Digging in Documents: Using Text Mining to Reveal Hidden Knowledge in the Lithium-Ion Battery Literature
ECS Meeting Abstracts(2021)
摘要
Scientific publications constitute the main information source of battery-related data for academic research. There is an impressive number of papers published on Lithium Ion Batteries (LIBs) for instance. Such impressive amount of data in principle could be used by machine learning (ML) methods in order to optimize efforts and even make possible the discovery of new optimal experimental conditions. Yet, the majority is published as unstructured data, making ML analysis difficult. Here, in order to unravel researchers’ habits when reporting their results, we present a text mining study using primarily publications containing original experimental data from the LIB field. We analyze the reported data structure and we discuss the remaining challenges for applying automated knowledge discovery techniques. We then present a Named Entity Recognition approach to automatically extract quantified parameters from the battery literature and how to build an in house generated database containing a wide diversity of materials, experimental parameters and conditions. Our in house approach achieves a data extraction F-scores ≥ 90% and can thereby pave the way towards an automatized way of generating large databases for use in battery ML models. References El-Bouysidy, H., Lombardo, T., Primo, E. N., Duquesnoy, M., Morcrette, M., Johansson, P., Simon, P., Grimaud, A., & Franco, A. A. submitted (2020). Torayev, Amangeldi, Pieter CMM Magusin, Clare P. Grey, Céline Merlet, and Alejandro A. Franco. Journal of Physics: Materials 2, no. 4 (2019): 044004.
更多查看译文
关键词
text mining,hidden knowledge,battery,lithium-ion
AI 理解论文
溯源树
样例
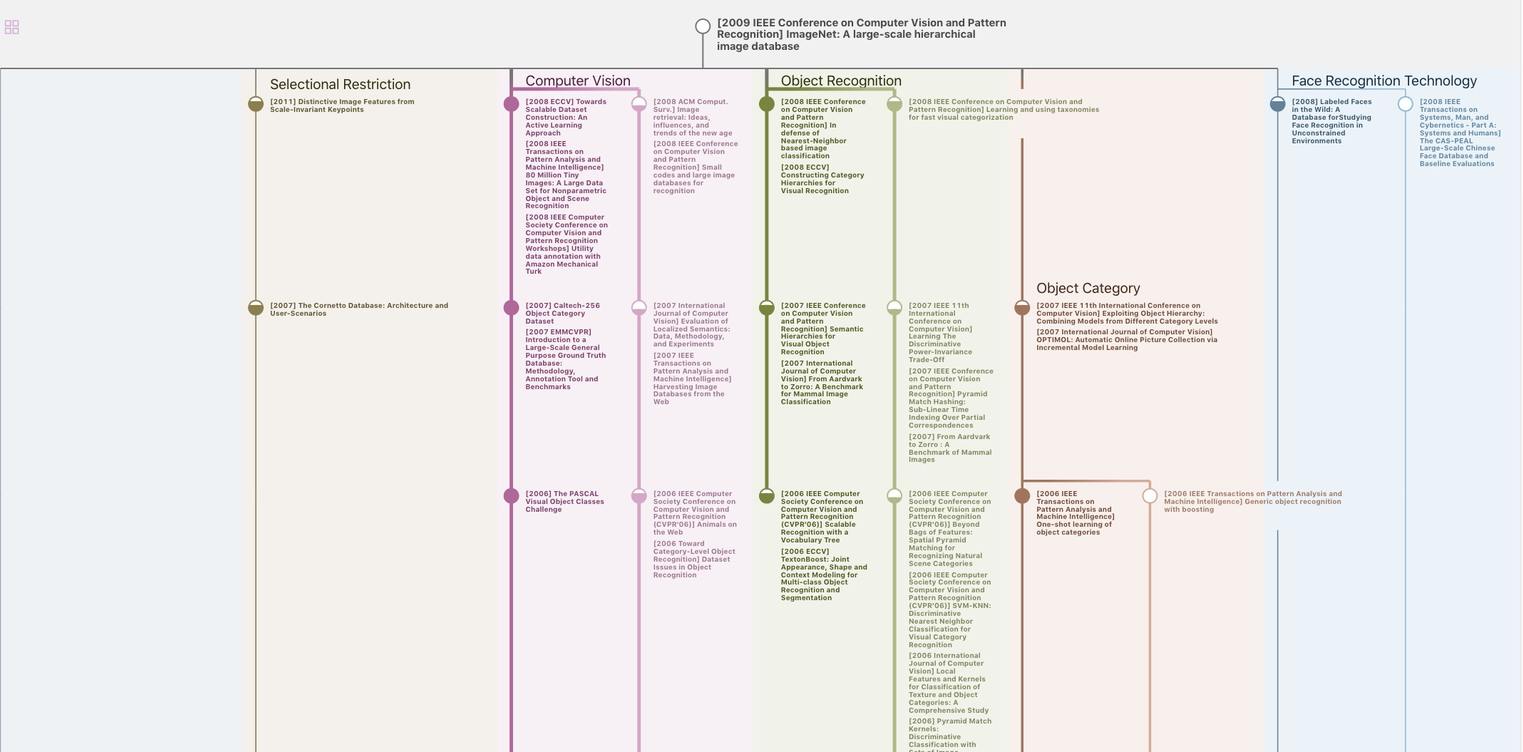
生成溯源树,研究论文发展脉络
Chat Paper
正在生成论文摘要