Large-scale, multi-vendor, multi-protocol, quality-controlled analysis of clinical cine CMR using artificial intelligence
European Heart Journal - Cardiovascular Imaging(2021)
摘要
Abstract Funding Acknowledgements Type of funding sources: Public grant(s) – National budget only. Main funding source(s): Advancing Impact Award scheme of the EPSRC Impact Acceleration Account at King’s College London Background Artificial intelligence (AI) has the potential to facilitate the automation of CMR analysis for biomarker extraction. However, most AI algorithms are trained on a specific input domain (e.g., scanner vendor or hospital-tailored imaging protocol) and lack the robustness to perform optimally when applied to CMR data from other input domains. Purpose To develop and validate a robust CMR analysis tool for automatic segmentation and cardiac function analysis which achieves state-of-the-art performance for multi-vendor short-axis cine CMR images. Methods The current work is an extension of our previously published quality-controlled AI-based tool for cine CMR analysis [1]. We deployed an AI algorithm that is equipped to handle different image sizes and domains automatically - the ‘nnU-Net’ framework [2] - and retrained our tool using the UK Biobank (UKBB) cohort population (n = 4,872) and a large database of clinical CMR studies obtained from two NHS hospitals (n = 3,406). The NHS hospital data came from three different scanner types: Siemens Aera 1.5T (n = 1,419), Philips Achieva 1.5T and 3T (n = 1,160), and Philips Ingenia 1.5T (n = 827). The ‘nnU-net’ was used to segment both ventricles and the myocardium. The proposed method was evaluated on randomly selected test sets from UKBB (n = 488) and NHS (n = 331) and on two external publicly available databases of clinical CMRs acquired on Philips, Siemens, General Electric (GE), and Canon CMR scanners – ACDC (n = 100) [3] and M&Ms (n = 321) [4]. We calculated the Dice scores - which measure the overlap between manual and automatic segmentations - and compared manual vs AI-based measures of biventricular volumes and function. Results Table 1 shows that the Dice scores for the NHS, ACDC, and M&Ms scans are similar to those obtained in the highly controlled, single vendor and single field strength UKBB scans. Although our AI-based tool was only trained on CMR scans from two vendors (Philips and Siemens), it performs similarly in unseen vendors (GE and Canon). Furthermore, it achieves state-of-the-art performance in online segmentation challenges, without being specifically trained on these databases. Table 1 also shows good agreement between manual and automated clinical measures of ejection fraction and ventricular volume and mass. Conclusions We show that our proposed AI-based tool, which combines training on a large-scale multi-domain CMR database with a state-of-the-art AI algorithm, allows us to robustly deal with routine clinical data from multiple centres, vendors, and field strengths. This is a fundamental step for the clinical translation of AI algorithms. Moreover, our method yields a range of additional metrics of cardiac function (filling and ejection rates, regional wall motion, and strain) at no extra computational cost.
更多查看译文
AI 理解论文
溯源树
样例
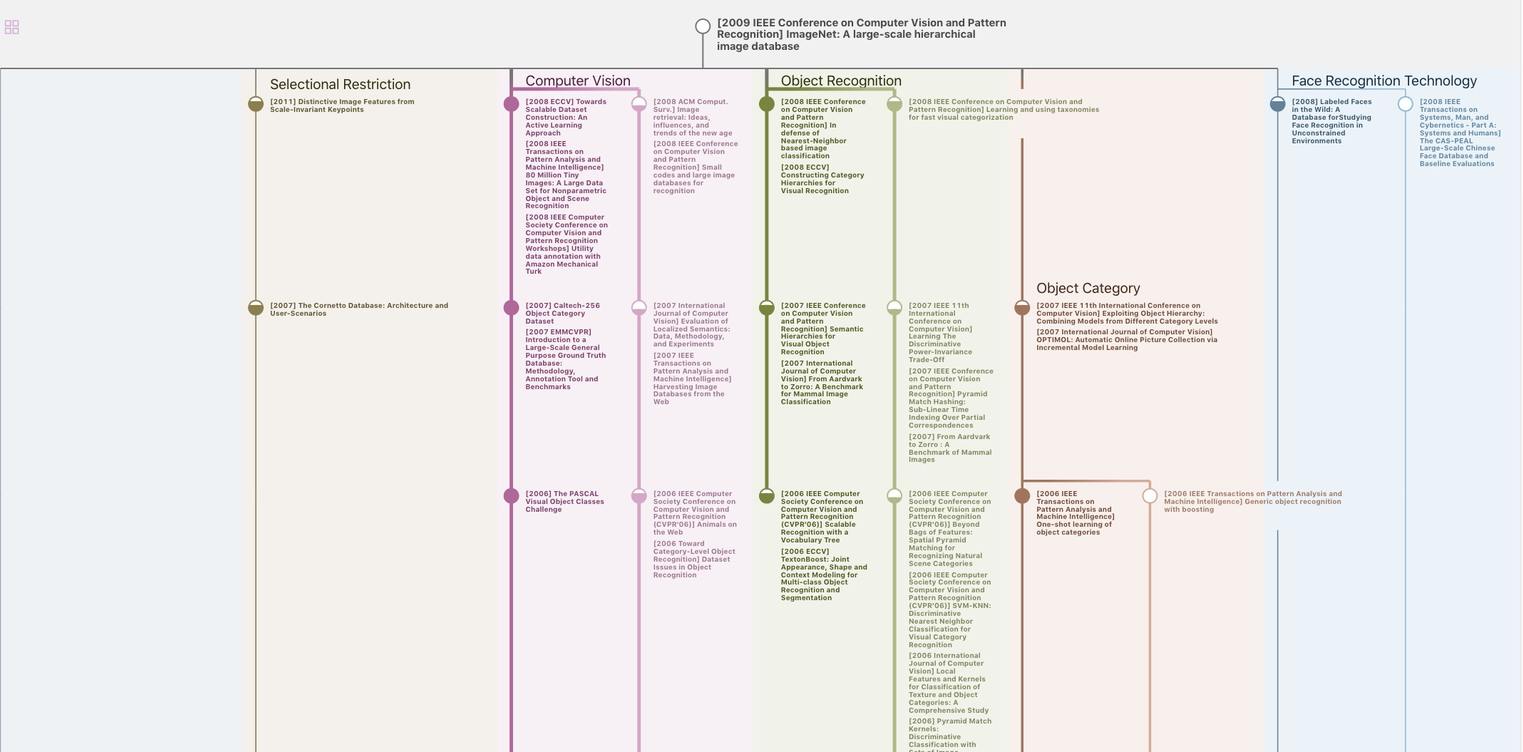
生成溯源树,研究论文发展脉络
Chat Paper
正在生成论文摘要