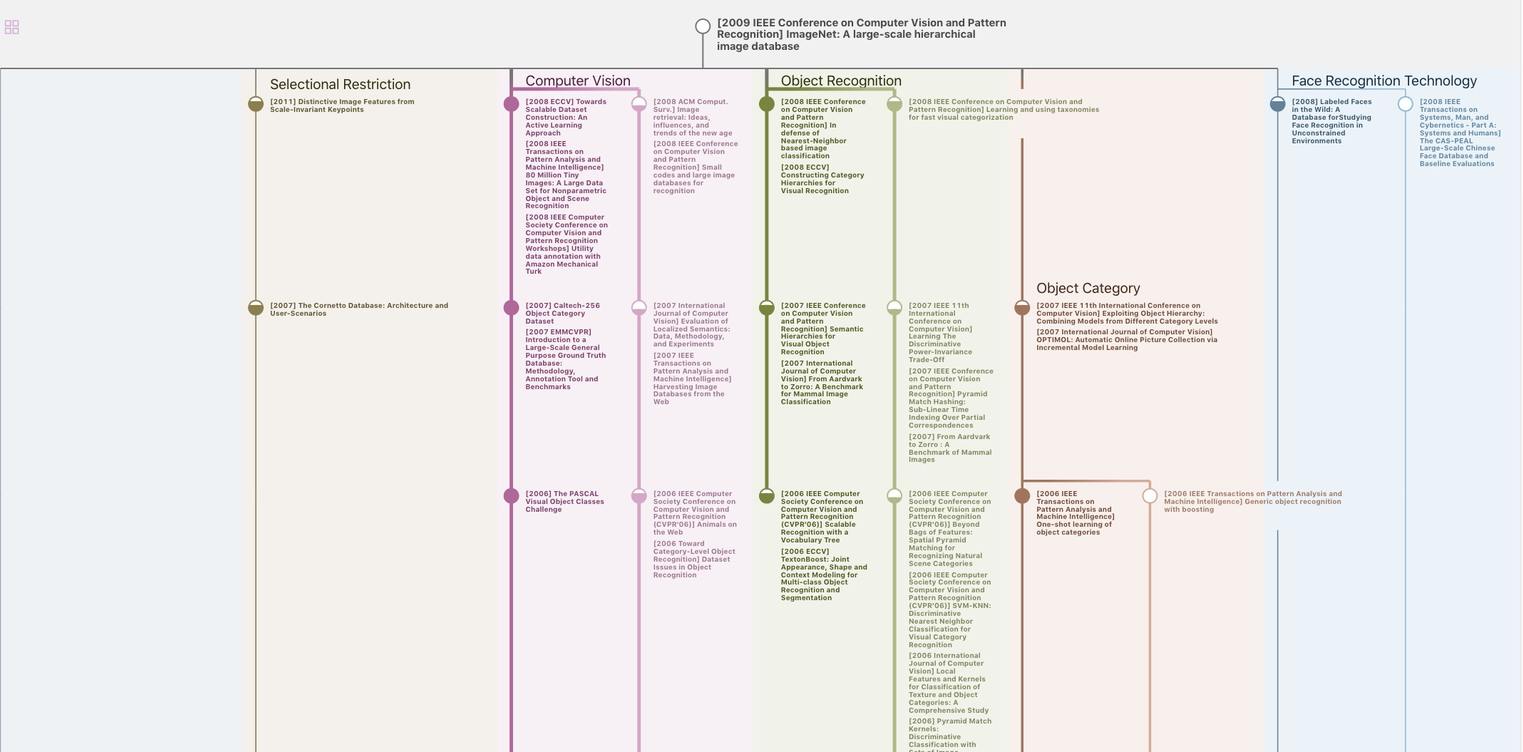
生成溯源树,研究论文发展脉络
Chat Paper
正在生成论文摘要